ABSTRACT
Organizational readiness for change is considered a critical precursor to the successful implementation of complex changes. Indeed, some suggest that failure to establish sufficient readiness accounts for one-half of all unsuccessful, large-scale organizational change efforts. The African economy and the world at large are at brink of economic depression as result of devastating effect of COVID-19. However, it is equally important to determine the readiness of countries to absorb the economic shock of the pandemic. Using the model by Battese and Coelli (1995), the translog production frontier was adopted to estimate technical efficiency of the financial sector of the continent. The 24 countries selected in Africa were based on the availability of data to cover our variables of interest for period 2000 to 2018. The findings were that financial sector in the continent have performed above average (72%) over the period of study and hence they are slightly ready to support ailing economy. Also lower middle income countries are relatively going to have more problems with pandemic. However, the probability of the continent not plunging into economic depression with the support of the financial sector is 0.42 which is not encouraging. It is recommended that policies to address interest rate margin, liquidity and market concentration should be managed properly to improve technical efficiency of the financial sectors on the continent.
Key words: Africa, COVID-19, financial sector, technical efficiency, translog production frontier.
Readiness is the state of preparedness of organizations to meet a situation and carry out a planned sequence of actions. Organizational readiness for change is considered a critical precursor to the successful implementation of complex changes. Indeed, some suggest that failure to establish sufficient readiness accounts for one-half of all unsuccessful, large-scale organizational change efforts. Drawing on Lewin’s three-stage model of change, change management experts have prescribed various strategies to create readiness by 'unfreezing' existing mindsets and creating motivation for change. These strategies include highlighting the discrepancy between current and desired performance levels, fomenting dissatisfaction with the status quo, creating an appealing vision of a future state of affairs, and fostering confidence that this future state can be achieved.
Future economic environment is very difficult to predict hence it is contingent on every economy to efficiently utilize its resources. For this reason countries or firms always have to strive to make the best out of the available resources so as to be able to weather the storm in future. A country or firm that has been performing in the past always stands the chance to support their economy when the need arises. During economic depression, countries that over the years have been doing well are always in better position to give stimulus packages to support livelihood and also to bring the economy back on track on time. For this reason the readiness of an economy to fight economic depression depends on the efficiency of the economy over the years. Efficient economies are able to save enough to cover unforeseen contingencies.
In the 80’s most emerging economies through directives from International Monetary Fund were taken through different forms of Financial Sector Adjustment Program (FINSAP). The objective was to address the institutional deficiencies of the financial system, and developing money and capital markets (Brownbridge and Gockel, 1996). According to Bikker (2010) banks contribute to investments, employment creation and the process of economic growth and development. They are the corner stone of an economy of a given nation (Omankhanlen, 2012; Athanasoglou et al., 2006; Sergeant, 2001). However, the continent Africa is always referred to as low income or developing countries. Since the mid-1990s, this continent have seen steady economic growth but struggled to sustain that development. United Nations Economic Commission for Africa (UNECA) is predicting a fall in the growth rate from 3.2 to 1.8% for emerging due to the novel virus COVID-19 (IMF, 2020).. The existence of a solid and efficient financial system is a crucial condition for a sustainable economic growth for the continent Africa.
The pandemic has posed a major disruption to economic activity across the world. It is estimated by World Bank that economic growth in sub-Saharan Africa will decline from 2.4% in 2019 to -2.1 to -5.1% in 2020, the first recession in the region in 25 years. Even though events on the coronavirus pandemic are still unfolding, a preliminary analysis of the impact of the coronavirus menace on the real sector for most countries if not all shows that the 2020 projected real GDP growth rate could decline significantly (AUC, 2020).
It is also stated that the effect will also depend on how countries India, China, the USA will perform or deal with the crisis. However, it is equally important to determine the readiness of countries to absorb the economic shock of the pandemic.
According to WHO as at 4th August, the situation by WHO regions were Americas 9,741,727, Europe 3,425,017, South-East Asia 2,242,656, Eastern Mediterranean 1,574,551, Africa 825,272 and Western Pacific 332,754. This is an indicative that relatively African is not had hit by the virus but it is a novel virus. On 17 April, the World Health Organization (WHO) warned that Africa could be the next epicentre of the Coronavirus. It worth noting that South Africa is fifth country in the world with the highest cases of COVID-19. Bloomberg estimates that in a pandemic situation, Covid-19 could cost the world economy $2.7 trillion Equivalent to UK’s economy. This implies that if developing countries and emerging economics will be significantly impacted without support. However, the global financial crisis in 2008 also did not spear emerging economies. Every single person on the continent must exercise the highest level of self-discipline which is evidently clear in the economic numbers. The greater the level of self-discipline and civic responsibility the greater chances of avoiding mass job losses and its concomitant hardships.
According to African for Center Economic Transformation (ACET), the continent needs growth with diversification, export competitiveness, productivity increases, technological upgrading and improve human well-being (DEPTH). To achieve DEPTH requires mobilization of savings and channeling of financial resources to seekers of loan (Gulde et al., 2006). In this era of COVID-19 the efficiency of the financial sector becomes more pronounced when it comes to economic development of the continent. The financial sector is crucial to the economies of various countries, and banks remain a core of the sector, especially in emerging economies where the capital market is not strong enough (Matthew and Laryea, 2012). Delis and Papanikolaou (2009) indicated that an efficient financial sector will be better able to withstand negative shocks and contribute to the stability of the financial system. Thus, it is crucial to analyze the efficiency performance of banks and the factors behind their efficiency performance.
This article seeks to determine the readiness of financial sector on the continent Africa to surpass the effect of COVID-19. To be precise on how market concentration, market liquidity and net interest margin account for efficiency of the sector. The main objective of our study is to identify the determinants of the technical efficiency. This is because what happens in the financial sector has impact on the productive capacity of the nation’s economy. Relieves and grants from World Bank, IMF, governments and other organizations and associations to members and various economic sectors will be implemented through the financial sector of various countries and hence the need for the sector to be relatively efficient in their activities with their stakeholders to get value for money.
Economic impact of COVID-19
The COVID-19 pandemic thus poses important risks, not only for people’s health but also for their economic wellbeing. Already disadvantaged groups will suffer disproportionately from the adverse effects. Low-income earners, especially informal workers, who earn a living on a day-to-day basis and have limited or no access to healthcare or social safety nets, are severely hit.
Informal sector businesses are like any other businesses. With COVID-19 bringing transportation and market demand to a halt, informal sector businesses, such as drinking and chop bars, small retail shops, hairdressers, and taxi drivers will see a reduction in customers because of the pandemic and they form the majority Africa.
Africa’s GDP growth at 2019 was 3.6%. This is not sufficient to accelerate economic and social progress and reduce poverty. Since 2000, Africa’s GDP growth has largely been driven by domestic demand (69% of the total), rather than increases in productivity. Africa’s labour productivity as a percentage of the US level stagnated between 2000 and 2018, and the Africa-to-Asia labour productivity ratio has decreased from 67% in 2000 to 50% today (AUC, 2020; OECD, 2020). Global markets account for 88% of Africa’s exports, mostly in oil, mineral resources and agricultural commodities.
At the onset of the crisis, prospects differed across economies. Some were displaying high growth-rates, in excess of 7.5% (Rwanda, Côte d’Ivoire and Ethiopia), but Africa’s largest economies had slowed down. In Nigeria (GDP growth of 2.3%), the non-oil sector has been sluggish, in Angola (-0.3%) the oil sector remained weak, while in South Africa (0.9%) low investment sentiment weighed on economic activity.
In response to the pandemic, most countries in the continent have come up with relief packages to support businesses and individuals. Côte d’Ivoire, Ethiopia, Nigeria, Senegal and Uganda are spending CFAF 96 billion, USD 1.64 billion, N 500 billion, CFAF 1 000 billion and USD 7 million respectively to support their economy. In the case of South Africa the government is assisting companies and workers facing distress through the Unemployment Insurance Fund (UIF) and special programmes from the Industrial Development Corporation. A new 6-month COVID-19 grant is also created to cover unemployed workers that do not receive grants or UIF benefits and the number of food parcels for distribution was increased. Funds are available to assist SMEs under stress.
Liquidity and technical efficiency
Liquidity plays a central role in its successfully functioning as a profitable firm. Liquidity has major importance to both shareholders and potential investors. The goal of liquidity management should be to enable a firm to maximize profits of its operations while meeting both short term debt and upcoming operational expenses, that is, to preserve liquidity (Panigrahi, 2014). Excessive investments in liquidity may lead managers to make investments towards maximizing their own utility, thus to the detriment of profitability (Fama and Jensen, 1983). Ensuring adequate liquidity is essential in banking operations because of the financial intermediation role of banks. This intermediation role of banks exposes them to an inherent liquidity risk, which can have dire consequences on the banks’ earnings and solvency (Berger and Bouwman, 2009). The ability to meeting obligations as and when they fall due is an important determinant of bank efficiency. Literature on banking failures has provided sufficient evidence showing that undue liquidity shortage has a predominant impact on the efficiency and solvency of banks. This determinant, called liquidity is measured by the ratio of loans to assets. The lower the variable (ratio) the higher the bank’s liquidity and the vice versa. The article expects liquidity to have a positive impact on the efficiency of banks (Nassreddine et al., 2013). Tochkov and Nenovsky (2009) also revealed that liquidity has positive effect on financial sector efficiency.
Market concentration and technical efficiency
Market concentration is an important indicator to define the bank sector stability in financial terms. Shim (2019) examines the effect of loan portfolio, market concentration on the bank’s financial stability. He has investigated the effect of loan diversification and concentration factor in banking market. Findings of the study explains that factor of market concentration is negatively associated to financial strength of the banks. Additionally, those banking firms which are operating in more diversified situation and highly concentrated markets are more stable, comparatively to those which are dealing under less concentrated market. Some of the other studies have provided their limited contribution in the literature for market concentration and financial stability in banking sector (Ghosh, et al., 2018; Syadullah, 2018; Cheng et al., 2018; Omodero and Ogbonnaya, 2018; Hallunovi and Berdo, 2018; Elkhayat and ElBannan, 2018). The article proxy market concentration with HHI because it is better than market shares of the big three (CR3) banks or five banks (CR5). This is a good proxy because it reflects the degree of market share inequality across the different banks in the banking industry (Schumann, 2011). This article expected that highly concentrated markets will lower the cost of collusion and foster tacit and/or explicit collusion on the part of banks; hence, all banks in the market earn monopoly rents. The range of this variable is within the ranges from zero to one, where large number of banks, each with a small share, produces an HHI close to zero, while a single monopolist bank with a 100% share produces an HHI of one. Koutsomanoli-Filippaki et al. (2009) and Brissimis et al. (2008) findings also suggest strong links of competition and concentration with bank efficiency while credit risk have negative effect on efficiency.
Interest margin and technical efficiency
Seelanatha (2012) evaluated the drivers of Technical Efficiency with different approaches and the conclusion was that gross interest margin has positive impact of technical efficiency. However, Řepková (2015) evaluated the banking efficiency determinants in the Czech Banking Sector over the period 2001 to 2012 reveal that interest rate have negative impact of technical efficiency. Also, Shanmugam and Das (2006) reveal that technical efficiency of raising interest margin is varied widely across sample banks and is time-invariant. Even though several reform measures have been introduced since 1992, they have not so far helped the banks in raising their interest margin.
Our sample covers a balanced panel dataset of 486 observations over the period 2000 to 2018 with 24 groups. The groups are the financial sector for 24 African countries. The selection of these countries was based on the availability of data. The data on the financial sector of these countries were collect from Global Findex Database 2018 and the unit of analysis is the financial sector.
Literature for estimating bank efficiency is Stochastic Frontier Approach (SFA), Distribution Free Approach (DFA) and Thick Frontier Approach (TFA). The non-parametric methods that have been employed in literature for estimating bank efficiency are Data Envelopment Analysis (DEA) and Free Disposable Hull (FDH). The SFA and DEA are the most commonly used parametric and non-parametric methods, respectively. The SFA approach is a stochastic technique which integrates the random errors but also requires the predefinition of the functional form (Eisenbeis et al., 1999). This article uses the stochastic frontier efficiency estimates since it present more precise stock price behavior and adopts the standard translog functional model for multi products in estimating efficiency. This is specified as follows:
Data presentation and analysis
To analyze the readiness of African countries to give relief support and manage the economy our sample covers a balanced panel dataset of 486 observations over the period 2000 to 2018 with 24 groups. The countries selected can be categorized into low income country (12), low middle income country (8) and then upper middle income country (4). The database was extracted from global Findex database 2018. Adopting Sealey and Lindley (1977), the article considers financial sector as intermediary that used inputs like deposit and overhead cost to generate assets. Through the efficiency scores, the article will identify the difference between countries as well economic blocks over the period 2000 to 2018.
Table 1 shows all the variables predicted to influence efficiency in the model presented. Each variable have mean, standard deviation and min-max. The standard deviations are given in three categories: overall, between and within. The between variation implies the variation of the same variable over time. On the other hand, within variation refers to the variable among the different countries in different time periods. The mean of interest margin (IM) variable is 7.085, the minimum is 1.162 and maximum is 28.982. The overall variation is 3.472, with between variation of 2.77 and within variation of 2.15 which implies between variations in relation to interest margin dominates the variations in interest margin. In the case of the variables concentration and liquidity between variations dominated the variations in the data as shown in Table 1.
Figure 1 shows the trend net interest margin, bank concentration, liquid liability and technical efficiency over the period 2000 to 2020.
On the average, net interest margin and bank concentration are all on the downward trend which is good for the continent. Also, wealth noting is the trend for liquid liability and technical efficiency which on the average have been positive indicative of the fact that the financial sector in the continent are in the position to pay their liabilities as when they fall due and efficiently managing resources to get the returns. However, with technical efficiency, Figure 1D shows that most of the countries selected have their technical efficiency dipping at around 2016 onwards even though the average for period is on the upward trend.
The trend of net interest margin, liquid liability and concentration converge with time is as shown in Figure 1E. This is indicative of the similarities in the sampled countries and also readiness of the sector to support economic growth on the continent.
The version of the stochastic model used for this article is the true fixed effect. The Wald Chi2 of the whole model was 21.3 with probability Chi2 of 0.000 with a log likelihood of -298.3405. This is indicative of the fact that the model is well fitted.
With the frontier regression model all the interaction variables and the squared variables were dropped because of multicollinearity problem with the exception of the product of technology and other variables. The variable Credit (C) and Deposit (D) and overhead cost were all significant at different significant levels in explaining stochastic production frontier for determining the efficiency scores. The trend variable (T) which proxy for technology was positive and significant at 1% shown in Table 2. The signs of the other variables conform to theory.
The second part of Table 2 is the regression of technical efficiency on concentration, liquidity and interest margin. The variables for the second regression start with prefix “mu”. The dependent variable in second regression is technical efficiency derived from the first regression. Interest margin is significant at 10% has positive effect on technical efficiency and the coefficient is negative 0.019. Put differently the negative effect here means that interest margin have negative effect on technical inefficiency. This implies that as interest margin improves or increases it improves the technical efficiency of financial sector on the continent Africa.
In the case of market concentration is also significant at 5% and coefficient of 0.001 implies that improvement or increase in market concentration affects technical efficiency of financial sector on the continent. These also conform to theory.
To validate whether the stochastic model is relevant to the article conducts a number of diagnostic checks. From the regression output the article computes the ratio of variance of the technical inefficiency to the total variance of the error term gives a statistic that accounts for the variations in output accounted for by technical inefficiency and this statistic should range between zero and one. If the statistic is close to one (1) then it means that much of the variations in output are accounted for by technical inefficiency implying the stochastic model is the appropriate one. From the regression output for Table 2 the article have standard error of Usigma to be 0.2518312 with a variation of 0.063419 and Vsigma is 0.1247323 with a variance of 0.015558 giving a total variance of the model to be 0.078977.
So the proportion of variation to technical inefficiency to total variance gives us 0.803. Since this statistic is closer to one (1) it is an indicative that the stochastic model is relevant. In other words technical inefficiency accounts for about 80% of the variations in the model.
The article again conducts the likelihood ratio statistic recommended by Kumbhakar et al. (2015) which is in this case is 1370.376. To validate whether the stochastic frontier model is relevant, the article computes the statistic recommended by Kumbhakar, Wang and Homcastle and compare it with the critical values that are reported in Kodde and Palm (1986). From the unrestricted and restricted regressions the maximum likelihood ratios are -290.4436 and -505.95362, respectively and this gives the statistic 431.02. Comparing the statistic 431.02 with critical values of the mixed Chi-square distribution it is realized that at one degree of freedom under 2.5% significance level the statistic is greater than the critical value of 3.841; hence the article can reject the null hypothesis which says that the stochastic frontier model is not appropriate. In other words the article strongly rejects the null hypothesis of no technical efficiencies.
Since the stochastic model is relevant the article predicts technical efficiency from the model. The average technical efficiency for the period 2000 to 2018 is 0.718. Within variations dominate the variations in the technical efficiency. The minimum and maximum within variations are 0.0781 and 0.9798, respectively as depicted in Table 3. The average technical efficiency of 0.718 is an indicative that about 72% of emerging economies are well positioned to help economies to bounce back from the smother of COVID-19 as at 2018. In the case of the readiness of the continent the 72% gives a readiness of 42% to the consequences of COVID-19 to economic activities. This implies that policy makers will have work hard to improve net interest margin, liquid assets and market concentration of financial sector on the continent if the sector can really help economic activities to bounce back. In other words, the continent faces a probability of 42% to prevent economic depression.
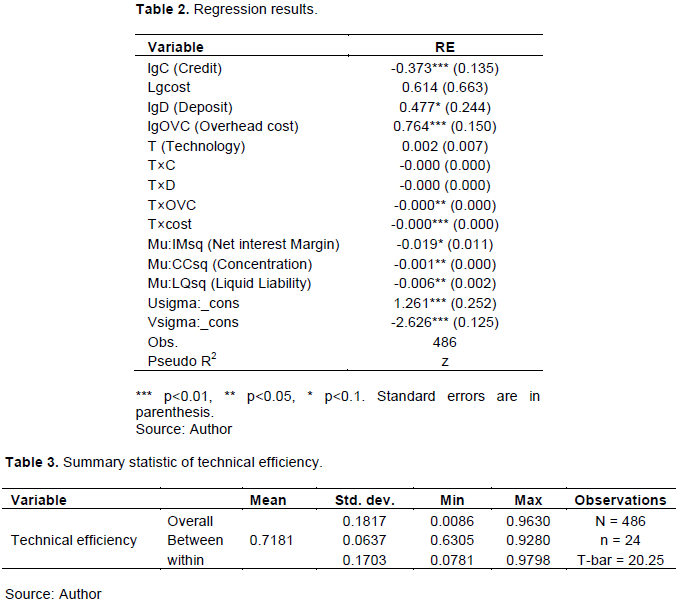
Figure 2 shows the trend for the predicted technical efficiency for the continent Africa sampled for this article. From the trend it can be deduced that most of the countries sampled had technical efficiency below 0.5 and after 2006 most countries had technical efficiency above 0.5. The bar graph of Figure 3 confirms that for the period under study, all countries sampled on the African continent have technical efficiency greater than 0.5 which is indicative that the financial sectors in these countries were all technically efficient on the average.
Appendix B depicts the rankings of the countries sampled in terms of technical efficiency. The first six (6) countries are Mauritius, South Africa, Botswana, Malawi, Madagascar and Kenya.
Countries on the continent that are likely to have challenges in the area of technical efficient in the financial sector are Zambia, Nigeria, Burkina Faso, Côte d'Ivoire, Cameroon and Gabon with Zambia being the worse country in terms of technical efficiency.
This article ranks South African financial sector among the best performing on the continent. However, according to S&P Global Ratings South African banks are unlikely to boost lending in 2020 amid economic uncertainty. This is because real GDP growth is expected to average 1.6% in 2020-2021 and also the fact the credit loses is expected to increase by 1.2% in 2020 hence banks extend credit slowly. For this reason, ratings for all top-tier banks in South Africa were assigned negative outlooks. This is an indication that as leaders in the continent fight COVID-19 pragmatic solutions should be also tailored to address the financial sector to ensure the necessary support for the economy. The continuous efficient operation of a financial sector in an economy is necessary if an economy is saving enough the unforeseen contingency. Botswana on the other hand was ranked 3rd on the continent. This is not surprising since there has been series of projects intended to position the country to serve international financial sector. In relation to readiness of Botswana to support the shock of the pandemic our results put the country at 54% ready.
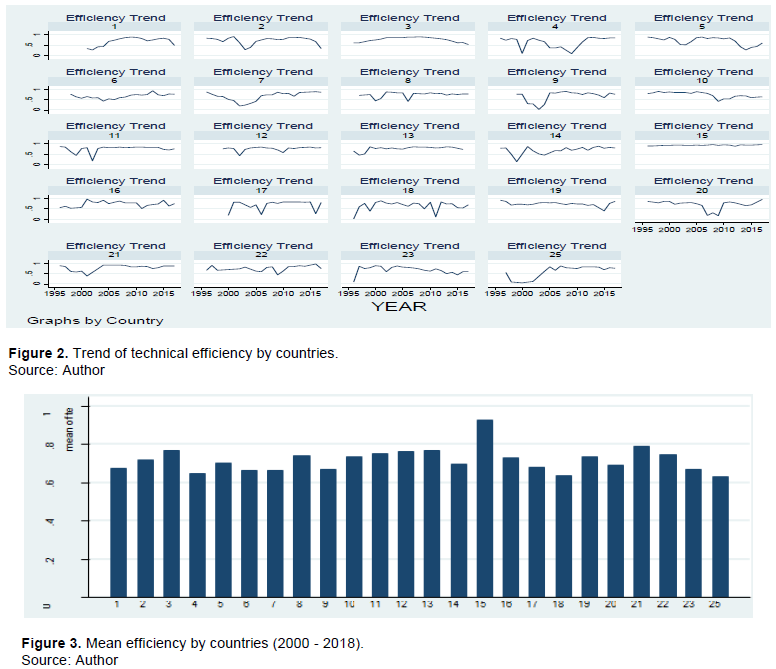
In the work of Antwi-Asare and Addison (2000) they concluded that Ghana's banks have performed poorly in the past, offering but a narrow range and inefficient delivery of financial instruments, being strongly biased in their reach to the urban formal sector and, in particular, to the holding of low-risk government paper, and being largely non-competitive in structure. These results are indicative that banks in Ghana pass on their inefficiencies to their customers by raising their lending rates and lowering their deposit rates.
Technical efficiency of financial sector by income grouping
Taking into consideration World Bank (WB) income groupings of countries, this article has considered low income, lower middle income and upper middle income countries. The mean technical efficiency for upper middle income (0.7934) and low income (0.7113) were as expected according to theory. However, mean technical efficiency for lower middle income of (0.6898) is not expected as shown in Appendix A. The implication here is that upper middle income and low income countries are more technically efficient than lower middle income countries. That means they are more resilient relatively when it comes to withstanding the economic shock as a result of COVID-19. The variations in technical efficiency for various income groups are more prominent in between countries for each group then within countries. However, Figure 4 shows that technical efficiency over the period improved significantly for upper income countries in 2005 and since then financial sectors have been doing very well.
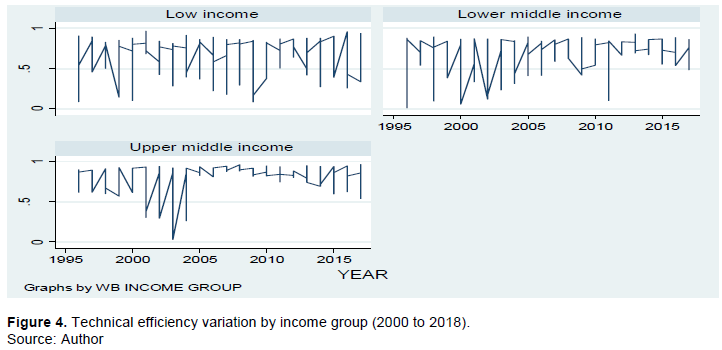
This is also the case for lower middle income countries. The difference is that financial sectors were really not doing very welling from 2000 to 2005. Afterwards there has been some appreciable improvement in the sector which gives the net effect performance in terms of technical efficiency behind the other groupings of low income and upper middle income countries. The implication is that lower middle income economies are more at risk to COVID-19 than low income and upper middle income countries on the African continent.
Organizational readiness for change is considered a critical precursor to the successful implementation of complex changes. Failure to establish sufficient readiness accounts for one-half of all unsuccessful, large-scale organizational change efforts. The readiness of an economy to withstand the economic depression of COVID-19 depends on how efficient that economy has been performed over the years. An economy that has been operating on the efficient frontier is likely to be in a better position to give up some relief packages for social interventions and also to oil the economic engine of the country to bounce back. Countries have to shut down their economic activities in attempt to fight this pandemic. Countries that have been efficient over the years are likely to manage post COVID-19 crisis better. This is because to efficiently operate for some years they are likely to have more reserves to rely on in times of need. In the light of this the financial sector of Africa is about 72% ready to support the economy due to the efficiency level of the financial sector of the continent. In other words, there is the probability of 0.42 that economy of the African continent will not plunge into economic depression. This is far below average and by implication Africa should be bracing itself for severe economic shock if proper effective relief programs are not put in place. It is recommended that policies to address interest rate margin, liquidity and market concentration should be managed properly to improve technical efficiency of the financial sectors on the continent. In terms of income groupings on the continent the lower middle income countries are relatively challenged by technical efficiency to impact positively on economic development. In the light of this it is proposed as IMF and other international monetary fund plans to support economies, the continent Africa should be considered.
The authors have not declared any conflict of interests.
REFERENCES
Antwi-Asare TO, Addison EKY (2000) Financial Sector Reforms and Bank Performance in Ghana. University of Ghana, Overseas Development Institute Portland House, Stag Place.
|
|
Athanasoglou P, Delis MD, Staikouras CK (2006). Determinants of Bank Profitability in the South Eastern European Region. Bank of Greece WP 47, Economic Research Department, Athens.
|
|
|
African Union Commission (AUC) (2020). Impact of the Coronavirus (COVID 19) on the African Economy, African Union Commission, Addis-Ababa.
|
|
|
Battese GE, Coelli TJ (1995). A model for technical inefficiency effects in a stochastic Frontier production function for panel data. Empirical Economics 20:325-332.
Crossref
|
|
|
Berger AN, Bouwman CHS (2009). Bank liquidity creation. The Review of Financial Studies 22(9):3779-3837.
Crossref
|
|
|
Bikker JA (2010). Measuring performance of banks: an assessment. Journal of Applied Business and Economics 11(4):141-159.
|
|
|
Brissimis SN, Delis MD, Papanikolaou NI (2008). Exploring the Nexus between Banking Sector Reform and Performance: Evidence from Newly Acceded EU Countries. Bank of Greece Euro System Working Paper 73, Athens.
Crossref
|
|
|
Brownbridge M, Gockel AF (1996). The Impact of Financial Sector Policies on Banking in Ghana. Institute of Development Studies WP 38.
|
|
|
Cheng CP, Phung MT, Hsiao CL, Shen DB, Chen BS (2018). Impact of Operational Risk Toward the Efficiency of Banking-Evidence from Taiwans Banking Industry. Asian Economic and Financial Review 8(6):815-831.
Crossref
|
|
|
Delis MD, Papanikolaou NI (2009). Determinants of bank efficiency: Evidence from a semi parametric methodology. Managerial Finance, 35:260-275.
Crossref
|
|
|
Eisenbeis R, Ferrier GD, Kwan SH (1999). The informativeness of stochastic frontier and programming frontier efficiency scores: Cost efficiency and other measures of bank holding company performance.
Crossref
|
|
|
Elkhayat N, ElBannan MA (2018). State Divestitures and Bank Performance: Empirical Evidence from the Middle East and North Africa Region. Asian Economic and Financial Review 8(2):145-171.
Crossref
|
|
|
Fama E, Jensen M (1983).Agency problems and residual claims. Journal of Law and Economics 26(2):327-349.
Crossref
|
|
|
Ghosh PK, Khatun M, Tarafdar P (2018). Bankruptcy Via Earning Volatility: Does it Integrate in Financial Institutions?. Asian Economic and Financial Review 8(1):52-62.
Crossref
|
|
|
Gulde AM, Pattillo C, Christensen J, Carey K, Wagh S (2006). 'Financial Sectors: Issues, Challenges and Reform Strategies. Technical Chapter in Regional Economic Outlook: Sub-Saharan Africa. Washington: International Monetary Fund, May.
|
|
|
Hallunovi A, Berdo M (2018). The Relationship between Risk Management and Profitability of Commercial Banks in Albania. Asian Themes in Social Sciences Research 1(2):44-49.
Crossref
|
|
|
International Monetary Fund (IMF) (2020). Policy Response to COVID-19.
View (accessed on 5th August 2020).
|
|
|
Kodde AD, Palm F (1986), Wald Criteria for Jointly Testing Equality and Inequality Restrictions, Econometrica 54(5):1243-1248.
Crossref
|
|
|
Koutsomanoli-Filippaki A, Margaritis D, Staikouras C (2009). Efficiency and productivity growth in the banking industry of Central and Eastern Europe.Journal of Banking and Finance 33:557-567.
Crossref
|
|
|
Kumbhakar S, Wang H, Horncastle AP (2015). A Practitioner's Guide to Stochastic Frontier Analysis Using Stata, Cambridge University Press.
Crossref
|
|
|
Matthew NG, Laryea AE (2012). A financial performance comparison of foreign vs local banks in Ghana. International Journal of Business and Social Science 3(21):82-87.
|
|
|
Nassreddine G, Fatma S, Anis J (2013). Determinants of banks performance: viewing test by cognitive mapping technique a case of Biat. International Review of Management and Business Research 2(1):20-36.
|
|
|
OECD (2020). COVID-19 and Africa: Socio-economic implications and policy responses.
View. (accessed on 5th August 2020)
|
|
|
Omankhanlen AE (2012). The role of banks in capital formation and economic growth: The case of Nigeria. Economy Transdisciplinarity Cognition 15:103-112.
Crossref
|
|
|
Omodero CO, Ogbonnaya AK (2018). Corporate Tax and Profitability of Deposit Money Banks in Nigeria. Journal of Accounting, Business and Finance Research 3(2):47-55.
Crossref
|
|
|
Panigrahi AK (2014).Relationship of working capital with liquidity, profitability and solvency: Acase study of ACC Limited. Asian Journal of Management Research 4(2):308-322.
Crossref
|
|
|
Řepková I (2015). Banking efficiency determinants in the Czech banking sector. Procedia Economics and Finance 23:191-196.
Crossref
|
|
|
Schumann L (2011). Measuring Market Concentration (online). Available:
View. Date accessed: 14 December 2014.
|
|
|
Sealey C, Lindley JT (1977). Inputs, outputs and a theory of production and cost at depository financial institution. Journal of Finance 32:1251-1266.
Crossref
|
|
|
Seelanatha SL (2012). Drivers of technical efficiency of Sri Lankan commercial banks". International Journal of Applied Economics 9:41-58.
|
|
|
Sergeant KA (2001). The role of commercial banks in financing growth and economic development in Trinidad and Tobago and the Caribbean: A perspective from the royal bank of Trinidad and Tobago. Belize: Central Bank of Belize.
|
|
|
Shanmugam KR, Das A (2006). Efficiency of Indian commercial banks during the reform period, Applied Financial Economics 14(9):681-686.
Crossref
|
|
|
Shim J (2019). Loan portfolio diversification, market structure and bank stability. Journal of Banking and Finance.
Crossref
|
|
|
Syadullah M (2018). ASEAN Banking Efficiency Review Facing Financial Services Liberalization: The Indonesian Perspective. Asian Development Policy Review 6(2):88-99.
Crossref
|
|
|
Tochkov K, Nenovsky N (2009). Efficiency of commercial banks in Bulgaria in the wake of EU accession. Bulgarian National Bank Discussion paper no DP/75/2009.
Crossref
|
|