Bank credit availability in the agricultural sector empowers farmers to adopt modern technologies and inputs that are vital for breaking poverty in developing economies like Zimbabwe. This study sought to establish the determinants of credit demand among farmers in Hurungwe District of Mashonaland West Province in Zimbabwe. A questionnaire survey was conducted on a sample of 354 farmers selected by stratified random sampling. The Direct Elicitation Approach was applied to comprehend the credit demand constraints faced by farmers using frequency statistics. Logistic Regression Analysis and Thematic Analysis were also used for analysing data. Farmers in Hurungwe District face price (95%), risk (79%) and transaction cost (58%) constraints. Interest rates, collateral and fear of debt have a negative and significant (p<0.05) effect on credit demand. Loan processing time emerged as another key determinant of credit demand among farmers. Policy should curb hyperinflation to ensure the affordability of loans and production inputs by farmers. Interest rate ceilings must also be restored, and financial markets literacy campaigns intensified to shield farmers from predatory lenders. Banks are challenged to improve communication with farmers, swiftly address their needs and relax collateral demands to enhance credit demand among farmers. Investments in irrigation and other weather resilience technologies should be prioritized to enhance agricultural sector performance and reduce credit uptake fears among farmers.
Agriculture supports majority of the population and industries in Zimbabwe (Ministry of Agriculture Zimbabwe, 2017). However, financial investments in the sector are not being prioritized as evidenced by low agricultural loan books in most commercial banks (Vitoria et al., 2012). Despite being an agro-based economy, agricultural credit comprised only 16.36% of the loans advanced by commercial banks in Zimbabwe in 2015 (Reserve Bank of Zimbabwe (RBZ), 2016). Regardless of improving tremendously to 31.69% by December 2019, the banks’ average agricultural loan portfolios are yet to surpass the pre-land reform maximum of 91.3% achieved in the year 1999 (RBZ, 2019). Consequently, the demand for bank credit in the agricultural sector remains unmet in Zimbabwe (Vitoria et al., 2012). This limited access to finance by farmers in Zimbabwe is cited as a major bottleneck to agricultural performance (RBZ, 2016). Besides, some studies have generally observed that a limited number of farmers, especially in the smallholder farming sector use credit, a financial resource whose deficiency constrains the farmers’ capacity to invest in inputs and technologies that are essential for enhancing production, income and livelihoods (Chitungo and Munongo, 2015; International Finance Corporation, 2014). Various studies have proposed that the credit constraints faced by households or individuals can be understood better if focus is not only placed on why they fail to access loans from banking institutions, but also on why they are not seeking or demanding any bank credit in the first place (Ali et al., 2014; Zeller, 1994). Chitungo and Munongo (2015)also highlighted that conventional methods of estimating demand for credit have only utilized information sourced from farmers who have accessed credit before, thereby neglecting crucial information from farmers who have not borrowed. This also makes it impossible to account for the farmers’ initial decision on whether or not to borrow.
According to the Iowa State University (2019), demand is a price and quantity relationship, which expresses the quantity of a product demanded at various price levels. In this study, credit demand is perceived as the farmers’ willingness to apply for bank credit from formal financial institutions at a given price, which is the interest rate. This study focused more on the taste/willingness/desire aspect of credit demand. Given the poor nature of most farmers in Zimbabwe, a focus on effective demand or the ability by farmers to afford the interest rates charged by banks would have segregated against farmers who had the desire to apply for bank credit, but could not afford the interest rates charged. According to Diagne et al. (2000), participation in credit programs is something that households or individuals choose to do, and was conceptualized by Zeller (1994)as a Sequential Decision Process (SDP). In the first stage of this SDP, the household or its member makes a decision to either apply or not apply for bank credit. In the second stage, the banker decides to supply or withhold the requested credit to the farmer who made credit application. The Direct Elicitation Approach (DEA) also embraces the SDP model’s quest to understand household/individual credit constraints from both the demand and supply side. Rooted in works by Boucher and Guirkinger (2005)in Peruvian agriculture, the DEA proposes that directly asking households on the reasons why they do not participate in credit markets can give a clearer picture of the constraints that they face. Therefore, according to the DEA, farmers can be unconstrained (no need for credit because they have adequate resources); price constrain (interest rates too high or lack collateral); transaction cost constrain (do not know where to apply, no bank account, lack of supplier etc.); or risk constrain (fear of losing collateral, fear of being rejected, do not like to be indebted). The approach mostly focuses on lender attributes as determinants of credit demand within households.
One of the variables recognized as a determinant of credit demand is the interest rate. Interest rate is defined by Faure (2014)as the reward paid by a borrower/ debtor to a lender/creditor for the use of money for a specified period. It is also widely referred to as the price of money. Collateral is another DEA variable, which is defined as the asset or assets pledged to secure a loan (Baiden, 2012). Another variable in the DEA is distance, which is simply the distance between the lender and the borrower. Awareness is the extent to which the borrower knows about the credit products offered by the lender, whilst fear of debt captures the farmer’s attitude towards borrowing, which may be partly attributed to the lender’s behaviour (for example, loan processing time and service quality), lending conditions (like collateral requirements) and characteristics (like location) . This study is mostly interested in understanding these local supply side attributes of banks that act as deterrents to credit demand among farmers, which justifies the adoption of the DEA. A few studies in Zimbabwe have undergone the determinants of credit demand among farmers in Zimbabwe. Chitungo and Munongo (2015)investigated the effect of various farmer and farm characteristics (age, marital status, education, household size, income, remittances, and crop type) on the farmers’ decision to apply for credit from informal lenders in the rural districts of Zaka, Chiredzi and Masvingo. Dube et al. (2015)only focused on the determinants of credit access in the formal sector among smallholder tobacco farmers in Makoni District. Therefore, no study to the researcher’s knowledge has applied the DEA to comprehend how lender/ supply side attributes affect Zimbabwean farmers’ credit demand for bank credit. Moreover, most of the available studies focused on credit demand determinants in the smallholder farming sector, somewhat neglecting the larger commercial farming sector. Most of the studies that investigated credit demand determinants also applied statistical techniques. Hence, there is a dearth of studies that have attempted to apply qualitative methodologies to comprehend credit demand constraints among farmers in general. These are the gaps that this study aims to fill, focusing on Model A1 smallholder and Model A2 commercial farmers in the Hurungwe District of Mashonaland West Province in Zimbabwe. Therefore, this study sought to establish the credit demand constraints faced by farmers in Hurungwe District, and the factors that influence their decision to seek formal bank credit.
The study adopted the pragmatism research philosophy, which embraces both quantitative and qualitative techniques (Madondo, 2015). Having adopted the pragmatism research philosophy as highlighted above, the study took up the embedded mixed methods research strategy in which qualitative techniques were used to support the main quantitative methods (Terrell, 2012). Quantitative and qualitative primary data were collected simultaneously and were subsequently mixed during the analysis phase, where the latter was used to explain and support the former in order to gain a broader perspective than could be gained from using the quantitative data in isolation (Almalki, 2016). Also known as triangulation (Dawson, 2002), this amalgamation of quantitative and qualitative methods in this study provided a broader and more complete vision of the low credit uptake problem in the Zimbabwean context (Almeida, 2018). The study was conducted in Hurungwe District, which is situated in the North-Western part of Zimbabwe in Mashonal and West Province (Hurungwe Rural District Council (HRDC), 2019). A cross-sectional questionnaire survey was carried out on a sample of 354 farmers, which was determined by the Raosoft sample size calculator. The questionnaire was structured and contained both closed and open-ended questions. Open ended questions allowed the study to collect some qualitative data which was used to complement the qualitative findings. Stratified random sampling was used to select farmers across the Model A1 and Model A2 farming sectors. The sample was thus made up of 281 Model A1 farmers and 73 Model A2 farmers.
Quantitative data analysis
This study mainly used quantitative techniques. Firstly, Ali et al. (2014)Direct Elicitation Approach (DEA) was adopted to define the credit demand constraints status of farmers in Hurungwe District, using frequency statistics from the Statistical Package for Social Sciences (SPSS). The study adopted Zeller (1994)Sequential Decision Process (SDP) model. The SDP model was adopted in various empirical studies. For example, Zapata (2017) study on credit decision and rationing rules in the informal credit markets of the Philippines adopted both stages of the model using the Probit (in Stage 1) and Ordinary Least Square (OLS) (in Stage 2) methods. In Zimbabwe, Chitungo and Munongo (2015) also applied Stage 1 of the SDP model in their study on the determinants of farmers’ decision to access credit in Masvingo, Zaka and Chiredzi rural districts, which were tested using the Probit method.
Therefore, this study adopted Stage 1 of the SDP model to comprehend the determinants of credit demand among farmers in Hurungwe District. It tested the statistical significance of some of Ali et al. (2014)DEA credit demand constraint factors (interest rates (IR), collateral (C), lender borrower distance (LBD), bank credit awareness (BCA) and fear of debt (FOD)) in influencing credit demand among farmers in Hurungwe District. Several studies adopted the DEA to comprehend households’ credit constrains, for example Ali et al. (2014); Boucher and Guirkinger (2005) and Mukasa et al. (2017)in Rwanda, Peru and Ethiopia respectively. The testing of DEA’s effect on farmers’ credit demand was done through Binary Logistic Regression Analysis in SPSS. Binary Logistic Regression Analysis was adopted because the dependent variable of credit demand is binary, taking zero or one values (credit demanded=1; no credit demand=0). Isaga (2018)also applied logistic regression to determine factors that affected smallholder farmers’ access to bank services in Tanzania. Its flexibility and ease of use also justified its adoption in this study (Isaga, 2018). The credit demand equation tested by Binary Logistic Regression Analysis in the study was modelled as follows:
CD = f (IR; CR; LBD; BCA; FOD)
Where:
CD= Credit Demand, the dependent variable. It is a dummy variable defined further as follows:
CD= 1 if credit is demanded;
CD= 0 if credit is not demanded.
The study’s independent variables were also defined as follows (Table 1). Findings from the quantitative data analysis were subsequently presented using tables and figures.
Qualitative data analysis
A few open-ended questions in the questionnaire allowed the researcher to access some qualitative data for the study to complement and support the main quantitative findings. NVivo was used to analyze the qualitative data through thematic analysis. Word Frequency Analysis was used to identify major themes from the farmers’ responses. Findings from the qualitative data analysis were presented through a word cloud in this study.
The findings from the study are presented in this section. The section initially presents and discusses the quantitative results before proceeding to present and discuss the qualitative findings.
Credit demand constraints status of farmers in Hurungwe District
Table 2 shows the Hurungwe District farmers’ credit application status, which captures their demand for credit. Only 6% of the farmers under study applied for bank credit within the last 12 months (Table 2). Therefore, the majority of the farmers (94%) never applied for any bank loans within the same period. Out of the 312 farmers who never sought bank credit within the last 12 months, the majority (89%) were in the smallholder Model A1 farming sector, whilst a few (11%) were in the commercial Model A2 sector. Among the 20 farmers who applied for bank credit, only one farmer belonged to the Model A1 farming sector. The majority of non-applicants were also males (65%), whilst women comprised a minority 35%. Among the 20 farmers who applied for bank credit, only 5 were women. The minimal participation by Model A1 smallholder farmers in formal credit markets may be attributed to the preference for highly collateralized and bigger Model A2 commercial farmers by most banks in Zimbabwe as confirmed by various studies (FACASI, 2015; Vitoria et al., 2012). Chitungo and Munongo (2015) and Tetteh (2011)also established that men demanded more credit than women because they owned larger plots and possessed egos that compelled them to fight for surplus production, which require increased financial investments, thereby increasing their demand for credit.
The majority (95%) of the farmers in Hurungwe District who never sought bank credit in the last twelve months were price constrained as they lamented that the interest rates charged by local banks were expensive and beyond their reach (Figure 1). The United Nations (2014) and Vitoria et al. (2012)confirmed that interest rates charged on loans in Zimbabwe are high, uncompetitive, prohibitive and only affordable to high income earners, a scenario that was attributed to the liquidity challenges faced by the entire financial sector. Some 87% of the farmers who never sought bank credit within the past year bemoaned that they lacked the immovable, titled collateral demanded by banks in Zimbabwe. A few (47%) farmers cited distance to the nearest bank as their barrier to seeking bank credit, whilst 40% claimed that they did not know where to apply for bank loans. Therefore, an average of 58% of farmers who never sought bank credit in Hurungwe District was transaction cost constrained (Figure 1). The dominance of collateral as a repellent of participation in credit markets by farmers in Zimbabwe was also observed by various studies, which ascribed it to the change in land tenure from freehold to usufruct rights after the Fast Track Land Reform Program (FTLRP) (Masiyandima et al., 2011; Ministry of Agriculture, 2013; Nyamutowa and Masunda, 2013; Richardson, 2005).
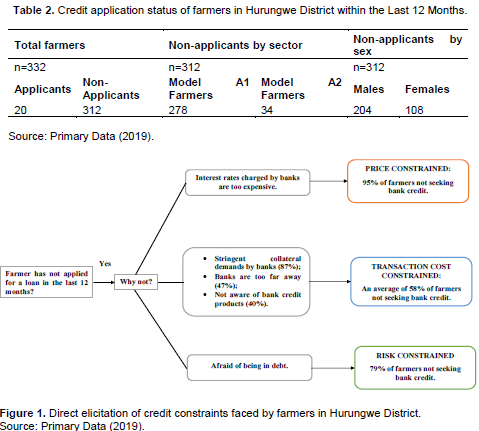
About 79% of the farmers who never sought bank credit in Hurungwe District were also risk constrained as they were afraid of being in debt (Figure 1). Most of the farmers highlighted that they were better-off using the little resources at their own disposal because they feared losing personal possessions if they defaulted, a situation which they felt was likely to happen because of the recurring droughts spells in the country and their high dependence on rain fed agriculture, due to lack of irrigation infrastructure. Several studies confirmed that extreme weather events like prolonged droughts are negatively affecting agricultural production in Zimbabwe and Africa at large, thereby affecting the farmers’ ability to forecast with certainty their expected yields unlike other entrepreneurs (Chakoma and Chummun, 2019; Maurer, 2014; Nyamutowa and Masunda, 2013; United Nations, 2014).
Determinants of credit demand among farmers in Hurungwe District
The determinants of credit demand among the farmers in Hurungwe District were analysed using the Binary Logistic Regression model which was employed to predict the likelihood of farmers’ credit demand based on a set of explanatory variables.
Binary logistic regression analysis results
The estimated logistic regression model was as follows:
CD = 6.675 - 0.675IR –0.771CR –0.425LBD –0.402BCA - 1.075FOD
Where:
CD = Credit Demand. The dummy dependent variable
CD = 1 if credit demanded; CD= 0 if credit not demanded.
IR = Interest Rate Price of Bank Loan in dollars ($)
CR = Collateral Requirements Loan collateral value required in dollars ($)
LBD = Lender Borrower Distance of bank’s location from the farmer in km
BCA = Bank Credit Awareness that is. Farmer’s awareness of loan products offered by banks:
1= Aware; 0= Unaware
FOD = Fear of Debt that is. Farmer’s reluctance to borrow due to fear of being in debt:
1= Fear Debt;0= No Fear of Debt.
Overall significance of the model
The Omnibus Test of Model Coefficients results (Chi-square=100.417, df=5, p=0.000) showed that the estimated model was highly statistically significant when compared to a model with the intercept only. This Likelihood Ratio test implies that the addition of explanatory variables to the null model contributed significantly to the model fitness thus enhancing its usefulness for prediction of credit demand status of farmers in Hurungwe District.
Table 3 shows the model summary results. The pseudo R2 values that is. the Cox and Snell R Square (0.262) and the Nagelkerke R Square (0.717) values derived from the Model Summary results (Table 4) show the amount of variation in the dependent variable, Credit Demand, that is explained by the model. The Coxand Snell R2 cannot achieve a value of 1 and the Nagelkerke R2 is a modification that deals with this shortfall. Therefore, based on the Nagelkerke R2 value, almost 72% of the variation in credit demand by farmers in Hurungwe District could also be explained by the estimated model. Table 4 shows the model’s category prediction results which were used to assess the effectiveness of the model’s predicted classification against the actual classification of farmers’ credit demand.
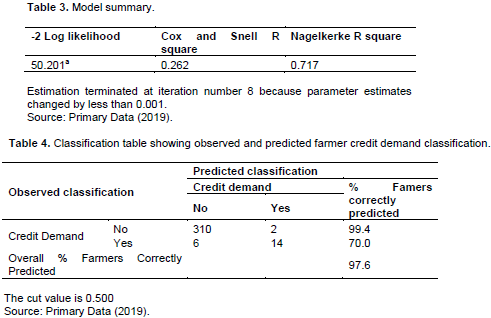
The overall Percentage Correct Prediction (PCP) was 97.6%. This means that the model, with all the explanatory variables included, correctly predicted almost 98% of the farmers in Hurungwe District in terms of their credit demand status. The sensitivity, which is the percentage of farmers that demanded credit and were correctly predicted by the model (that is, true positives) was 70%. The specificity, which is the percentage of farmers that did not demand credit and were correctly predicted by the model (that is, true negatives) was 99.4%.
Partial significance of individual variable coefficients
The Wald statistic was used to test for the statistical significance of the individual predictor variables. Table 5 shows the logistic regression coefficient B, standard error of B, Wald statistic, p-value and the odds ratio (as implied by the Exp(B)) for each of the predictor variables. Benchmarking with a significance level of 0.05, it was observed that the credit demand partial effects of Interest Rate (p=0.018), Collateral (p=0.011) and Fear of Debt (p=0.004) were statistically significant. However, Lender Borrower Distance (p=0.366) and Bank Credit Awareness (p=0.380) had statistically insignificant effects on the credit demand of Hurungwe District farmers. The following is a discussion of the effects of the individual determinant variables on credit demand.
Interest rates (IR)
Interest rate loan price had a statistically significant but negative effect (B=-0.675, p=0.018) on farmers’ credit demand (Table 5). The inverted odds ratio (1/Exp(B)=2) indicates that a marginal unit increase in the loan interest price will double the odds of a farmer not demanding credit, holding all the other variables constant. This coincided with the Law of Demand (Marshall, 1890) and the study’s apriori expectation that as interest rates increased, less farmers would apply for bank credit. These findings were consistent with various studies that also established an inverse relationship between credit demand and interest rates, for example, Ololade and Olagunju (2013); Ijioma amd Osondu (2015) and Enimu et al. (2018) that were all done in Nigeria. Studies in Zimbabwe also confirmed that interest rates charged by banks in Zimbabwe are high and segregated against poor individuals (United Nations, 2014; Vitoria et al., 2012). However, on the contrary, Sebatta et al. (2014) (Zambia) and Mukasa et al. (2017) (Ethiopia) established a positive association between interest rates and credit demand due to illiteracy challenges among farmers. Farmers in their studies were unable to read and understand market signals, and were also desperate to finance agricultural production inputs even if it meant facing exorbitant interest rates.
Collateral required (CR)
Collateral requirements by banks were a statistically significant (B=-0.771, p=0.011) negative determinant of the farmers’ demand for bank credit in Hurungwe District (Table 5). The inverted odds ratio (Exp(B)=0.462) reveals that the odds of a farmer not demanding credit will more than double by a factor of 2.2 if the collateral requirements are increased by a marginal one unit. This conforms to the study’s apriori expectation that as the collateral value demanded by banks increased, the demand for bank credit by farmers would decline. Therefore, this study infers that farmers with low or no collateral endowments have low demand for bank credit in Zimbabwe. The lack of collateral among farmers in Zimbabwe was confirmed by various studies (Masiyandima et al. (2011); Ministry of Agriculture (2013); Richardson (2005)), which ascribed it to the lack of property rights among land reform beneficiaries. Other studies in Zimbabwe also established that local banks demanded upfront immovable collateral before lending to farmers, which created a huge barrier to credit access by the farmers (FACASI, 2015; Masiyandima et al., 2011; Nyamutowa and Masunda, 2013; Vitoria et al., 2012).
Similar to this study’s findings, Kedir (2003); Akram et al. (2008) and Ijioma and Osondu (2015) studies identified lack of collateral as the major constraint limiting farmers from seeking agricultural credit in studies in Ethiopia, Pakistan and Nigeria respectively. Some studies in Ghana (Tetteh et al., 2015) and India (Samuel et al., 2015) also established higher demand for bank credit among farmers with higher collateral endowments for augmenting and replacing their capital stock and meeting the demands of all-year round production, especially where there was irrigation infrastructure. However, on the contrary, some scholars established low credit demand among farmers with higher collateral endowments. For example, Korir (2015) (Trans-Nzoia County of Kenya) and Adams (2015) (Ghana) expounded that as the collateral/ asset value in the farm increased, its owners would demand less credit because of their improved wealth and income base that made more money available in the household.
Fear of debt (FOD)
The Wald test showed an extremely statistically significant and inverse effect of Fear of debt (B=-1.075, p=0.004) on credit demand among the farmers in Hurungwe District (Table 5). Inverting the odds ratio (Exp(B)=0.341) showed that when a farmer is in fear of debt, the odds of not demanding credit increased by a multiplicative factor of 2.9. The findings were also aligned with the study’s prior expectation that as the fear of debt increased among farmers, their demand for bank credit would decline. Most farmers highlighted that they feared losing their personal possessions if they failed to pay back loans. The farmers foresaw a high likelihood of failure of their agricultural projects because of frequent drought spells that continue to occur in Zimbabwe. This was worsened by their dependence on rainfed agriculture and lack of irrigation facilities especially in Model A1 farming as supported by various studies (Chakoma and Chummun; 2019; United Nations, 2014). Similar findings were obtained in Ghana, where the fear of loan default was the most important reason that prevented farmers from participating in agricultural credit programs (Asante-Addo et al., 2017). In Zimbabwe, Dube et al. (2015) also established that the majority (63%) of farmers not accessing formal credit feared taking the risk that came with borrowing.
Lender-borrower distance (LBD)
Although Lender-Borrower Distance had a negative effect (B=-0.425) on credit demand, it was not statistically significant (p=0.366) among the farmers in Hurungwe District (Table 5). A marginal unit increase in the Lender-Borrower Distance, had the effect of increasing the odds (Exp(B)=0.654) of a farmer not demanding credit by as much as 1.5 times, based on the inverted odds ratio. The results agreed with the study’s expectation that as the distance to the bank increased the farmers’ demand for bank credit would decline. However, the statistical insignificance of distance on credit demand showed that it was not a barrier to credit demand among farmers in Hurungwe District.
Bank credit awareness (BCA)
Bank credit awareness (B=-1.075, p=0.380) did not seem to increase the likelihood of credit demand in Hurungwe District (Table 5). Contrary to expectations, the inverted odds ratio reveals that farmers, who lacked bank credit awareness, were surprisingly associated with a higher likelihood of credit demand by a factor of 1.5. This anomaly may have been the confounding effect caused by the fact that most of the farmers receive credit passively, not from the banks but indirectly, through integrated value chain financing arrangements. The study had expected a positive relationship between bank credit awareness and the farmers’ demand for bank credit. As evidenced by the statistically insignificant result, awareness of bank credit products by farmers does not influence their demand for bank credit in Hurungwe District, all other factors held constant.
Summary of logistic regression results
The Binary Logistic Regression model was employed to test and predict the likelihood of farmers’ credit demand based on selected predictor variables among the farmers in Hurungwe District. The estimated model was as follows:
Credit demand = 6.675 - 0.675 Interest rate price - 0.771 collateral requirements -0.425 lender-borrower distance -0.402 bank credit awareness -1.075 fear of debt + error term
The estimated model was statistically significant (Model Chi-square=100.417, df=5, p=0.000) implying that the addition of explanatory variables to the null model contributed significantly to its usefulness in predicting the credit demand status of farmers. Based on the Nagelkerke pseudo R2 value, the model explained almost 72% of the credit demand variation among the farmers in Hurungwe District. The overall Percentage Correct Prediction (PCP) indicated that the model correctly predicted almost 98% of the farmers in Hurungwe District in terms of their credit demand status.
The Wald statistic was used to test for the statistical significance of the individual predictor variables. Benchmarking with a significance level of 0.05, it was observed that the credit demand partial effects of Interest Rate (p=0.018), Collateral requirements (p=0.011) and Fear of Debt (p=0.004) were statistically significant. However, Lender Borrower Distance (p=0.366) and Bank Credit Awareness (p=0.380) had statistically insignificant effects on the credit demand of Hurungwe District farmers. Interest Rate loan price had a statistically significant but negative effect (B=-0.675, p=0.018) on farmers’ credit demand. A marginal unit increase in the loan interest price would double the odds of a farmer not demanding credit, holding all the other variables constant. Collateral requirements by banks were a statistically significant (B=-0.771, p=0.011) negative determinant of the farmers’ demand for bank credit. The inverted odds ratio revealed that the odds of a farmer not demanding credit would more than double by a factor of 2.2 if the collateral requirements were increased by a marginal one unit. The Wald test showed an extremely statistically significant and inverse effect of Fear of debt (B=-1.075, p=0.004) on credit demand among the farmers. When a farmer is in fear of debt, the odds of not demanding credit increase by a multiplicative factor of 2.9; although Lender-Borrower Distance had a negative effect (B=-0.425) on credit demand, it was not statistically significant (p=0.366). A marginal unit increase in the Lender-Borrower Distance had the effect of increasing the odds of a farmer not demanding credit by as much as 1.5 times. Bank credit awareness (B=-1.075, p=0.380) did not seem to increase the likelihood of credit demand. Contrary to expectations, farmers who lacked bank credit awareness were surprisingly associated with a higher likelihood of credit demand by a factor of 1.5.
Thematic analysis
Loan processing time emerged as the major theme from the verbatim responses of farmers (Figure 2). Loan processing time was mentioned by all the 33 farmers who provided verbatim responses to open-ended questions 40 times. Farmers bemoaned long loan applications processing time, indicating lack of feedback on the progress or outcome of their applications. These findings were consistent with Ijioma and Osondu (2015), Abdul-Jalil (2015), Enimu et al. (2018)and Filli et al. (2015), whose studies argued that most of the formalities involved in securing loans delayed the release of credit funds to farmers. This resulted in the farmers’ failure to meet their primary needs of bank credit like purchasing inputs, managing stock, harvesting and marketing. However, contrary to these findings, loan processing time was found to be insignificant in influencing agricultural credit demand in Rwanda (Musabanganji et al., 2015).
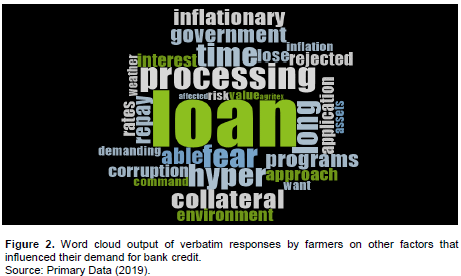
Converging with this study’s prior findings, other themes that emerged as barriers of credit demand included the farmers’ fear of debt, stringent collateral requirements by banks and the hyperinflationary environment that makes loans more expensive and out of reach of most farmers (Figure 2). These qualitative data analysis findings are supported by the frequency statistics (Figure 1), which showed that 95% of the farmers in Hurungwe District are price constrained due to high interest rates; 79% are risk constrained due to fear of losing their personal possessions in case of loan default; whilst 87% are no longer seeking bank credit due to stringent collateral requirements by banks. Logistic Regression Analysis results also converge with these findings as they established a negative relationship between interest rates, fear of debt, collateral requirements and credit demand (Table 5).