The recent global financial crisis has caused the failure of many firms in several countries, renewing the interest of the literature towards forecasting models for default risk. Although these models have been developed since the 1960s, studies have been published in increased numbers during the last decades, proposing new approaches or comparing different existing models to understand which of them have the best predictive power. However, the recent financial crisis has underlined how important adopting early warning systems are. Although forecasting business failure and implementing warning systems are conceptually different, there is a risk of overlapping these concepts. Accordingly, this study aims to review the literature on these topics by using a conceptual review methodology, at the same time considering the trend to implement approaches aiming to facilitate corporate rescue.
As stated in the previous section, this study aims to review the literature, focusing not only on models suggested by researchers to forecast bankruptcy and assess the default risk, but also on early warning systems, whose aim being to prevent bankruptcy. As underlined in the previous section, forecasting models and early warning systems have been considered frequently as synonym; more specifically, taking into account that the objective to be achieved through the implementation of forecasting models is to select a set of indicators that best foresee distress, scholars have considered them as triggers events which, in turn, have been interpreted as warning signals. However, the expression ‘early warning system’ has a different meaning, since it is not focused only on the output of a model (namely indicators or financial ratios considered as warning signals or red flags) but also means implementing a procedure able to avoid the distress. This preliminary consideration helps us while selecting the appropriate methodology to be adopted while reviewing the existing literature. According to Jesson et al. (2013), the literature review format depends on the purpose of the review, hence the process defines the type of the review being adopted over a continuum of approaches from the so-called traditional reviews to the systematic reviews. The ‘traditional review’ methodology is based on an iterative approach to search relevant articles, rather than a comprehensive and replicable search for a specific question (as in the case of systematic review approach). Although the traditional review could be considered too subjective, due to the personal selection of articles by the writer, this does not automatically imply that this approach is not scientific. Indeed, a traditional review is not merely descriptive, but is based on a critical approach, seeking to add new insight on the topic.
Accordingly, both traditional and systematic reviews address a research question or problem, even if they use a different methodological approach. Having clarified that, it is worth observing that scholars have used different expressions to identify traditional review approaches, such as Jesson et al. (2013):
(1) Traditional review, frequently based on a critical analysis of methods and results of previous studies, with a focus on contextual materials;
(2) Conceptual review, which aims to synthesise conceptual knowledge in order to contribute to a better understanding of the investigated issues;
(3) State-of-the-art review, which intends to bring readers up to date on the most recent studies on the topic being investigated;
(4) Expert review, namely a review written by a recognized expert; and
(5) Scoping review, which depicts the scene for a future research agenda.
Bearing in mind the different aims to be pursued through these approaches, this study adopts a conceptual review methodology, which is particularly appropriate when the aim of the review is ‘to compare and contrast the different ways in which authors have used a specific word or concept’ (Jesson et al., 2013). Therefore, this approach is particularly useful since a risk of confusing and overlapping the expressions ‘forecasting models to predict distress’ and ‘early warning systems to prevent distress’ do exist since they have been used interchangeably (see, for example, the recent study of Wieprow and Barlik, 2017). Accordingly, this study aims to underline the conceptual differences between the above-mentioned expressions, contributing to a better understanding of these core concepts.
Forecasting models for default risk
The prediction of firm financial distress has been largely investigated since the 1930s. The first studies were based on the use of ratio analysis to predict bankruptcy, evolving from univariate models (Beaver, 1966) to multivariate studies, with the best-known paper by Altman (1968). Following these seminal papers, several approaches have been developed, aiming at proposing more accurate and robust models to predict bankruptcy. More concretely, researchers have mostly implemented models based on a comparison between healthy and distressed firms (Du Jardin, 2010; Amendola et al., 2011), trying to select the best failure indicators. The approaches suggested by researchers for predicting business failure can be classified looking at both the model types used for distress forecasting and the factors that influence the risk of default (that is, the financial indicators selected in the study), as well as considering their different levels of predictive accuracy.
Forecasting model types: Main findings
The great depression of 1929 and the recent financial crisis that began in 2008 caused a significant increase in the number of companies in danger of bankruptcy, stimulating research on bankruptcy prediction. The literature classifies forecasting models into two categories (Alaka et al., 2018). The first one consists of statistical models, which analyse two samples of healthy and distressed firms, and where the selection of financial ratios having a predictive ability is based on empirical studies. The selected ratios are then used to estimate the parameters of the model and the probability of default. A drawback of these models is that they typically rely on some restrictive assumptions (Korol, 2013):
(1) Variables (that is, financial ratios) should have normal distributions, they must be independent and must have a high discriminative ability to separate healthy companies from distressed ones;
(2) Values for all indicators of all firms are required, that is, information for each unit (healthy and distressed firms) must be complete, in the sense that there are no missing values for any variables; and
(3) Classification of firms must be clearly defined (that is, a firm belonging to one group precludes its belonging to a different group).
The second group of approaches used to forecast the risk of default is based on soft computing techniques, whose primary assumption is that data can be incomplete and environmental conditions can change over time. Accordingly, these methods are designed to take into account that some parameters may be affected by changing environmental conditions; therefore, these models are dynamic and are often labelled as learning systems.
Statistical models
Statistical models consist of a set of approaches, which aims to estimate the probability of default and select the best predictors (namely ratios calculated on financial statements’ items) of bankruptcy. The most straightforward models were primarily based on univariate approaches. The first studies were proposed by Smith (1930) and FitzPatrick (1932). Smith (1930) investigated a sample of 29 failed firms belonging to different sectors, referring to 24 ratios, while FitzPatrick (1932) examined a sample of 20 companies, calculating 13 ratios. Several ratios were founded as good indicators of the unhealthy financial conditions of firms, notably Working Capital to Total Assets, Net Worth to Total Assets, Net Worth to Debt and Net Profits to Net Worth. In the following years, other studies were published, representing the fruitful groundwork for further investigations. In particular, the research of Merwin (1942) was based on a large sample of 581 small firms for the period 1926-1936. Three ratios (Working capital/total assets; Net worthy/total liabilities; Current assets/current liabilities) were selected because of their high predictive ability. The article of Beaver (1966) represented the most widely recognised univariate study within the literature on bankruptcy prediction. He investigated a paired sample composed of 79 failed and 79 non-failed firms for the period 1954 to 1964. Thirty ratios, classified into six categories (1. Cash flow ratios; 2. Net-income ratios; 3. Debt to total assets ratios; 4. Liquid-assets to total assets ratios; 5. Liquid asset to current debt ratios; 6. Turnover ratios), were selected according to their relevance and adoption in previous studies.
Comparing the mean of these ratios, Beaver tested their predictive ability in classifying failed and non-failed firms: Net income to Total Debt gained the higher predictive ability, followed by Net Income to Sales, Net Income to Net Worth, Cash Flow to Total Debt and Cash Flow to Total Assets. Through a Dichotomous Classification Test, Beaver defined a cut-off point to minimise classification errors and correctly classify firms as healthy or distressed. The research of Beaver was more rigorous than previous studies and underlined the importance of ratios as default risk estimators; however, as further development, Beaver suggested testing the predictive ability of multiple ratios instead of a single ratio. Therefore, forecasting models for default risks started to evolve, being based on the multivariate discriminant analysis. In general, the aim of a multivariate discriminant analysis is to classify the observations into two (or more) groups, minimising the classification errors (more specifically, two misclassification errors can occur: a type I error, which means that a failing firm is misclassified as a healthy firm, or a type II error, which means that non-failing firm is misclassified as a failing firm). This goal is achieved by adopting the decision rule of maximising the between-group variance relative to the within-group variance. Following this rule, the discriminant score calculated for each firm is compared to an optimal cut-off value, in order to determine the group to which the firm belongs. If this score is less than the cut-off point, the firm is assigned to the failing group; otherwise, it is assigned to the non-failing group (Amendola et al., 2011). The study of Altman (1968) can be considered as a milestone in the field since he is the first author who applied discriminant analysis to the prediction of business failure. He developed the so-called ‘Z-score model’, a five-factor model to forecast bankruptcy of manufacturing firms, and investigated the financial statements of a sample of 66 companies, divided into two groups (failed and non-failed firms) through the estimation of a linear combination of five variables:
Z = 0.012 X1 + 0.014 X2 + 0.033 X3 + 0.006 X4 + 0.999 X5
where:
X1 = Working capital / Total assets;
X2 = Retained earnings / Total assets;
X3 = EBIT / Total assets;
X4 = Market value of equity / Total liabilities; and
X5 = Sales / Total assets.
His model showed a higher predictive ability compared with previous models one year before bankruptcy; however, this capacity was considerably lower in two to five years before failure: for example, the type I error varies from 6% (one year before failure) to 28% (two years before failure). The cut-off point (Z = 2,675) was calculated as a mean of the average values of z-score for both groups, which allowed for reducing the misclassification errors. The author also defined a grey area that takes into account any uncertainty when classifying firms. Altman further enhanced and improved his model (Altman et al., 1977; Altman, 2000), also considering the Basel 2 environment (Altman and Heine, 2002). Studies on bankruptcy prediction increased considerably after Altman’s research, and several statistical models have been developed, such as logit and probit regression, and survival analysis. Logit analysis is used when the dependent variable is binary, assuming only two values, 0 and 1, which in our case represent healthy and failed firms. The use of logistic regression is a way of overcoming restrictive assumptions of discriminant analysis, such as normality and equal covariances between groups. Among the studies that use the logit model for the business failure prediction, we mention the most relevant. Ohlson (1980) pioneered the use of logit analysis in failure prediction. He analysed a sample of 105 failed firms and 2,058 non-failed firms in the period 1970 to 1976, and the predictive ability of the model, based on nine ratios, was over 92% of the bankrupt firms two years earlier. Therefore, the logit model predicting corporate failure performs well.
Zavgren (1985) investigated a sample of industrial firms for predicting bankruptcy 1-5 years in advance. His model was based on seven indicators and used factor analysis to obtain the independent variables for the logit model. Its predictive ability for one-year prediction was about the same as Ohlson (1980). The error rates for longer predictions were similar to or slightly lower than those reported in the previous bankruptcy prediction studies using multivariate discriminant analysis. Peel (1987) examined a sample of non-listed firms, and the predictive ability of the model was satisfactory in the last five years before the bankruptcy. Finally, the highest predictive ability (around 98%) was achieved by Dambolena and Shulman (1988), whose model was based on fourteen ratios. The main problem of discriminant analysis and logistic regression is that they are cross-sectional models and assume that the underlying failure process remains stable over time. This assumption is usually violated in the real world (Luoma and Laitinen, 1991). Therefore, researchers have suggested using survival analysis to forecast business failure. Survival analysis differs from the other approaches since it deals with business failure prediction, not as a classification problem, but as a timeline, representing firms through lifetime distributions (Gepp and Kumar, 2008). Accordingly, survival analysis is more flexible, allowing for taking into account ‘the relationship between survival and a set of explanatory (prognostic or covariate) variables, (which) may also describe changes in the status as a function of time’ (Luoma and Laitinen, 1991). The pioneering study on survival analysis for financial distress prediction was the paper of Lane et al. (1986). They found that the use of Cox models was empirically comparable to discriminant analysis, but with fewer Type I errors. Luoma and Laitinen (1991) achieved less satisfactory results than those of discriminant analysis. Then the accelerated time survival analysis proposed by Shumway (2001) got better results compared to those of previous approaches. However, as Gepp and Kumar (2008) have underlined, comparing time-series survival analysis with cross-sectional models is not easy. Indeed, only the former takes into account the longitudinal nature of business failure prediction, while the latter are static models (Shumway, 2001) de facto ignoring that firms can change over time.
Soft computing models
Soft computing models (also named artificial intelligence models) try to deal with the incompleteness and uncertainty of data, as well as changes affecting them, because of evolving environmental conditions. Accordingly, while variables entered into statistical models are supposed to be reliable, accurate and precise, soft computing models accept that data may be partially inaccurate and imprecise. In so doing, these models take into account that precision and certainty mean sustaining costs and that the decision-making process is expected to tolerate uncertainty and approximation of data. Several approaches can be included in the artificial intelligence models, such as the recursive partitioning algorithm (RPA), genetic algorithms, and neural networks. After some applications in the medical context, scholars (Marais et al., 1984; Frydman et al., 1985) implemented RPA to forecast bankruptcy risk. The model of Marais et al. (1984) was based on both financial and non-financial indicators and aimed to estimate the misclassification costs. Frydman et al. (1985) investigated a sample of 200 firms (58 failed and 142 healthy) in the period 1971-1982, implementing both RPA and the classical multivariate discriminant analysis, and compared their predictive ability. The results showed that RPA on average performed better than the discriminant analysis. Genetic algorithms (GAs) try to imitate the Darwinian logic of evolution through a natural selection while selecting predictors of failure. This approach was first implemented to forecast bankruptcy by Kingdon and Feldman (1995), according to whom GAs can be considered as a class of probabilistic optimisation techniques.
More specifically, GAs are similar to the Monte Carlo simulations, drawing a set of inputs from different domains randomly and calculating a result from these inputs. These results, in turn, are used to measure the fitness by using a specific function, with the main aim being to evolve to better solutions (Egan, 2007). GAs are frequently used in conjunction with artificial neural networks (ANNs), whose implementation to forecast bankruptcy progressively increased in the last decades.ANNs consist of different layers of nodes, starting from one (or several) layers in the input, at least one hidden layer and final output. The set of inputs is used to train the network so as to get a target output by changing the weights assigned to the different nodes. Several studies were published (Rahimian and Singh, 1993; Serrano-Cinca, 1997; Laitinen and Kankaanpaa, 1999; Zhang et al., 1999; Charalambous et al., 2000; Neves and Vieira, 2006), sometimes comparing results with those of the classical discriminant analysis. The predictive accuracy of network models is, on average, quite high (Serrano-Cinca, 1997; Laitinen and Kankaanpaa, 1999; Zapranis and Ginoglou, 2000), although their generalizability can be lower than that of multivariate discriminant analysis because they require a high number of parameters to improve predictive accuracy. For this reason, researchers have suggested simplifying neural network (Sen and Stivason, 2004).
Forecasting model ratios: Main findings
Models adopted in previous studies can be classified in accordance with the selection of factors, that is, financial ratios used to predict bankruptcy. These factors are usually classified into several groups, expressing the performance of a firm (Maksimovic and Phillips, 2001), its liquidity and solvency (Rege, 1984), the leverage (Heiss and Köke, 2004), the size (Bethel et al., 1998; Heiss and Köke, 2004) and so on. It is also worth noting that many studies have classified ratios into several categories. The most common are:
(1) Profitability ratios, such as Return on Equity (ROE), Return on Assets (ROA), Return on Sales (ROS), Net Income to Total Assets, Net Income to Total Debts, Sales to Fixed Assets, Finance Charges to Net Sales and so on;
(2) Liquidity and solvency ratios, such as Current Ratio, Quick Ratio, Current Assets to Fixed Assets, Inventory to Current Assets, Working Capital to Total Assets, Liquid Assets to Total Assets, Cash Flow and so on;
(3) Size and capitalisation, such as Total Assets, Long-term Assets to Total Assets, Net Worth to Fixed Assets, Net Worth to Total Debts, Total Debts to Total Assets and so on;
(4) Turnover ratios, such as Inventory to Sales, Accounts Receivable to Sales, Accounts Payable to Sales, Total Debts to Sales; and
(5) Operating structure ratios, such as Labour Cost to Production Cost, Labour Cost to Net Sales, Finance Charges to Debt, Finance Charges to Financial Debt.
The primary aim of this classification is to facilitate the interpretation of ratios, supporting the selection of those having a good predictive ability. Additionally, several recent studies (that is, Liao and Mehdian, 2016 and references therein) have adopted an aggregation process, to build an aggregate bankruptcy index which ranges between 0 and 1, ranking firms by their relative financial distress. Several studies have also found that corporate governance indicators influence the prediction of bankruptcy (Liang et al., 2016; Bredart, 2014; Chen, 2014). These indicators can be classified into five groups, including board structure, ownership structure, cash flow rights, key person retained and others. Even if some authors (Lin et al., 2010; Chen, 2014) have shown that combinations of financial ratios and corporate governance indicators can improve models’ performance, there is not a unanimous consensus on the best set of variables to be used in the model estimation for predicting business failure. The choice of the most significant predictors usually depends on some details, such as the aim of the study, the activity sector under investigation and the data availability (Amendola et al., 2017).
Early warning systems and safeguard procedure: Main findings
The models aiming to forecast bankruptcy, described in the previous section, adopt different approaches (statistical vs. soft computing techniques) and select ratios to be used as predictors of financial distress. Notwithstanding the differences, these models are substantially based on an ex-post perspective, comparing samples of distressed and healthy firms, namely firms whose status is already known. Accordingly, these models are not necessarily able to support the decision-making process of practitioners who are involved (or are going to be involved) in transactions with a firm experiencing a decline. Indeed, as previously stated, financial distress is a dynamic process (Volkov et al., 2017) which evolves over time, and bankruptcy is only the final step when there are no other alternatives. This would mean that other ways of exiting the market should be taken into consideration, to understand and assess whether a troubled firm has future chances of survival by implementing a restructuring strategy. Consistently, legislation in many countries is evolving in the direction of introducing timely intervention, sometimes without the direct involvement of the courts, with the main aim being corporate rescue. Focusing on the European context, notable legislative innovations in insolvency laws have taken place in countries like Germany (1999 and 2012), England (Enterprise Act 2002), Poland and Romania (2003 and 2006), Spain (2004 and 2013), France (2006 and 2014), Finland (2007), Greece (2007 and 2012; see Paulus et al., 2015); Italy (2017) and so on. Although several differences still exist between these legislations, a common orientation towards corporate rescue can be observed, as an alternative to liquidation procedures (Tollenaar, 2017).
The EU itself has recently proposed a new directive, aiming to implement preventive restructuring frameworks, in order to give firms a second chance and increase the efficiency of restructuring, insolvency and discharge procedures. Even if this proposal has been criticised from a juridical perspective (Tollenaar, 2017), from an economic point of view, it intends to facilitate timely interventions. From a theoretical viewpoint, this would mean investigating and implementing models to prevent financial distress. Indeed, in the light of a timely intervention, the main problem is not to forecast default risk but mainly to prevent it. Accordingly, from a practitioners’ perspective, we would argue that the central point is to understand how a safeguard procedure can be implemented and how its efficiency can be assessed. France has a long tradition of implementing such a procedure. The legislation is specifically designed to stimulate managers to become aware of the financial difficulties of the firm at an early stage, and consequently to adopt the necessary measures to recover the position. The legislation regulates three forms of pre-insolvency situations (Kastrinou, 2016): the safeguard-preservation procedure (sauveguarde), the conciliation procedure (conciliation) and the ad hoc mandate (mandate ad hoc). The first procedure is based on a safeguard plan, which could provide several solutions to overcome the financial difficulties, such as a change in the control of the company, the sale of certain assets or dismissal of a business area, a rescheduling of the debt and so forth. The principal aim of the safeguard-preservation procedure is to stimulate managers to take a timely initiative to rescue the firm; they have to demonstrate the difficulties their company is experiencing, asking to begin the procedure to solve them. However, there is a fundamental pre-condition to start the procedure: the firm cannot be insolvent. The principal actor is an administrator, appointed by the Court, who is expected to serve as a sort of temporary manager, with the main aim being to assist the management of the firm in redesigning the strategy and implementing the plan.
The conciliation procedure aims to facilitate the rehabilitation of firms experiencing legal, financial or economic difficulties. While the safeguard procedure requires that firms not be insolvent, the conciliation procedure asks for a more precise requirement: firms can access the conciliation if they have ceased payments for no more than 45 days (Kastrinou, 2016). The Court appoints a conciliator, who supports the management of the troubled firm to negotiate with creditors in order to conclude an agreement, whose primary aim is to guarantee the going concern of the firm.
The ad hoc mandate intends to ensure the rescue of a distressed firm. It starts at the initiative of the firm, asking the Court to appoint a mandatee; the request must be based on a plan, where managers illustrate the measures to implement, in order to restructure the business and to repay the firm’s debts. The ad hoc mandate is less formal and more flexible than the safeguard procedure, which means that achieving an agreement with the creditors should be facilitated. Other contexts provide similar procedures: for example, the UK’s insolvency law provides the company with a voluntary arrangement, a debtor in possession procedure which aims to make easy the rehabilitation of firms experiencing financial difficulties. A recent reform (2017) approved in Italy has regulated a procedure close to the French ‘sauveguarde’, providing a new tool to firms experiencing financial difficulties, in order to make a timely intervention possible. More generally, it is worth recalling that, in the EU context, a proposal of a new directive has been approved, with the main aim being to give firms experiencing financial difficulties a second chance, supporting them in restructuring the business. This directive also intends to harmonise the legislation, considering that several relevant differences can be observed in the European context. The literature has paid comparatively little attention to these timely – intervention procedures: while a large number of studies have investigated models to forecast bankruptcy, early warning systems seem to be under-investigated. Indeed, scholars have analysed safeguard procedures principally by adopting a judicial perspective (Kastrinou, 2016; Paulus et al., 2015; Tollenaar, 2017), aiming at investigating legal problems (such as the absolute priority rule) rather than the economic and financial implications of the tools being investigated, with a few exceptions (Di Carlo et al., 2009) but focused on specific contexts.
Forecasting model types: Discussion of main findings
It is noteworthy that newer and more complex models do not necessarily have a higher predictive ability than older and simpler ones (Bellovary et al., 2007); moreover, in several studies, the highest success rates have been shown by both multivariate discriminant analysis and neural network models. This means that both statistical methodologies and soft computing approaches allow for achieving good results, although the second ones are more complex than the first. Along this line of thought, Alaka et al. (2018) propose an integrated framework based on 13 criteria (including accuracy, result transparency, ability to use and small sample size), emphasising that the selection of an appropriate tool to predict bankruptcy should be based on its strengths and weaknesses rather than on its popularity (as has frequently occurred). However, it should also be observed that it is not easy to compare models, taking into account that different definitions of business failure have been adopted. While many papers define this concept as actually filing for bankruptcy, others adopt larger definitions, considering as “unhealthy” all firms that are suffering financial distress or are not able to pay obligations. It is also important to note that bankruptcy is only one of the possible ways of exiting the market, as Schary (1991) has pointed out. Accordingly, researchers (Esteve-Pérez et al., 2010; Chancharat et al., 2010; Balcaen et al., 2012; Jones and Hensher, 2007; Amendola et al., 2015) suggest focusing on the different forms of exiting the market, considering not only bankruptcy but also voluntary liquidation as well as merger and acquisition (M&A), which constitute out-of-court exit procedures. These studies have appreciably improved our knowledge in this field, highlighting that these different forms of exit are likely to be caused by various factors.
It should be further observed that the vast majority of previous studies, with few exceptions (Amendola et al., 2017), have adopted an ex-post perspective, testing the predictive accuracy of the model they propose on a sample of failed and non-failed firms, that is, firms whose status is already known. However, in several circumstances (e.g. when a bank is going to decide whether or not to lend money to a firm), an ex-ante perspective is required. Therefore, there is a need to investigate from an ex-ante viewpoint the probability of moving from healthy status to another (for example, liquidation or bankruptcy), and scholars are highly encouraged to examine these issues by adopting a dynamic approach.
More specifically, considering the above and bearing in mind that the failure of a company is a process which evolves over time, we would argue that several statuses should be taken into consideration while forecasting the risk of default:
(1) Default of payment, which occurs when a firm (more generally, a debtor) starts not to pay its debts regularly;
(2) Insolvency proceedings, which occur when a firm is unable to pay its debts. In this case, even if the insolvency is declared, the firm remains active, though it is in administration or receivership or under a scheme of arrangement (US - Chapter 11). During this period, the firm is usually placed under the protection of the law, continues operating and repaying creditors, while trying to reorganise its operating activities. At the end of the procedure, the firm will alternatively (a) return to normal operating (the default of payment was thus temporary), (b) be reorganised (parts of its activity can be restructured or sold) or (c) be liquidated;
(3) Bankruptcy, which occurs when a firm is formally declared distressed since it is not able to pay its creditors. The court will appoint an insolvency expert, whose main aim is to sell the assets and repay the debts. At the end of the procedure, the firm will be dissolved; and
(4) In liquidation, which occurs when all the assets of the company are being sold, and the firm will be dissolved.
Considering these steps is essential when assessing the financial and economic condition of a firm, with the main aim being to compare two alternatives. The first one regards the liquidation of a company, through a judicial procedure (such as bankruptcy) aiming at selling all the assets and paying all the debts of the distressed company, which will disappear from the market. The second alternative concerns the rescue of a firm, through a turnaround process: in this case, the firm is experiencing a decline, and a timely intervention would mean reducing losses and restoring the company, making the creation of new value possible. This would mean emphasising more the dynamic nature of the failure process, at the same time enhancing the connection with early warning systems: instead of focusing merely on predicting bankruptcy, implementing one or several models and selecting those having a higher predictive ability, it would be highly desirable to take into account all the steps preceding the final distress.
Forecasting model ratios: discussion of main findings
The findings from this review show that the most adopted factor is Net Income to Total Assets, followed by Current Ratio and Working Capital to Total Assets. However, it can be observed that increasing the number of factors to be included in a model does not necessarily improve its predictive accuracy. Moreover, a ratio can have a high predictive ability one year before bankruptcy and a lower one in the previous years. This means that other factors should be taken into account. One of the elements deserving attention is the legal requirements (namely the bankruptcy or safeguard procedures provided by the insolvency law), which can strongly affect the formal declaration of bankruptcy as well as the characteristics of timely intervention. Furthermore, management skill can also influence the financial distress (Platt and Platt, 2008). More generally, apart from the factors showing a high predictive ability, financial distress and/or bankruptcy can be defined and influenced by the context where a firm is managed. Following this line of thought, another important factor to consider could relate to the differences in the accounting rules to be adopted, which can have a relevant influence on the way of translating economic transactions into accounting numbers. Accordingly, taking into account different contexts and comparing them could be of great interest to both researchers and practitioners. To select countries to be examined, future studies could relate to international accounting classifications (Alexander and Nobes, 2002), which group countries according to different factors, such as:
(1) Commercially driven vs. government-driven and tax-dominated corporate financial reporting;
(2) Large vs. small stock exchange;
(3) Shareholder vs. creditor orientation;
(4) Strong vs. weak profession;
(5) Professional-driven vs. government-driven accounting standards; and
(6) Common law vs. civil law system.
Although several characteristics are evolving, due to the international accounting harmonisation at a global (and especially European) level, reducing the accounting differences between countries, these criteria could be useful to identify different clusters because of the persistence of some dissimilarities. Finally, bearing in mind the contradictory results concerning the role of corporate governance variables, further studies are recommended to investigate these issues, assessing their role in both listed and non-listed firms. In this way, it could be possible to implement models and use ratios which are not based on stock market data, as frequently occurred where listed firms are investigated. Indeed, as Tobback et al. (2017) underline, data regarding firms’ managers and directors are highly desirable while investigating small- and medium-size entities. Finally, it is worth observing that the vast majority of previous studies have used a unique prediction rule, with the consequence being that they could not take into account the diversity of the different components upon which the performance model has been built. This would imply that such a model could not capture the complexity governing bankruptcy; accordingly, as suggested by du Jardin (2016), combinations of classifiers are desirable since they tend to be more accurate than those based on a single prediction rule. Therefore, further research could investigate these issues.
Early warning systems and safeguard procedures: discussion of main findings
The main point deserving attention is that several countries are paying attention to early warning systems, considering them as an alternative to bankruptcy. This article points out that in the current context there is a need to investigate and propose tools able to prevent financial distress rather than merely predict it. Following Volkov et al. (2017), it is worth reaffirming that financial distress is a dynamic process; accordingly, the faster a remedy is appointed, the higher the probability of solving troubling situations, avoiding the distress and safeguarding the going concern of the firm experiencing financial difficulties. However, only a few studies (Amendola et al., 2017) have emphasised this aspect.To make this central point clearer, we would argue that selecting an appropriate tool to prevent failure would mean taking into account several criteria, essentially based on the analysis of strengths and weaknesses, as Alaka et al. (2018) suggest. Table 1 illustrates the recovery rate, its time and costs and the strength of insolvency framework index regarding several European countries and the US. The strength of insolvency framework index is calculated (only in 2016) as the sum of the scores concerning four sub-indices: the commencement of proceedings index, the management of debtor’s assets index, the reorganisation proceedings index and the creditor participation index.
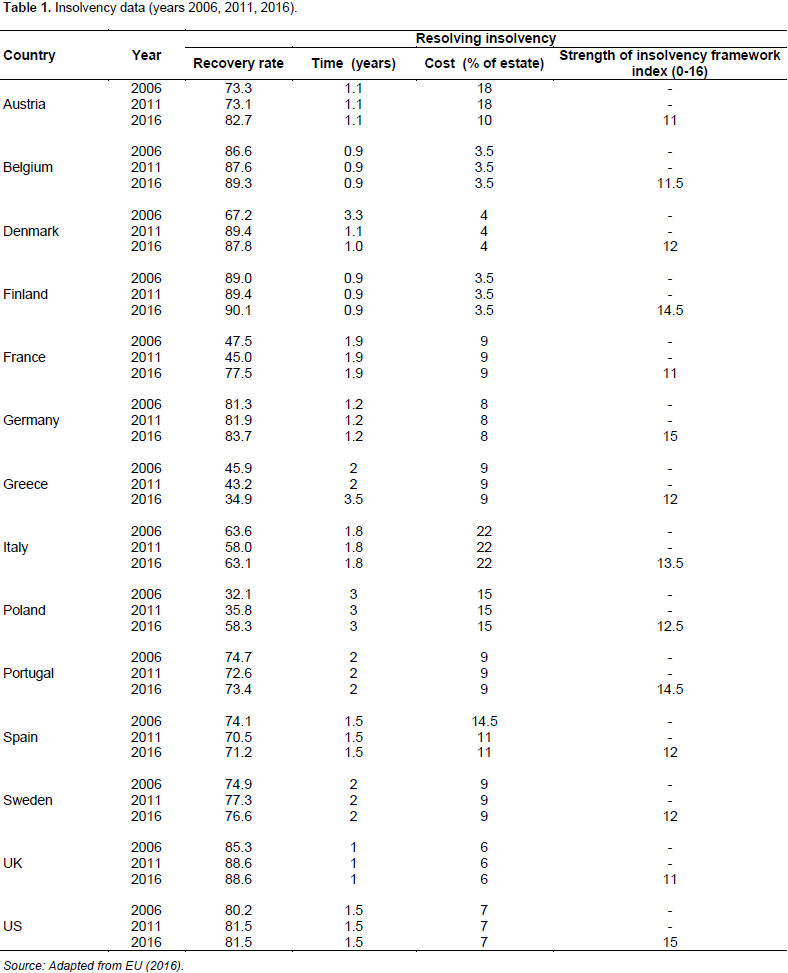
According to the World Bank, this index ranges from 0 to 16: the higher the value of the index, the better the insolvency legislation is designed for rehabilitating viable firms and liquidating nonviable ones. As can be easily observed, several differences exist between countries, especially regarding the recovery rate (which ranges from 32.1% in Poland to 90.1% in Finland) and the strength of insolvency framework index. Focusing on the case of France previously examined, where early warning systems have a long tradition, we can observe that the recovery rate was quite low in 2001 and 2006; however, bearing in mind that important reforms concerning early warning procedures were implemented in 2014, the recovery rate of 2016 increased a lot. Accordingly, these procedures testify how relevant having a proactive approach towards firms experiencing financial difficulties could be. Indeed, managers are required to develop a culture of default risk, since experiencing financial problems should be considered as a ‘physiological’ condition during the life-cycle of a firm. In other words, managers are required to manage the risk of crisis rather than the state of crisis of their company. This means developing a positive approach toward this risk, prearranging the strategic and operational paths to follow to solve troubling conditions. This would imply also adopting early warning systems through which triggers events can be promptly perceived and timely interventions can be carried out. From a theoretical perspective, this would call for further research based on an interdisciplinary approach, merging law, economics, accounting and finance. However, the effectiveness of these procedures should not be taken for granted. Accordingly, we call for further research, to provide models through which the evolving condition of a firm experiencing financial difficulties can be forecast. Furthermore, additional efforts are required to assess the efficacy of timely intervention procedures, also comparing different countries, to take into account the effects of the legislation as well as other factors (such as those illustrated in the previous section) that could play a role in guaranteeing the rescue of the firm or accelerating its liquidation, reducing the (indirect) costs of bankruptcy (Bisogno and De Luca, 2014).