ABSTRACT
In this paper, the panel data of China from 2003 to 2012 and stochastic frontier and threshold regression models were used to analyze the impact of government’s fund and enterprises’ research and development (R&D) investment on China’s innovation efficiency and the optimal intensity of different R&D investment and their interval analysis. This research indicates that in the process of innovation in China, the impact of government R&D funding on innovation efficiency is negative, called "government failure"; enterprises’ R&D investment can promote innovation efficiency, meaning "market failure" phenomenon will be less. The optimal interval of investment intensity is 0.288 or above and the optimal interval of R&D investing intensity coefficient is between 0.688 and 0.775.
Key words: Government R&D fund, enterprise R&D investment, innovation efficiency.
With the rise of Chinese economy and increasing cash accumulation of enterprises, in addition to meeting daily operation, enterprises will have more money to invest in research and development; while the current domestic and international market competition is fiercely increasing, in order to increase product competitiveness, enterprises need not only to improve the domestic and oversea market participation, but also need to increase investment in research and development, and thus enhance the overall innovation efficiency (Innovation efficiency refers to the input-output ratio of innovation behavior). Therefore, enterprise’s research and development (R&D) investment refers to R&D activities conducted by the enterprises themselves or through self-raised funds. Government R&D investment refers to R&D activities supported by financial funds. In recent years, the scale of government R&D funds has been greatly expanded in China and has always played a basic and important role in promoting innovation efficiency. In particular, since the reform and opening up policy, the government has set up a number of national research programs to support scientific research and development, mainly as follows: National Spark Program, National Natural Science Foundation, "863" plan, Torch Plan, and "973" plan. These government’s research programs promote the development of China's scientific research to some extent and provide an important guarantee for innovation efficiency. However, compared with the developed countries, China's innovation efficiency is still at a low level, which is still an indisputable fact. Therefore, assuring efficiency optimization of government R&D funding and enterprise R&D investment has become the key factor to the impact of innovation efficiency.
A country's R&D funding sources are mainly self-financing or government funding. The two kinds of models as part of innovation system play an important role in promoting economy growth. Enterprises’ R&D investment follows market-oriented principle; it applies new technologies, uses new products and then seeks competitive advantage for the purpose. So enterprises’ R&D investment may be more efficient than government’s R&D investment (Wang, 2011). However, due to the existence of "market failure", the monopoly of R&D resources, externalities and information asymmetry will interfere with enterprises’ R&D investment, which gives theoretical explanation for government funding enterprise’s research and development (Guellec and Van, 2003). At the same time, the government's investment on enterprise’s R&D will also be affected by imperfect information and market imperfection; there may be "government failure" problem, namely government's market response signals is numb and slow, inefficient and rent-seeking corruption problems (Jian and Qi, 2007). So what are the roles of a country's innovation efficiency? Are there any differences? How do we coordinate R&D fund structure and make a country's innovation efficiency to come to the optimal? These issues are our major concern. Based on the earlier considerations, this paper focuses on the following two aspects: first, utilizing transcendental logarithmic random frontier model to study the overall impact of government’s funding and enterprises’ R&D investment on the efficiency of innovation; second, with the help of threshold regression model to seek the optimization zone of government’s funding and enterprises’ R&D investment to effectively avoid "market failure" and "government failure". The contributions of this paper include: (1) establish dual failure framework of government and market at first, then analyze a country's innovative efficiency; (2) provide beneficial experiences on how to adjust the relationship between government and market for the promotion of innovation efficiency.
Government’s R&D fund and enterprises’ R&D investment as two kinds of important sources of R&D funding have raised and promoted innovation efficiency. However, due to the co-existence of "government failure" and "market failure", both of them do not only show the difference between the innovative output effects, but also show a "complementary relationship" and "alternative relationship" coexistence phenomenon.
Analysis on the different influence of government’s R&D funding and enterprises’ R&D investment on innovation efficiency
In the process of innovation in a country, government’s funding is an important guarantee for R&D investment and enterprises’ investment as an important source of R&D funds; both kinds of funds have impact on the improvement of innovation efficiency. However, due to the differences between the two kinds of bodies, government’s R&D funding and enterprises’ R&D investment have different influence. This paper mainly summarizes three aspects: the degree of participation, the impact field and the impact style. As the main body of technology innovation, enterprises’ R&D investment plays a leading role in the innovation process of a country. While government’s funding plays a more important role in standardization and management. As the competition between enterprises increases, in order to obtain higher profits and seek competitive advantage and based on market demand-oriented, enterprises mainly obtain the application of new technologies and new products and introduce foreign technology at the same time, enlarge R&D self-investment, improve their own R&D technology and product technology. Therefore, enterprises concern more on the utilization of investment funds; the utilization efficiency of enterprises’ R&D funds may be higher than the government’s R&D funding (Miao, 2011). Government’s funding is mainly concentrated on the field of basic research and enterprises’ R&D investment mainly focuses on application research. These have different effects and influences in different innovation areas. In the short term, application research often targets R&D and technology acquisition, as business mainstay; in order to obtain commercial technology and new products and seek competitive advantage, enterprises’ R&D investment frequently focuses on more efficient innovative projects that are market-oriented; R&D results output is faster (Yan and Gong, 2013).
However, basic research has much more and relatively large impact on the radiation and guidance of other research areas in long term, often brings greater economic and social value, and improves the overall innovation level; government’s R&D funding is much more efficient on overall innovation efficiency. Government’s R&D funding based on original R&D resources and enterprises’ R&D investment can bring breakthrough on R&D funds’ structure. Government’s R&D funding mainly provide funds directly, with relatively large funds support to ease the pressure of enterprises’ investment shortage. When the government exists within the rent-seeking corruption, the existence of internal effects, making the “government failure” phenomenon continues to affect the usage efficiency of government’s funds (Yue et al., 2002). Enterprise can bring knowledge overflow through market information feedback, bring new technology and knowledge "learning by doing" such as staff exchange and demo imitation, guide enterprises to allocate innovative resources much more effectively and promote innovation efficiency (Fang et al., 2011). The incomplete and asymmetry information caused by the "market failure" phenomenon misleads their own R&D investment, affects the rational allocation of R&D resources and improves innovation efficiency (Xing, 2004).
Analysis on the optimal intensity interval between Government’s R&D funding and enterprises’ R&D investment
Government’s R&D funding and enterprises’ R&D investment complement with each other in the whole innovation process. The intensity of government R&D funding and the intensity of enterprise’s R&D investment indicate in this paper the weighting of government and enterprises’ investment in innovation and R&D activities. However, limited nature of government’s R&D funding and enterprises’ R&D investment will result in government’s R&D funding and enterprises’ R&D investment push out with each other and form "alternative relations"; this has negative impact on the overall innovation efficiency. The "complementary relationship" between government’s R&D funding and enterprises’ R&D investment can improve the usage efficiency of government’s R&D funding. First of all, government’s R&D funding has an effect on enterprises’ R&D investment. Spence (1984) studied European experiences and found that government’s R&D funding directly reduces the cost of enterprise technology innovation, reduces the risk of enterprise’s R&D investment; thereby could enhance the motivation and enthusiasm of enterprises’ devotion to research and development. Czarnitzki and Licht (2006) have confirmed the positive incentive of government’s R&D funding on enterprises’ R&D investment through empirical research from German enterprises, and R&D funding has also increased the likelihood of enterprises acquiring patents. Li and Wang (2010) pointed out that crowding-out effect between Chinese government R&D funding and enterprises’ R&D investment does not exist, but effectively stimulated the motivation and enthusiasm of enterprises’ devotion to research and development.
Secondly, enterprises’ R&D investment has an "incentive effect" on government’s R&D funding. Enterprises’ R&D investment does not only improve their own research and development capabilities and ensure the realization of expected purpose of government’s funds support; but also fully demonstrate the consistency between enterprises and national interests, and further attract government’s R&D funding. Liu (2000) and Wu (2006) indicate that: by strengthening R&D investment, enterprises improve their own technology innovation and product innovation, and improve their competitiveness and profits. At the same time, that improves the level and progress of whole society’s technology. In this beneficial process of self-realization, the role of enterprises in national innovation system has become increasingly prominent and government will further increase government’s R&D funds to promote the role of enterprises in national innovation system (Perkmann and Walsh, 2009). The "alternative relationship" between governments’ funding and enterprises’ investment in research and development is mainly embodied in mutual "crowding-out effect". Firstly, government’s R&D funding will squeeze out enterprises’ R&D investment. If enterprises are relatively easy to obtain government’s R&D funding, then they will be in loss of competitiveness and enthusiasm on R&D activities. Klette et al. (2000) and Schreyer (2000) found that government is generally inclined to support the projects with high success probability and high returns, which are exactly what enterprise itself is ready to implement. If the subsidized enterprise directly utilizes government grant funds to replace its own R&D investment or adjust to carry out the new subsidized R&D projects, to give up the unsupported R&D projects, government’s funding for enterprise will produce "crowding out effect" (Cheng and Zhao, 2009).
In addition, the increasing of government’s funding also increases enterprises’ demand for scarce R&D fund resources. If the determinants of R&D (such as high-level labor) are insufficiently supplied, market mechanism will increase the salary level of R&D personnel and then reduce the enthusiasm of enterprises in employing R&D personnel, and change their R&D investment behavior. Goolsbee (1998) confirmed that when R&D expense and cost increase, enterprises will abandon some R&D projects and turn to other profitable projects, lead to squeeze some enterprise’s R&D investments. This "squeeze effect" in the country with scarce scientific and technological resources becomes especially serious. Secondly, enterprises’ R&D investment will reduce government’s R&D funding. Enterprise, as main-bodies in market, could be aware of enormous and various market information. At present, R&D investment from Chinese enterprises has achieved good results, which have significantly improved R&D innovation efficiency, and led enterprises to expand their R&D investment scale (Zhu et al., 2014). With the continuing improvement of enterprises’ R&D, the scale of enterprise R&D investment is expanding, will be part of government R&D funds, that makes the scale of government funds gradually to be narrowed, the ration of China’s enterprises’ R&D investment accounted in the total social R&D investment from less than 60% in 2000 to about 75% in 2010 (Kang, 2013).
EMPIRICAL MODEL SETTING AND VARIABLE DEFINITION
Empirical model setting
Basic model settings
In choice of production function, most commonly used two kinds of function are Cobo-Douglas and beyond logarithmic forms. Although the former is with simple form, but its assumption of technology is neutral and output elasticity is fixed (Pai et al., 2009). The latter relaxes these hypotheses, which are more flexible in form and can better avoid estimate deviation due to misuse of functions (Fu and Wu, 2007). In order to examine the effect of government’s R&D funding and enterprises’ R&D investment on China innovation efficiency, according to Battese and Coelli (1995) model setting, this paper utilizes stochastic frontier model of beyond logarithmic production function as regression model, as follows:
lnPatentit=β0+β1+lnKit+1/2β2(lnKit)2+1/2β3+(lnLit)2+β4lnKitlnLit+vit+uit (1)
uit=δ0+δ1+lnGRDit+δ2lnBRDit+δ3lnTMTit+δ4lnLaborit+δ5lnInfit+δ6lnOpenit+wit (2)
In Equation 1, βi (i=0,1…5) is the coefficient of regression variable, Patentit, Kit, and Lit, respectively denote patent output, R&D capital stock and R&D personnel input at i-region in t-year, νit denotes random disturbance items, and uit denotes technical inefficiency. In Equation 2, ri(i=0,1…5) is the coefficient of the regression variable, GRDit, BRDit, TMTit, Laborit, Infit and Openit, respectively represent the level of government’s R&D funding, enterprises’ R&D investment, technology innovation environment, human capital level, region infrastructure level and opening up level, and ωi denotes random error term.
Threshold model setting
Based on the threshold regression model developed by Hansen (1999), this paper selects government’s R&D funding intensity ωitG and enterprises’ R&D investment intensity ωitB as threshold variable; tG, tB is the threshold value of these two threshold variables, take dual-threshold as example to build a regression model:
Uit=δ0+ηlnXit+κ1lnGRDit*I(ωitG≤τ1G)+κ2lnGRDit*I(τ1G<ωitG<τ2G)+κ3lnGRDit*I(ωitG>τ2G)+ωit (3)
Uit=δ0+ηlnXit+ρ1lnBRDit*I(ωitB≤τ1B)+ρ2lnBRDit*I(τ1B<ωitB≤τ2B)+ρ3lnBRDit*I(ωitB>τ2B) (4)
where i denotes unit entity, t denotes time, u denotes explained variable, LnX denotes other variable that significantly affects explained variable, and I(•) denotes exponential function, ωit~idd (0, σ2). In the model (Equation 3), lnGRD is the explanatory variable affected by threshold variable, wit denotes intensity of government’s R&D funding, κ1, κ2, κ3, respectively denote influence coefficients of explain variable influence on explained variable when the threshold variables with the terms of ωit <τ1G,t1 <ωit <t2, ωit> t2,. In Equation 4, lnBRDit is explanatory variable affected by threshold variable. ωitB is the intensity of enterprises’ R&D investment. ρ1, ρ2 and ρ3, respectively denote the regression coefficients of explain variable influence on explained variable when the threshold variables with terms of ωitB<τ1B, τ1B<ωitB<τ2B, ωitB> τ2B.
Variable definition and data source
Variable definitions
(1) Kit and Lit: This paper takes perpetual inventory method to calculate the R&D funds stock Kit, Kit=(1-δ)*Kit-1+Eit. The R&D capital stock in 2003 is: Ki2003=Ei2003/(g+r); g denotes the region average annual growth rate of internal investment in R&D, r denotes depreciation rate, here δ=15% (Wu, 2006). While, Eit denotes the actual annual R&D expenditure in each region, the value takes reference to R&D expenditure price index (R&D expenditure price index = 0.55 × Consumer Price Index + 0.45 × fixed asset investment price index) fabricated by Zhu and Xu (2003); 2003 is the base period; it reduces the nominal R&D expenditure of internal expenses. R&D personnel investing takes definition of R&D staff in equivalent full-time in each region and every year.
(2) Patentit: In measuring R&D output, this paper selects the number of patents as assessment index. The patent number of Patentit is measured by the number of patents granted in China over the years.
(3) GRDit, BRDit, TMTit, Laborit, Infit and Openit: GRDit denotes government’s R&D funding and is expressed as the amount of government funds in each region and every year of a country's annual R&D expenditure. BRDit denotes enterprises’ R&D investment funds and is expressed as the amount of enterprise’s investment in each region and every year of a country's annual R&D expenditure. Technology Innovation Environment TMTit is measured by annual technical market total turnover in each region of China. The level of human capital Laborit is measured by average annual number of years of education in each region of China. This paper classifies education level into five categories: uneducated (0 years), primary education (6 years), junior middle school education (9 years), high school education (12 years) and college education (16 years), to denote the average age of education in each region (Laborit). The formula is: Labor=pri×6+jun×9+sen×12+col×16. Infrastructure level Infit is measured by annual post and telecommunications business in each region of China. Opening up level Openit is measured by the actual usage of foreign direct investment in each region of China.
Data sources
The empirical data of this paper are mainly from panel data of each region in China between 2003 and 2012. The amount of internal expenses incurred by R&D personnel, the total amount of R&D personnel, the R&D internal expenses, the enterprises’ funds and the government’s R&D funds are derived from the "China Science and Technology Statistics Yearbook"; the data obtained by per year of education are derived from "China Labor Statistics Yearbook". The total amount of the postal and telecommunications business, the consumer price index and the fixed asset investment price index are from "China Statistical Yearbook"; the actual usage of foreign direct investment is from the "China Statistics Yearbook "; some data are from regional statistical yearbook. In addition, due to the lack of data in Tibet Autonomous Region, the country data of China exclude Tibetan file data.
ANALYSIS OF EMPIRICAL RESULTS
Basic model test and analysis of empirical results
There is a certain time lag in the process of innovation from R&D investment to patent output. Liu and Guan (2002) set the lag time to 1 year, Furman et al. (2002) set to 2 years, Li (2009) studied and found that would take about 3 years between invention application to patent authorization in China. For the sake of comprehensive consideration, this paper will test the stochastic frontier model with four consideration of no time lag, lag 1 year, lag 2 years and lag 3 years. The regression results between the input and the output are lagging 0 year, lagging 1 year, lagging 2 years and lagging 3 years that are shown in models 1, 2, 3 and 4 (Table 1). From the estimation results, r2 and y of models 1 to 4 passed the 1% significance level test, which indicates that technology inefficiency is significant in the R&D innovation process. At the same time, it confirms the rationality of application of SFA technology in this paper. The regression coefficients of lnGRDit in models 1 and 4 are positive and the 1% significance level test shows that government’s R&D funding does not promote innovation efficiency, but does have significant negative effect. The main reasons for this analysis are: government’s R&D funding is too low. Government, as an important part in national innovation system, plays an important role in a country's innovation activities. The purpose of government's R&D funding is to guide the main direction of enterprises’ R&D investment, reduce cost of enterprises’ R&D investment, and solve the problem of unequal investment caused by technology spillovers between private investment and social benefits.
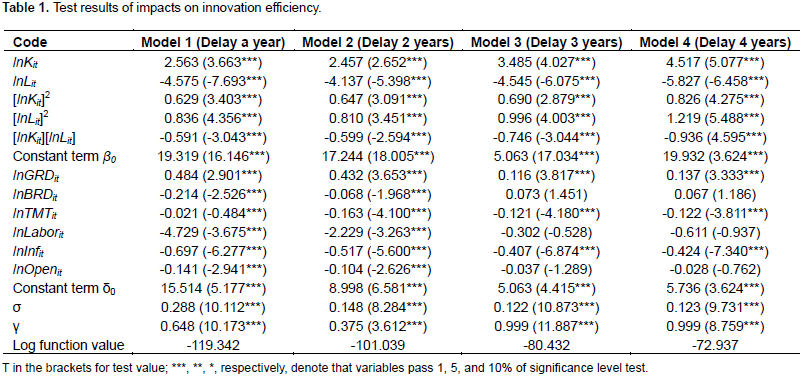
At present, because of lack of vitality of the market economy in China, enterprises’ R&D investment capacity and desire are inadequate; government, as a strong backing power for R&D activities, greatly needs to provide necessary and enough financial support for R&D activities. However, few government’s R&D funds result in lack of financial support for enterprises’ R&D activities and reduces their R&D scale, thereby reduces their research and development capabilities and affects the improvement of national innovation efficiency. On the other hand, the intensity of government’s R&D funding is too high. If the intensity of government’s R&D funding is too high, it will encourage the increase in demand for R&D resources and elements, but the shortage in the supply of scarce R&D resources will lead to increase in R&D decision-making elements, thereby increasing the cost of enterprises’ research and development. Some enterprises will give up part of R&D activities and turn to other profitable projects; this "crowding out" phenomenon is particularly serious in countries that lack R&D resources (Li and Wang, 2011). In short, inappropriate government intervention, that is, if government’s funding is too low or too high will both be detrimental to the improvement of innovation efficiency. The regression coefficients of enterprises’ R&D input variable lnBRDit in models 1 and 2 are significantly negative through the 5% significance test. The regression coefficients are positive in models 3 and 4, but not significant, which indicates that enterprises’ investment has played a catalytic role in improving innovation efficiency. With the improvement of economic development level and technical level, Chinese market economy is becoming increasingly active and dynamic; enterprises’ R&D investment is gradually increasing. The capabilities and level of R&D are continuously improving. Chinese market economy develops very stable, "market failure" phenomenon appears less, enterprises can accurately and completely grasp market information, reasonably adjust their own R&D funds scale to ensure a reasonable proportion of enterprise R&D investment, making the level of innovation efficiency of the whole country gradually improve.
But, with the deepening of government’s intervention, the influence of "market failure" on R&D innovation has been effectively regulated by government policy strategy, and enterprises’ R&D investment can guarantee correct investment direction and investment scale under the guidance of government policy. To ensure that enterprises’ R&D funds can be invested into high level of innovation and with scarce R&D resources areas, the overall efficiency of Chinese innovation needs to be improved. Among other explanatory variables, lnTMTit, which represents the technology innovation environment, lnLaborit represents the human capital level, lnInfit represents the infrastructure level, and lnOpenit represents the opening up level. In the four models of the regression coefficients, the above four coefficients are significantly negative; it indicates that they significantly promote the improvement of innovation efficiency; the better the technological innovation environment, the higher the level of human capital; the better the conditions of infrastructure, the higher the level of opening up and the innovation efficiency. The analysis shows that R&D investment can promote the improvement of innovation efficiency level, but the impact of government’s R&D funding on innovation efficiency is negative. It can be seen that the intensity of enterprises’ R&D investment in China is relatively reasonable, but government’s R&D funding is with too low or too high proportion. Is there an optimal interval between them that can make our innovation efficiency to be optimal? To this end, this paper continues to use the valve regression model to test the existence of strength of government’s R&D funding and enterprises’ R&D investment strength; it further discusses government’s R&D funding strength and enterprises’ R&D investment strength threshold characteristics. Finally, there is a comprehensive analysis on the optimal interval of government’s R&D funding strength and enterprises’ R&D investment strength.
Threshold model test and empirical analysis
Through the previous analysis, this paper further explores whether there is an optimal interval in the process of government’s R&D funding and enterprises’ R&D investment influencing innovation efficiency to minimize the "market failure" and "government failure" to ensure maximum innovation efficiency. In order to test the intensity of government’s R&D funding and the optimal range of enterprises’ R&D investment intensity, then we need to improve the innovation efficiency of China. This paper will take the intensity of government’s R&D funding and enterprises’ R&D investment as the threshold variables.
Threshold test
Firstly, in order to determine the number of thresholds, we need to examine the threshold effect. This paper examines models 3 and 4 in the absence of the threshold, the existence of a threshold, the existence of a double thresholds and the existence of a triple thresholds. The specific test results are shown in Table 2. It can be seen from Table 2 that the threshold effect test shows that the intensity of government’s R&D funding in model 3 and the intensity of enterprises’ R&D investment in model 4 are significantly different from each other. The regression results of each threshold are shown in Table 3. It can be seen from Table 3 that the wG thresholds of model 3 are respectively estimated by Bootstrap to be 0.161, 0.208 and 0.282. According to the threshold value, the innovation efficiency is affected by different government subsidy intensity. The intensity of government’s R&D subsidy can be divided into four intervals: low government’s R&D subsidy intensity (wG<=0.161), sub-low government’s R&D subsidy intensity (0.106<wG<=0.208), sub-high intensity of government’s R&D subsidy (0.208 <wG<0.282) and high intensity of government’s R&D subsidy (wG>0.282). Similarly, the respectively estimated value by Bootstrap of wB from model 4 is 0.688, 0.775 and 0.838. According to the threshold, it can be seen that the intensity of enterprise’s R&D investment that can affect innovation efficiency can be divided into four intervals: low enterprises’ R&D investment intensity (wB<=0.688), sub-low enterprises’ R&D investment intensity (0.688<wB <0.775), sub-high intensity of enterprises’ R&D investment (0.775<wB<0.838) and high intensity of enterprises’ R&D investment (wB>0.838).
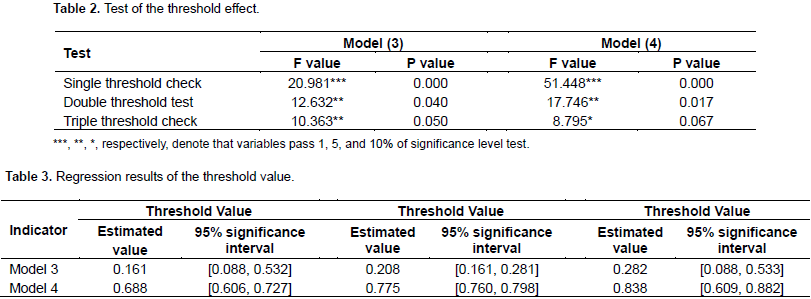
Parameter estimation and empirical results analysis
The threshold variables are added to models 3 and 4 and regression analysis. The results are shown in Table 4. The test results of model 3 show that the negative effect of government subsidy on innovation efficiency can be reduced only when the intensity of government’s R&D funding breaks through certain thresholds, thus reduce the impact of "government failure" on innovation level. Specifically, when the intensity of government’s R&D funding is less than 0.161, although the negative impact of government’s R&D funding on innovation efficiency is the smallest, the elasticity coefficient is -0.161; but because of the current government funding in our country has been basically higher than this ratio, here we need to seek a higher proportion. When the intensity of government’s R&D funding reaches 0.161 to 0.208, the negative effect of government’s R&D funding on innovation efficiency is larger, and its elasticity coefficient is -0.216. However, when the intensity of government’s R&D funding is 0.208-0.282, the negative effect of government’s R&D funding on innovation efficiency is reduced and the elasticity coefficient is increased to -0.197. When the intensity of government’s R&D funding reaches 0.282, the negative effect of government’s R&D funding in the innovation process is relatively small, its elasticity coefficient is -0.177; the elasticity is lower than the first interval, but the gap is small, and the government’s R&D subsidy strength is also in line with the current development trend of innovation efficiency in China.
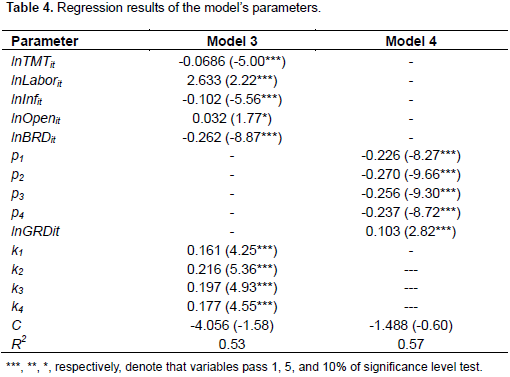
The regression of model 4 shows that it is obvious that there is threshold for the impact of enterprises’ R&D investment on innovation efficiency. Overall, only the enterprises’ R&D investment intensity is in a reasonable range; the enterprises’ R&D investment can promote the innovation efficiency to a full role; when the enterprises’ R&D investment intensity is too low or too high, it will both reduce the positive effects of enterprises’ R&D investment on innovation efficiency. Specific performance is: when the enterprises’ R&D investment intensity is lower than 0.688, enterprise cuts down investment in R&D innovation; though enterprises’ R&D investment on the innovation efficiency is relatively small, its flexibility coefficient is only 0.226; when the enterprise R&D investment strength increased to 0.688-0.775, enterprises’ R&D investment promote the innovation efficiency to be optimal; the significantly elastic coefficient is 0.270. However, when the intensity of enterprises’ R&D investment increased to 0.775-0.838, the role of enterprise’s R&D investment in innovation efficiency began to decline; and when the intensity of enterprises’ R&D investment exceeds 0.838, the promotion of enterprise’ R&D investment to innovation efficiency is weakened, and its elasticity coefficient is reduced to 0.237, but still higher than the elasticity of first interval is 0.226. Based on the threshold characteristics of government’s R&D and enterprises’ R&D investment, this paper argues that China is currently setting the intensity of government’s R&D funding above 0.282, and the intensity of enterprises’ R&D investment is controlled between 0.688 and 0.775. This can reduce the "market failure" and "government failure" on the innovation efficiency, and thus improve the overall level of innovation in China.
CONCLUSION AND POLICY RECOMMENDATIONS
This paper summarizes the impact mechanism of government’s R&D funding and enterprises’ R&D investment on innovation efficiency from the perspective of "double failure". Then, this paper analyzes the impact of government’s R&D funding and enterprises’ R&D investment on innovation efficiency based on the data of Chinese region panel data between 2003 and 2012. On the whole, the impact of government’s R&D funding on innovation efficiency is negative, and enterprises’ R&D investment can promote the improvement of innovation efficiency. This shows that in Chinese overall innovation system, there is a certain degree of "government failure", but less "market failure" phenomenon. At present, the optimal interval of intensity of government R&D funding based on over 0.161, and over 0.282, and the optimal range intensity of enterprises’ R&D investment is between 0.668 and 0.775. And further, the threshold regression method is used to define government R&D funding and enterprises’ R&D investment of the optimal strength. This study finds that only the government’s R&D funding and enterprises’ R&D investment is in a reasonable interval, that is, the intensity of government R&D funding is above 0.282, enterprises’ R&D investment intensity is constrained into 0.668 and 0.775, in order to minimize the impact of "government failure" phenomenon on innovation efficiency, and improve the role and importance of enterprises’ R&D investment in promoting innovation efficiency. Therefore, we should fully understand the role of government and the market- mechanism in the innovation system, providing rich amounts of funds resources and a good environment for the purpose of Chinese innovation well developed. First of all, the government should continue to expand the scale of government’s R&D funds, so that it is close to the level of funding in developed countries to ensure the intensity of government R&D funding in a reasonable range, and prevent the occurrence of inefficient "government failure" phenomenon. Secondly, it should increase the support of strength to innovative enterprises, improve enterprises’ innovation investment preferential policies to mobilize the enthusiasm of enterprises’ R&D investment; at the same time, enterprises should strengthen their own R&D investment, introduce external advanced technology and high-quality personnel, improve the competitiveness of their products, and fully play their own role in the innovation system.
The authors have not declared any conflict of interests.
This project (2018M631557) was supported by China Postdoctoral Science Foundation.
REFERENCES
Bai J, Jiang K, Li J (2009). Using the stochastic frontier model evaluating regional innovation efficiency of China. Management World, 10:51-61.
|
|
Battese GE, Coelli TJ (1995). A model for technical inefficiency effects in a stochastic frontier production function for panel data. Empirical Economics, (20):325-332.
Crossref
|
|
Cheng H, Zhao X (2009). Size of enterprise, intensity of R&D, intensity of subsidies, and government subsiies performance—Empirical study on Zhejiang private science and technology enterprises. Science Research Management, 29(2):37-43
|
|
Czarnitzki, D., Licht, G (2006). Addtionality of public R&D grants in a transition economy, the case of eastern Germany. Economic Transiton, 14(1):101-131.
Crossref
|
|
Fan G, Wang X, Ma G (2011). Contribution of marketization to China's economic growth. Economic Research Journal, 2:4-16.
|
|
Fu X, Wu L (2007). The applicability of the frontier analysis method of accounting in China's economic growth. Journal of World Economics 7:56-66.
|
|
Furman JL, Porter ME, Stern S (2002). The determinants of national innovative capacity. Research Policy, 31(6):899-933.
Crossref
|
|
Goolsbee A (1998). Does government R&D policy mainly benefit scientists and engineers?. American Economic Review, 88:298-302.
Crossref
|
|
Guellec D, Van Pottelsberghe B (2003). The impact of public R&D expenditure on business R&D. Econ. Innovative New Technology 12(3):225-243.
Crossref
|
|
Hansen BE (1999). Threshold effects in non-dynamic panels: Estimation, testing and inference. Journal of Economics, 93:345-368.
Crossref
|
|
Jiang J, Qi J (2007). The tax policy effect for promoting enterprise' R&D investments. China Soft Science 8:65-84.
|
|
Kang Z (2013). Technology choice, input intensity, and enterprise innovation performance. Science Research Management 34(6):42-49.
|
|
Klette TJ, Moen J, Griliches Z (2000). Do subsidies to commercial R&D reduce market failures? Microeconomic Evaluation Studies. Research Policy, 29:471-495.
Crossref
|
|
Li P, Wang C (2010). The optimal level of public direct subsidies to business R&D and the targeted project select: A threshold regression analysis based on the industrial heterogeneity. Review of Industrial Organization, 9(3):37-53.
|
|
Li P, Wang C (2011). The contribution of public R&D organization to the innovation in China. Science Research Management, 9:15-21.
|
|
Li XB (2009). China's regional innovation capacity in transition: An empirical approach. Research Policy, 31(6):899-933.
Crossref
|
|
Liu S, Guan J (2002). The evaluation on the innovating performance of regional innovation systems. Chinese Journal Management Science, 1:75-78.
|
|
Liu X (2000). The impact of the structure of China's industrial enterprise's ownership on its efficiency. Economic Research Journal, 2:17-25.
|
|
Miao S (2011). On the spillover effect of university R&D on enterprise technological innovation in China. Science Research Management, 6:11-17.
|
|
Perkmann M, Walsh K (2009). The two faces of collaboration: Impacts of university-industry relations on public research. Industrial Corporation Change, 18(6):1033-1065.
Crossref
|
|
Schreyer P (2000). The contribution of information and communication technology to output growth: A study of the G7 countries. OECD Science, Technology and Industry Working Papers, OECD Publishing.
Crossref
|
|
Spence M (1984). Cost reduce, competition, and industry performance. Econometrica 52(1):101-121.
Crossref
|
|
Wang J (2011). Comparison on R&D output elasticity about government and enterprise. The Journal of Quantitative & Technical Economics, 6:93-106.
|
|
Wu Y (2006). R&D stock, knowledge function and productive efficiency. China Economy Quarterly, 4:1129-1156.
|
|
Xing Y (2004). Market failure-The reason of government's regulation of high-tech industry. East China Economics Management, 5:60-63.
|
|
Yan C, Gong L (2013). R&D ratio, R&D structure and economic growth. Nankai Economics Studies, 2:3-19.
|
|
Yue H, Zhang L, Liang L (2002). A comparative study on age structure between NSFC project principals and S&T research personnel. Science Research Management, 6:100-106.
|
|
Zhu D, Pan F, Gu X (2014). The contrastive analysis of science and technology R&D affect profitability between small and medium—sized enterprise. Statistics Decision, 17:180-181.
|
|
Zhu P, Xu W (2003). On the impact of government's S&T incentive policy on the R&D input and its patent output of large and medium-sized industrial enterprises in Shanghai. Economics Research Journal, 6:45-53.
|