In breeding programs, obtaining breeding lines was important, and first selfing selection generations is common. In addition, an analytic approach through mixed models can lead to more success in genotype selection because it lends flexibility in analysis of unbalanced data and provides more precise genotypic values in regard to progenies evaluated. The goal of this study was to make early selection (in the S2 generation) of progenies evaluated in top crosses, using a mixed-model approach. Five hundred S2 progenies were plant derived from three populations using selection intensity (40%), which were crossbreeding with three testers. The hybrids obtained, together with control treatments, were set up in five experiments in Brazil: three in Minas Gerais, one in Santa Catarina, and one in Paraná, which evaluated grain yield. The REML method was used for calculation of variance components, and means were predicted through BLUP. The BLUPs of general combining ability (GCA) and specific combining ability (SCA) were also predicted, and the Spearman correlation coefficients among BLUPs were estimated. The dominance effects had a bigger influence on yield expression, as seen from wider amplitude in SCA values. There was an 86% coincidence considering strategy in which selection index was carried out within three populations, in relation to selection by the overall value of GCA. Considering superior hybrids, the progenies of population C exceeded the quantity of hybrids expected by 24.6%, whereas there was a reduction of 30.8% for A and 20% for B. The hybrids that exhibited the highest BLUP means were derived from crosses between progenies of population C together with the tester LE84. The low correlation among testers, both for SCA and for BLUPs, indicates that there is progeny per tester interaction.
Grain yield increase in the maize crop is directly related to the use of superior hybrids, associated with biotechnological events that contribute to taking better advantage of the genetic potential of these hybrids. Troyer (2006) reports the importance of the development of modern single hybrids that assisted in increasing maize production in the United States as of 1960. Thus, the exploitation of heterosis makes the use of hybrids in the production system viable.
Currently, there are a large number of high-yielding hybrids available to producers. Therefore, replacing current hybrids with even better ones is one of the big challenges facing breeders (Bison et al., 2003). Since this is an extremely important matter for the seed sector, breeding companies must adopt efficient breeding strategies to obtain new hybrids. In addition to its consequences for increasing crop yield, releasing new cultivars directly affects company profitability.
The probability of obtaining superior hybrids is greater when evaluating a larger number of lines and experimental hybrids. The use of adequate methodologies for identification of pairs of lines that can provide better hybrid combinations improves the efficiency of breeding programs (Iemma, 2003). In this regard, one strategy is evaluation of the combining ability between parents.
The selection of lines with greater values of general combining ability (GCA) increases the probability of obtaining high-yielding hybrids. This occurs due to greater contributions of favorable alleles in the lines. In addition, the identification of hybrids with higher values of specific combining ability (SCA) indicates the possibility of effective use of heterosis between lines (Costa et al., 2013).
One of the main steps in breeding programs is obtaining lines. In this step, early selection of these genotypes in the first generations of self-pollination is common, based on their combining ability with an elite tester line whose performance is already known by the breeder (Guimarães et al., 2012). This substantially reduces the number of lines before reaching homozygosity (Fuzatto, 2003), as well as the cost of carrying out the breeding program.
Success in early selection based mainly on GCA is due to good stability obtained over the self-pollination generations (VALÉRIO et al., 2009). In addition, this method allows greater variation to be obtained between progenies than within them, which shows the individuality of lines even in generations with a lower degree of inbreeding. A study conducted by Bernardo (1991) shows the high genetic correlation between topcrosses of S2 progenies with S6 topcrosses. This shows that it is possible to discard progenies in an efficient manner while lines are being obtained, keeping only the most promising for formation of hybrids.
In breeding programs, it is common to carry out large scale trials with many environments. Naturally, this imposes greater difficulties in controlling experimental balance, whether through the loss of plots or through the difficulty of obtaining seeds from all the crosses. Thus, data treatment that does not consider this imbalance will add error to the genotypic values and will lead to reduction in genetic gain.Analysis through mixed models is a tool that can increase success in early selection and efficiency in breeding programs. This type of analysis, especially BLUP, generates more accurate estimates of the genotypic value of the individual (Arnhold et al., 2009; Mendes, 2011), provides great flexibility of analyses, and allows the breeder to better deal with the imbalance problem, which provides more adequate comparisons between genotypes with different numbers of observations.
Under conditions of experimental imbalance, genotypes can undergo a big change in ordering of the estimated phenotypic values in relation to the genotypic values predicted through BLUP. This leads to a gain in obtaining genotypic values. Thus, BLUP has come to be considered the most accurate method for analysis in these conditions, which justifies its use in this study, in which the quantity of hybrid seeds derived from the topcrosses was not sufficient for their evaluation in all the environments, or in all the genetic combinations (Santos et al., 2016).
In light of the foregoing, this study was carried out with the aim of selecting, in an early inbred generation (S0:2), progenies evaluated in topcrosses, using the mixed model method.
The experiments were conducted from November 2011 to May 2013. In the 2011/2012 crop season, the field was set up for obtaining top cross hybrids. We used 500 S0:2 progenies derived from three distinct populations; S0:2 progenies are derived from the second generation of selfed plants from F2 populations coming from commercial hybrids commonly used in southern Brazil, composed of 175 progenies of population A, derived from the three-way hybrid Garra (Syngenta); 88 progenies of population B, derived from the single-cross hybrid AS 1532 (Agroeste); and 237 progenies of population C, derived from the single-cross hybrid 30B39 (DuPont Pioneer).
Phenotypic selection was carried out near flowering time and pre-harvest, adopting the criteria of most vigorous plants, lowest occurrence of leaf diseases, upright leaves, and uniformity of plants within the progeny, among others. Considering a selection intensity of 40%, the selected progenies were crossed with three testers, the elite line LE84, the experimental single-cross hybrid HS2532, and the commercial single-cross hybrid GNZ2004. The LE84 line was selected as an elite line from the maize breeding program and characterized as having high general combining ability. The hybrid GNZ2004 was used due to recommendation for use in same growing regions as A, B, and C hybrids. The experimental hybrid HS2532 was chosen as a good parental line and for allowing identification of high yielding three-way hybrids.
Of the 200 progenies selected, 189 were obtained with sufficient seed quantity to carry out at least one experiment, thus forming 457 top cross hybrids.
In 2012/2013 crop season, experiments were carried out at five locations, three at Crop and Livestock Scientific and Technological Development Center of UFLA (Universidade Federal de Lavras, Lavras, MG, Brazil), at different sowing times: on 09/11/2012 with 144 hybrids tested, on 16/11/2012 with 81 hybrids tested, and on 23/11/2012 with 289 hybrids. Another experiment was conducted at Guarapuava, PR, with sowing on 17/10/2012 with 316 hybrids, and at São Miguel d’Oeste, SC, sown on 07/11/2012 with 348 hybrids.
The hybrids were randomly distributed in the experiments so as not to confuse environmental effects with the effects of population or testers. Thus, 13.8% of the hybrids (63) were tested in only one experiment, 16.6% (76) were tested in only two experiments, 68.5% (312) in three experiments, 0.87% (4) in four experiments, and only 0.43% (2) were tested in five experiments, thus totaling the 457 hybrids evaluated.
Among 457 hybrids evaluated, 444 are top cross hybrids, resulting from crosses of progenies from the three populations with testers. However, for some progenies, not enough seeds were obtained in crosses with three testers, and 7.4% of the progenies were tested with only one tester, 50.3% were tested with only two testers, and 42.3% were tested with three testers (Table 1).
The number of crossbreeding from each population combination and testers can be seen in Table 2. In addition, 13 control treatments were included, consisting of 12 commercial hybrids and 1 experimental hybrid.
A simple square lattice experimental design was used in the trials set up in Lavras, MG; the first was 12 × 12, the second 9 × 9, and the third 17 × 17. In the trials set up in PR and SC, randomized blocks were used, with two replications. The experimental plots in Lavras, MG, consisted of two 5-meter rows at a spacing of 0.55 m; and in the trails of PR and SC, of four 5-meter rows at a spacing of 0.5 m.
Grain yield was evaluated as weight of grains obtained in plot, and data were extrapolated to Mg ha-1, with moisture corrected to 13%.
Data analysis was carried out through the mixed model approach, using Restricted Maximum Likelihood (REML) method for genotypic and residual variance components calculation. From fitting the model through iterative process, prediction of variance components of each random vector was obtained, as well as solution of fixed-effects vector.
Initially, BLUP mean values (u+g) of hybrids were obtained through combined analysis of data using the following model:
in which y is phenotypic observations vector; is fixed effects (locations, blocks, and overall mean) vector; g is genotypic effects vector, assumed as random; w is effects of genotype vector per environment interaction, assumed as random; and X, , and represent incidence matrix of said effects.
The estimates of the BLUPs of general combining ability and specific combining ability were carried out with the assistance of SAS software, using proc mixed. In this study, we chose to use with all genotypes within a single group model, according to Balestre et al. (2010). According to these authors, model that considers the genotypes in a single group provides more accurate estimates of GCA and SCA than model that considers two distinct groups, even with kinship information.
Thus, the following model was considered:
in which y is observations vector; is parameters of fixed effects (overall mean, locations, and blocks within locations) vector; g and s are general combining ability and specific combining ability respectively (assumed as random) vectors; ga and sa are interactions between general combining ability and specific combining ability with the environment, respectively (assumed as random) vectors; and is residues vectors.

, represent the incidence matrices of , g, s, ga, and sa effects, respectively.
In abid to analyze best manner of selecting progenies, a comparison was made between two different ranking strategies. In the first, a selection intensity of 30% within each population was considered. In second strategy, same selection intensity was adopted. However, the populations that gave rise to progenies selected were disconsidered.
The Spearman ranking correlations were estimated between BLUP means (u+g), BLUE means, and SCA. Analysis was carried out using proc corr of SAS software.
Among parameters useful for selection of genotypes, combining ability stands out through ease of interpretation. In this study, the estimate of variance of SCA was 4.15 times greater than that of GCA (Table 3).
The variance components of models can express genetic variability of genotypes, which allows inferences to be made regarding the type of gene action predominant for each one characteristics. Such a result indicates that in set of progenies under study, dominance effects have a bigger influence on expression of grain yield.
The bigger contribution of dominant effects toward an increase in grain yield is reported in various studies (Balestre et al., 2010; Bordallo et al., 2005; Guedes et al., 2011; Viana and Matta, 2003; Vivek et al., 2010; Werle et al., 2014).
In contrast, Englesong et al. (2011), Oliveira et al. (2011), Paterniani et al. (2006), and Rovaris et al. (2014) studies higher estimate of GCA in relation to SCA indicated that additive gene action contributes more toward an increase in yield. Such divergent results can be explained by the simple fact that combining ability of a genotype is relative to other genotypes being tested; thus, a determined genotype can exhibit high or low GCA, depending on genotypes that are being tested. Oliveira et al. (2011) cited that the type of allelic interaction that is predominant depends on population involved in diallel; thus, the result is specific for genotypes evaluated. Even so, it is possible to verify the predominance of additive genetic effects and of dominance simultaneously in same breeding population. Guimarães et al. (2007), seeking to evaluate maize single-cross hybrids in regard to yield from diallel crosses between divergent lines, found significant contributions of GCA and SCA, thus showing the importance of aforementioned effects.
Expression of the predominant allelic interaction also depends on the breeding intensity to which the genotypes under testing have been subjected. According to Paterniani et al. (2006), lines that have been extensively bred in distinct environments tend to have a bigger contribution from additive effects on manifestation of yield. This effect is in agreement with this study, in which progenies with a low degree of inbreeding were used that were target of phenotypic mass selection. Thus, predominance of the dominance effects on the study of genetic control of the trait in question is possible.
The magnitude of the BLUPs of the GCA for all the progenies of population A ranged from -0.1622 to 0.1780 ton ha-1. The magnitude of the BLUPs of the GCA for all the progenies of population B ranged from ‑0.1711 to 0.1399 Mg ha-1, and variation for all the progenies of population C was from -0.1182 to 0.2001 Mg ha-1. This scenario of low variation, considering the yield potential of the crop, is a direct reflection of the low estimate of the variance component related to GCA in comparison to SCA.
Oliveira et al. (2011) observed that low variance estimates of GCA were also reflected in low amplitude in the estimates of GCA of the progenies, just as observed in this study. Balestre et al. (2010) found that the variances of the BLUP values of the combining abilities (GCA and SCA), estimated from 90 S0:2 progenies genotyped with 25 microsatellite markers, were low, corroborating the results obtained in this study. These same authors discuss importance of use of progenies as a viable alternative in synthesis of high production hybrids.
In Table 4, ranking of the 57 best progenies based on GCA can be observed, considering best progenies within each population. Considering 58 progenies of population A, 17 progenies with highest GCA were selected, with variation in values from 0.01248 to 0.1780 Mg ha-1. In the case of 32 progenies of population B, the 10 progenies with highest GCA were considered, with values ranging from 0.00936 to 0.1399 Mg ha-1. As for 99 progenies of population C, 30 were selected, exhibiting GCA values from 0.03899 to 0.2001 Mg ha-1.
Considering selection of the progenies with highest values of GCA, among 58 progenies of population A, 13 were selected with GCA values from 0.0414 to 0.1780 Mg ha-1 (Table 5). In 32 progenies cases of population B, six progenies with highest GCA were considered with values from 0.0447 to 0.1399 Mg ha-1. Furthermore, among 99 progenies of population C, 38 were selected, with variation from 0.0319 to 0.2001 Mg ha-1.
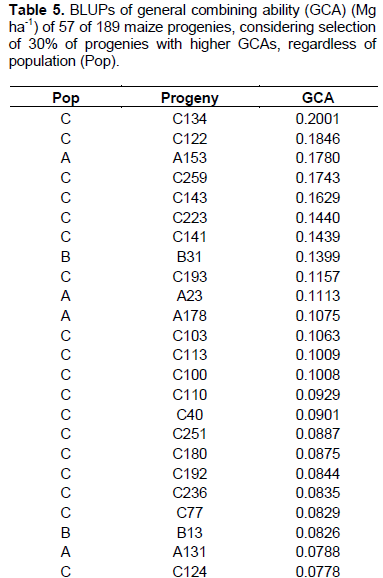
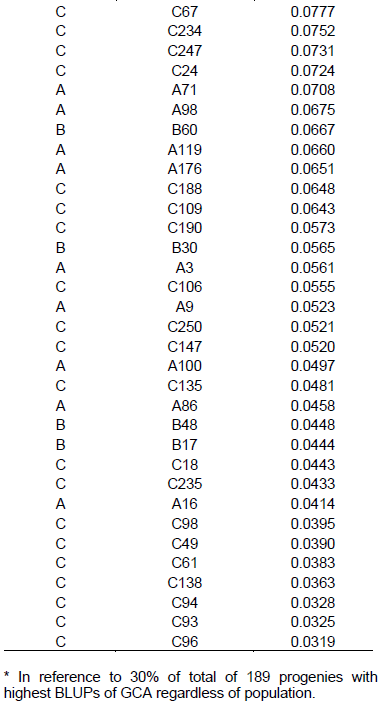
There was coincidence of 49 progenies (86%) considering the strategy in which the selection index was carried out within each one of three populations in relation to selection by overall value of GCA, disregarding pedigree of the progenies. This indicates that there was superiority in progenies production of one population in relation to others. The superiority of population C in generating progenies of higher general combining ability can be observed, considering that in the classification by overall GCA (Table 5), 8 progenies of population C were included, together with fact that there was superiority of 3.24% in GCA mean values upon using second selection strategy that is, disregarding progenies origins.
The estimates of GCA, in their magnitude, generally occur for genotypes with highest and lowest frequencies of favorable alleles in relation to mean value of genotypes tested. Thus, it is understood that purpose of selection of progenies based on highest estimates of GCA is to increase frequency of favorable alleles in following generations. Thus, it can be inferred that population C has higher frequency of favorable alleles, reflected in superiority in progenies production. The importance of high frequency of favorable alleles as a strategy for analysis of the potential of a population for extracting lines has already been discussed in literature. Bison et al. (2003), evaluating progenies derived from two different commercial hybrids, describes in regard to the estimate of m+a that there is a close relationship to frequency of favorable alleles in a population. It follows that the highest estimates of GCA can be derived from the most promising populations.
Associating progenies with high GCA values with hybrids resulting from these progenies that also have high values of SCA should be prioritized in selection because they associate a high mean and wider genetic variance. There are thus greater chances that in future generations these progenies will be lines with high potential for generating high production hybrids.
The higher expression of the dominance effects reflected in wider variance of SCA (Table 3) can also be seen by wider amplitude in the SCA values in relation to amplitude in GCA values (Table 5).
Table 6 shows 133 hybrids among 444 (corresponding to a selection intensity of 30%) with highest values of progenies of population C exceeded number of hybrids expected by 24.6%, whereas A and B had a reduction of 30.8 and 20%, respectively. From breakdown tester and population combinations, it can be observed that progenies of population C with tester LE84 resulted in an increase of 56.3% in number of hybrids among superior ones (Table 7). The only combination that generated results above that expected, except for those of population C, was combination of progenies of population B with HS2532 tester, leading to an increase of 33.3% in number of hybrids among superior ones. Included in this combination was hybrid that has highest value of SCA, B31 X 2532, with 0.6916 Mg ha-1.
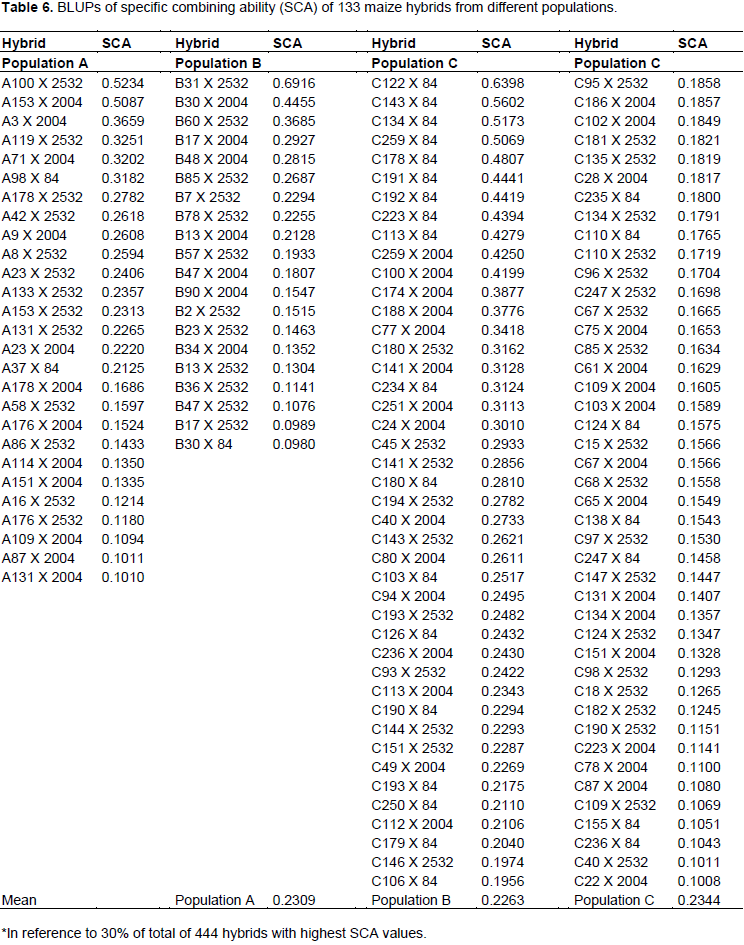
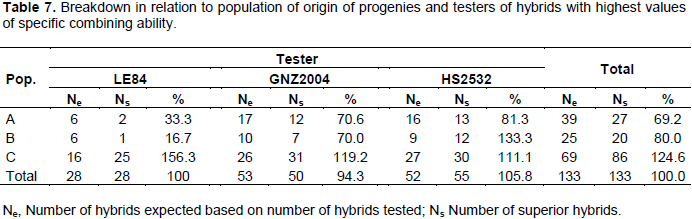
The ability to generate hybrids with superior production is connected with medium/high frequency of favorable alleles of parental lines, just as gene complementarity of parents, often expressed by high estimates of specific combining ability. Thus, progenies complementary of population C with LE84 tester can be explained by high proportion of contrasting loci between tester and population, which can, moreover, belong to distinct heterotic groups.
The high complementarity of population C with LE84 tester can furthermore be observed by SCA values, in which nine hybrids with higher SCA values are part of this combination. This scenario indicates that there is potential for obtaining high production single-cross hybrids after obtaining inbred lines derived from this population and crossed with this tester.
According to Bordallo et al. (2005), most favorable hybrid is that with highest estimate of specific combining ability, in which one of parents has higher general combining ability. Thus, we have 24 progenies example A100, A153, A3, A119, A71, A98, B31, B30, B60, C122, C143, C134, C259, C192, C223, C113, C100, C188, C77, C180, C141, C234, C251, and C24, in which hybrids resulting from these progenies have SCA values above 0.3000 (Table 6) and have high GCA values, above 0.0497 (Table 4).
To obtain inter population hybrids, populations derived from hybrids should be given priority in choice of population to be used for extracting lines. In these cases, hybrids should have high SCA estimates (Balestre et al., 2008).
There was wide variation in the estimate of SCA as a function of tester; such a situation can be important if one of the testers is of interest for obtaining hybrids in crosses with some lines that may come to be obtained from these populations. In fact, this is main argument upon using an inbred line as a tester in which result can be guide of high potential crossbreeding the future; preferentially, this tester will be from a distinct heterotic group (Elias et al., 2000).
The SCA is a very useful parameter in population choice for extraction of lines and obtaining inter population hybrids. Balestre et al. (2008), evaluating potential extraction of lines from ten commercial hybrids using mean components and mixed models with incorporation of multiallelic molecular markers, found a significant variation in SCA estimates, showing influence of non-additive effects in hybrid combinations.
Thus, considering that 40.2% of population C progenies are present among 33% of hybrids with higher SCA values (Table 6), and considering only crossbreeding with LE84 tester, these progenies of population C are present in more than half of superior hybrids. It can be inferred that greatest probability of obtaining a good hybrid will be using lines derived from population C in crossbreeding with LE84 tester. Such a situation reflects higher allelic complementarity in these crossbreeding’s. Considering that LE84 tester is an elite line, the chance of obtaining good hybrids after obtaining inbred lines from population C increases.
Fritsche-Neto et al. (2010), evaluating efficiency of REML/BLUP approach in prediction of genotypic values and in unbalanced experiments in maize crop, simulated various conditions of imbalance in number of environments, as well as in number of hybrids within environments, finding good efficiency when imbalance is up to 20% of hybrids within environments and 23% of environments. Balestre et al. (2010) also evaluated the efficiency of BLUP with the incorporation of kinship information for prediction of the production of untested maize hybrids, observing significant estimates of correlation between observed and predicted genotypic values, which ranged from 0.55 to 0.7, depending on degree of imbalance.
In general, hybrids that had higher BLUPs were derived from crossbreeding between progenies of population C with LE84 tester (Table 8).
Of 133 genotypes selected, 93 were derived from population C, equivalent to 69.69% of total, regardless of tester used. Another important observation can be made upon comparing BLUPs of hybrids with hybrids used as controls. The amplitude of variation of BLUPs of controls was 2.81, whereas top crossbreeding hybrids exhibited lower amplitude of variation, namely, 1.1.
Considering BLUPs of hybrids, a large number of top crosses that have mean values above some commercial hybrids are observed, thus showing that in addition to populations resulting in progenies with high variability, they also allow high mean values to be obtained; it should be noted that this is considered to be ideal situation for selecting progenies and obtaining lines (Alves, 2006; Ferreira et al., 2009).
To obtain genetic gains in future generations, ensuring lines with good combining ability associated with ability to generate high yielding hybrids, progenies that have high GCA and that also contribute to obtaining hybrids with high SCA should be selected in early generations. In addition to good combining ability, they should exhibit
high mean values. In this study, progenies with highest values of GCA (Table 5) that contributed to formation of hybrids with high SCA values (Table 6) and that obtained high yields (Table 8) should be selected to advance generation and obtain lines.
Except for progenies A23, A86, and C18, all progenies selected based on GCA (Table 5), also formed hybrids with high SCA values associated with high grain yields. It is thus considered that even with the greater influence of dominance effects on yield expression, additive effects should not be disregarded.
Correlation coefficients of high magnitude were manifested among SCA, BLUP, and BLUE, as well as for correlation between BLUP and SCA within each tester (Table 9). It can be inferred that, regardless of the tester, using BLUP mean values or SCA, ranking of hybrids will not change very much. Therefore, one of these parameters alone can be adopted for use in progeny selection process. Due to considerable flexibility of analysis, mixed model approach (BLUP) can be an advantageous option, and problem of imbalance can be dealt with in a better way.
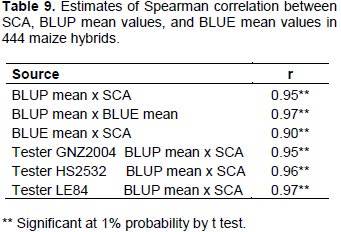
The high correlation between predicted BLUP means and estimated BLUE means can be observed in Figure 1. A similar result was found by Iemma (2003), comparing methodology of mixed models with fixed model in diallel analysis, obtaining correlation of 0.99 between BLUP and BLUE, attributing this to fact that BLUP considers matrix identity in structure of variances and covariances, and thus there are not big differences in methodology. Consequently, it can be inferred that for grain yield, classification of the hybrids undergoes little change when mixed model and fixed model methodologies were considered. Similar results were obtained by Iemma (2003).The greater correlation of SCA with BLUP mean values (Table 10) in comparison to BLUE mean values were also observed in this case (Figure 2). It can be seen that the points are distributed in a more clustered manner around the mean value. This greater correlation between SCA and BLUP can be attributed to shrinkage method effect, which approximates observations to overall mean, more intensely penalizing hybrids with a smaller number of plots and in environments with greater lack of experimental uniformity.
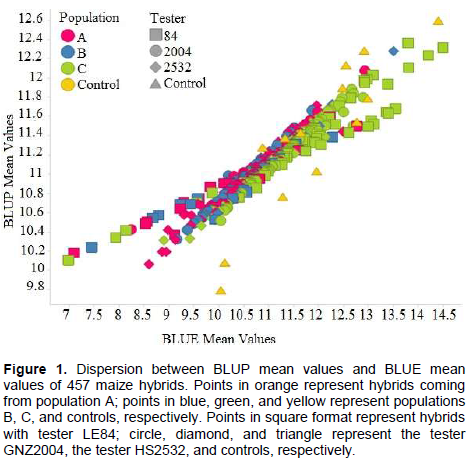
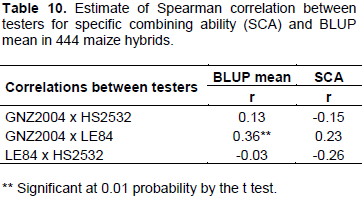
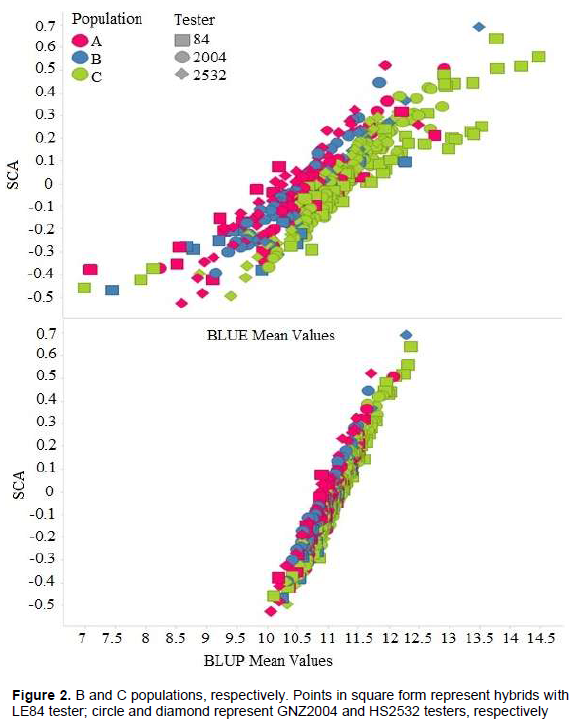
The high magnitude of correlation coefficient between SCA and BLUP mean was also observed by Balestre et al. (2008), which was attributed to fact that yield is main parameter used in these estimates. These authors furthermore report that SCA and heterosis are highly correlated with yield, and that these correlations are useful in choice of population used for extraction of lines.
Low correlation was detected between testers considering both BLUP means and SCA. Thus, production of progenies is totally dependent on tester, indicating that allelic frequency of tester is an important factor and must be considered in the progeny test. Elias et al. (2000) also reported that there is low correlation in the production and classification of top cross hybrids when different testers are used, attributing this difference to the genetic structures in populations, just as in testers.
When commercial hybrids or elite hybrids from breeding programs are used as testers, it is expected that they have high frequency of favorable alleles for diverse genes. Thus, there may be confounding effects of dominant favorable alleles in tester (Elias et al., 2000). This situation also contributes to low correlation between testers, as well as to results that do not represent real merit of progenies, but rather specific production of progeny per tester combination (Barreto et al., 2012). Even if testers with high frequency of favorable alleles are not considered ideal from conceptual point of view, results may be highly valuable if objective is to obtain three-way hybrids.
Low correlations between testers also have implications for intensity of selection because correlations between the combining abilities of progenies with diverse types of testers are normally too low to have some predictive value and, therefore, high selection intensity can only be applied to a specific tester because probability of selecting lines with high combining ability for distinct testers is very low.
Barreto et al. (2012) further state that unrelated testers used, on a strict genetic basis, as in case of single-cross hybrids, is justified because it allows expression of greater genetic variance in relation to evaluation per se of progenies, observing five times greater variance.
Thus, it can be concluded that progenies A100, A119, A131, A153, A176, A178, A23, A3, A71, A9, A98, B13, B17, B30, B31, B48, B60, C100, C103, C106, C109, C110, C113, C122, C124, C134, C135, C138, C141, C143, C147, C180, C188, C190, C192, C193, C223, C234, C235, C236, C24, C247, C250, C251, C259, C40, C49, C61, C67, C77, C93, C94, C96, and C98 were selected based on high production through GCA, SCA, and BLUP and are able to be selected to generation advance and obtain lines of high value.
The hybrids that showed greater yield potential were derived from crossbreeding between progenies of population C with 84 tester.
There was greater influence of dominance effects on yield expression, but additive effects should not be disregarded. There was high correlation between SCA and mean BLUP, allowing that one alone can be used in selection of progenies.
The low correlation between testers both for SCA and for mean BLUP indicates that there is progeny per tester interaction.