ABSTRACT
Planting and harvesting are important stages in the sugarcane crop cycle, because well planned planting and harvesting phases promote a series of benefits throughout the cultivation cycle and in the subsequent industrial use of the products. These benefits are operational, economic and environmental such as: improved utilization of the land area and transport logistics; an increase in sugarcane output; better cane reception in the factory; in administrative simplification of the industrial activities; in enhanced response to the demands of the industry; in cost planning; and in the control of pests and weeds. In this work a methodology of optimal cultivation planning to sugarcane planting and harvesting is proposed. The cultivation plan is for 5 years; and key decisions to be made in this period are to determine the planting date, the variety selection and the harvesting date corresponding for each plot such that the global production is optimized. We propose a mathematical model for this optimization task. The model uses computational and mathematical strategies to ensure that date of harvesting is always in period of the maximum maturation of the sugarcane and considers all demand and other operational constraints of the processing mill. The binary nonlinear optimization model was solved by a proposed genetic algorithm, giving an optimum plan with a potential sugarcane production 17.8% above production obtained by conventional means in the mill.
Key words: Genetic algorithm, integer nonlinear optimization model, optimal planning, Saccharum spp., sugarcane planting and harvesting.
Despite the negative perception of the society, chemical control using pesticides are still going to be used for many decades to ensure the food supply for the ever growing world population. One of the possible reasons for this is that alternative methods for plant protection are either inefficient or too costly for farmers (Wang and Liu, 2007).
Correct selection of application equipment and spray adjuvants are powerful tools to maximize pesticide efficacy, reduce detrimental environmental effects and improve the economic viability of the farmer (Dorr et al., 2014). Adjuvants are used to modify the physical, biological and chemical properties of spray mixtures to improve chemical performance (Kudsk and Mathiassen, 2007) impacting on viscosity, surface tension, contac angle, droplet retention, and deposits on the target (Gitsopoulos et al., 2014; Lin et al., 2016; Stock and Brings, 2000; Wang and Liu, 2007). The adjuvants may also influence spray atomization and formation, which is important because each type of application requires a certain optimum droplet size for its biological activity (Gimenes et al., 2013).
The spray efficacy depends on the amount of pesticide solution retained on leaves surface. In most cases, the wax on a leaf surface acts as a substantial barrier to wetting for having hydrophobic characteristic, which can make spray applications ineffective and increasing the environmental pollution (Tang et al., 2008). According to Lin et al. (2016) surfactant may not only effectively reduce the surface tension of solution but also dissolve the epicuticular waxes on the leaf surfaces and consequently, the addition of surfactant could strongly enhance the spreading ability of droplets. In order to improve targeting of the spray, it is important to know how formulation/liquid properties interact with the characteristics of the target plant to affect spray deposition (Butler-Ellis et al., 2004).
During disease and pest control applications, surfactant additives are commonly used to improve the efficiency of pesticides (Lin et al., 2016). Several studies showed that surfactants can greatly reduce surface tension and maximize the spread, penetration, and absorption efficacy of pesticides on leaf surfaces (Gimenes et al., 2013; Gitsopoulos et al., 2014; Lin, et al., 2016). Besides foliar uptake and biological efficacy of the active ingredients are improved with the surfactant by overlapping some leaf barriers such as cuticular membrane, trichomes and others features that decrease
droplet deposition, spread and uptake of pesticide solutions.
The global planted area of Eucalyptus crop has increased significantly in the last decades. The trend in areas where Eucalypt are being grown in plantation is that pest and pathogen problems are increasing (Wingfield et al., 2003). Despite the contamination problems presented by the use of pesticide, this practice is required in various situations to control satisfactory pest and disease. The addition of corrected adjuvants on tank-mixture could enhance the performance of pesticides on pest, weed and disease control. The comprehension of adjuvants behavior is fundamental to prescribe the correct product/concentration maximizing pesticide control, avoiding losses and environmental contamination.
The aims of this research were to identify (1) the most effective and economic adjuvants/concentrations in reduce surface tension of water and (2) the spray retention capacity of adjuvants/concentrations on leaves of different Eucalyptus species used as a target surface.
Surface tension determination
Static surface tension (SST) assessments were performed at the Laboratory of Pesticide Application Technology Laboratory, at the College of Agricultural Sciences UNESP - Botucatu, SP - Brazil. Details of the adjuvants composition selected for evaluations are summarized in Table 1. The adjuvants were tested at 11 concentrations levels (0.001; 0.0025; 0.005; 0.01; 0.025; 0.05; 0.1; 0.25; 0.5; 1.0 and 2.0% v v-1) plus an additional treatment with no adjuvant (distilled water).
γ = (wt × 72)/ww (1)
Where: γ = Surface Tension (mN m-1); wt= Droplet weight (g) of treatments and ww= Droplet weight (g) of distilled water.
Statistical analysis of each adjuvant data was performed using the SAS program (SAS Institute, 1995) and regression analysis was used the Mitscherlich equation model, denoted by γ = a[1-10c-(x + b)]. Mitscherlich model was modified to get better fit to the data (Silva et al., 2006), expressing relationship between surfactant input and surface tension reducing.
Modified model used: γ = γdw - a[1-10-cx]
Where γ: surface tension in mN m-1; γdw: distilled water surface tension (72 mN m-1); a: maximum horizontal asymptote attainable in the original model; c: curve concavity representing the efficiency of the surfactant. Higher value of this parameter represents the most effective the surfactant is to attainable the minimum surface tension in a lower concentration; x: surfactant concentration (%v v-1); γdw - a: corresponds to the minimum surface tension reached by adding surfactant in aqueous solution. To compare the effects between adjuvants on SST a factorial design 6 adjuvants x 11 concentrations were analyzed.
Spray retention determination
Eucalypti leaf spray retention was evaluated by the same adjuvants used to determine SST. The experimental design was done in a factorial 6 (adjuvants) x 5 (Eucalyptus species: Corymbia citriodora, Eucalyptus urophylla, E. camaldulensis, E. torelliana and E. grandis) x 8 (adjuvants concentrations at 0; 0.005; 0.01; 0.05; 0.1; 0.5; 1.0 and 2.0% v v-1) with seven replicates (each leaf was considered one repetition). Eucalyptus mature leaves were classified by Gaskin et al. (2005) as an easy moderate-to-wet. The Eucalyptus leaves were collected from a four years old plants grown outdoor located at Plant Protection Department - UNESP – Botucatu, Brazil. Eucalyptus branches were removed from the trees and immediately carried to the laboratory. At the time of tests the branches base was kept into a 10 L pot filled with water to avoid leaves humidity losses. Eucalyptus mature leaves were removed from the branches and determined the weight by analytical precision balance (Marte, model AY 220, São Paulo, SP, BR). Afterwards the leaves were placed in a vertical position (holding the petiole leaves by hand) and the solution was sprayed by an adaptation of Potter Spray Tower (Burkard Scientific, Uxbridge, UK) sprayer at 100 kpa pressure, typically producing fine spray droplet diameter. The spraying was performed on both sides of leaves at the distance of 0.3 m until run-off point was reached at temperature of 21 ± 2°C and 50 ± 7% air relative humidity. After spraying the leaves were again weighted and by weight difference the spray solution retained on each leaf was determined. Area of each target was measured using a foliar area meter (Licor Inc., Li 300, Lincoln, NE, US). The weight value was divided by the foliar area of each leaf and the retention results expressed in µg cm-2.
Statistical analysis
Spray retention and surface tension dates were subject to analysis of variance (ANOVA) using SISVAR Statistical Software (Ferreira, 2011). Fisher’s least significant difference (LSD) was calculated to identify significant difference between mean treatments at 5% probability.
Surface tension study
The median values of surface tension (mN m-1) of adjuvants on 11 concentrations levels are showed in Table 2. Significantly difference were verify in the interaction adjuvants x concentrations (F=77.4; p<0.001). All aqueous solutions containing the adjuvants reduced the surface tension of destilled water with the increased concentration. The adjuvant heptomethyltrisiloxane (HT) presented the best performance to reduce surface tension of aqueous solution follow by polyoxyethylene alkyl phenol ether (PAPE), mineral oil (MO), nonylphenoxy polyethoxy ethanol (NPE), vegetal oil (VO) and soyal phospholypids and propionic acid (SPPA) (Table 2). Stevens et al. (1993) verify a more rapid and more extensive reduction of surface tension on aqueous solution when organosilicone surfactant is used.
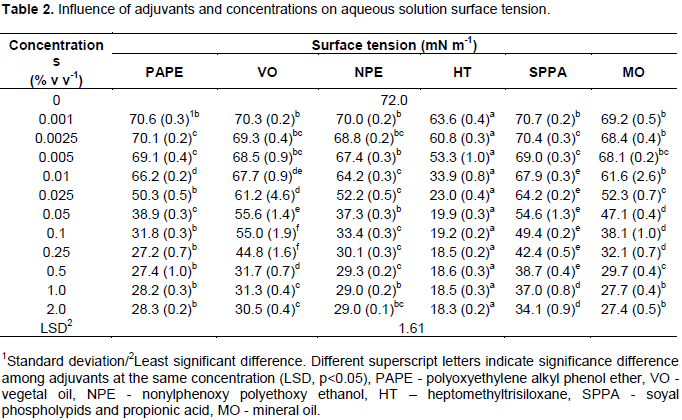
The surface tension value of HT (18-19 mN m-1) for the aqueous solution is significantly lower compared to the other adjuvants tested. Lower surface tension of trisiloxanes surfactant (approximately 22 mN m-1) measured by a pendent drop technique is reported by Wang et al. (2015). The surface tension of HT was a little lower than reported by those authors, probably due the different technique performed in this research. SPPA is the adjuvant with least ability to lowering surface tension of aqueous solutions at all adjuvants studied. Similar to this research Nairn et al. (2014) observe surface tension values approximately of 19 mN m-1 with HT and 31.5 mN m-1 with (alcohol ethoxylate both at concentration of 0.1%. Iost and Raetano (2010) report approximately surface tension values of 19 and 33 mN m-1 for aqueous solutions of the adjuvants HT and SPPA respectively using the same technique adopted in this research.
The SST became steady state at concentrations up to 0.25% to PAPE, 0.5% to VO, 0.25% to NPE, 0.05% to HT and 1% to MO (Table 2). These minimum concentrations points are considered to be the Critical Micelle Concen-tration (CMC) (Aliverdi et al., 2009). When a surfactant concentration is above the CMC the surfactants produce aggregates called micelles and generally the minimum equilibrium surface tension is achieved (Hazen, 2000). An increase of concentration above the CMC will not modified the surface tension. The CMC of the adjuvant SPPA, apparently, was not reached by the concentrations tested in this research as illustrated in Table 2.
Variance analysis results of surface tension by Mitscherlich modified model are shown in Table 3. High coefficient of determination (R2) values and low coefficient of variation (CV) indicate that these equations provided good models profiles and accurate estimate of SST of the aqueous solutions containing adjuvants. According to parameter “c” the adjuvant HT is the most efficient adjuvant to reach the minimum surface tension in a lower concentration (46.3) follow by NPE (11.9); PAPE (11.3); MO (7.8); SPPA (4.6) and VO (4.3) (Table 3).
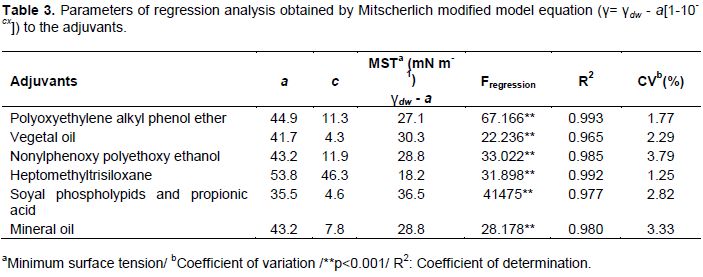
Linking the minimum surface tension values adjusted by the Mitescherlich model can be verify that HT present the lower SST to 18.2 mN m-1 follow by PAPE (27.1 mN m-1); NPE and MO with the same value (28.8 mN m-1); VO (30.3 mN m-1) and SPPA (36.5 mN m-1) as show in Table 3. Stevens et al. (1993) demonstrate that the reduction in surface tension of aqueous solutions is the most important factor controlling droplets adhesion and the organosilicone surfactants must have an advantage because they reduce aqueous surface tension more quickly than conventional hydrocarbon-based surfactants. HT showed lower surface tension values (33.9 mN m-1) at concentration of 0.01% v v-1 (Table 2). Despite the adjuvants NPE and MO reached the same minimum values of surface tension they presented different values of “c” parameter of 11.9 and 7.8 respectively (Table 3).
Spray retention study
A regression analysis among adjuvants concentrations did not fit well in any model with coefficient of determination low than 40% and for this reason a mean comparison was analyzed.
The average retention on leaves provides by all adjuvants and all concentrations on Eucalyptus species are given in Figure 1. Significant difference on spray retention values (F= 47.8; p<0.001) on leaves of Eucalyptus species is detected. Leaves of E. toreliana had the highest retention with the mean values differing significantly to the other species. Lowest retention value was attributed to leaf of E. grandis (Figure 1a). Spray retention difference verified between the species could be attributed to leaf surface structure as for example surface micro-roughness, trichomes and microcrystalline waxes composition which can vary from Eucalyptus species. Lin et al. (2016) verify larger wetted area on E. tereticornis leaves comparing to leaves of eucalipt hybrid urograndis (E. urophylla × E. grandis) at any surfactant concentrations
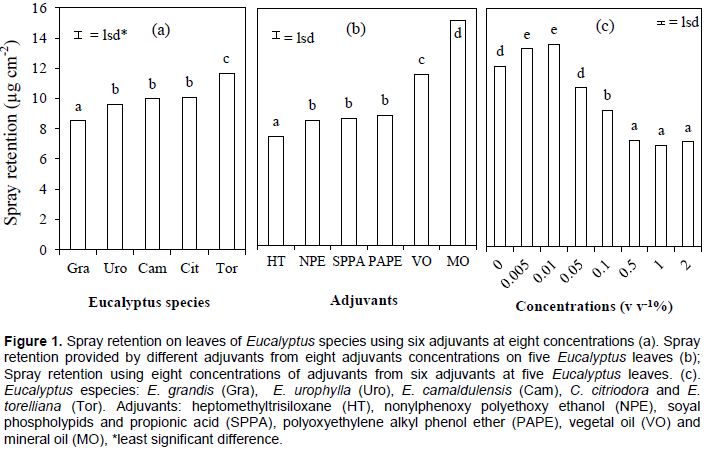
A significant effect of adjuvant concentration in spray retention on Eucalyptus leaves (F= 189.2; p<0.001) was observed (Figure 1c). Spraying retention reduction was verified as adjuvant concentrations increased becoming steady at concentration of 0.5%. Concentrations over 0.1% reduced the spray retention on leaves Concen-trations of 0.005 and 0.01% increased spray retention differing significantly from treatments applied with only distilled water. The highest spray retention (13.6 µg cm-2) appeared on the adjuvant concentrations of 0.005 and 0.01% increased by 2 times over the concentration of 1% (6.8 µg cm-2).
Gaskin et al. (2000) report that aqueous solution of the insecticide acephate with addition of spreader-sticker adjuvants reduced spray retention on cucumber plants. The effects were attributed to observable spray droplets coalescence and run-off. As this research had the spraying done until run-off point (maximum volume of leaf saturation) the spray retention decreased as the adjuvants concentrations increased. In the previous papers published by Matuo and Baba (1981); Ocampo-Ruiz and Matuo (1994); Oliveira et al. (1997) and Silva et al. (2008) is verified reduction of spray retention liquid capacity on leaves when spraying was made with aqueous solution containing spreader-sticker adjuvants at high spray volume. Pesticide application using adjuvants which reduce surface tension must ensure more security when is done at high spray volumes in order to avoid run-off and consequently pesticide losses.
It is very important attempt that the values of spray retention obtained in this research were realized using only water plus adjuvants. Different spray retention results can be found when used solution with pesticide formulation due to the different characteristics. Lin et al. (2016) studying the effects of surfactant concentration on the spreading properties of pesticides, observe as nonionic surfactant concentration increased continuously from 0.1% to 0.25, 0.5 and 1%, the wetted area of solution (water + surfactant) droplets present a reduction rather than expansion trend on surface of Eucalyptus leaves. When the solutions contained pesticide the trend of wetted area is increase as surfactant concentrations also increase. The same authors conclude that spread properties using only water are distinct than those pesticide droplets containing surfactant and a specific spray solution has an optimal spreading efficacy at a specific surfactant concentration
The adjuvant heptomethyltrisiloxane show the best performance on reducing surface tension of aqueous solution at the lowest concentrations and exhibited the highest efficiency. Spray retention on Eucalyptus leaves varies with the species. E. grandis leaves had the higher amount of spray retention and C. citriodora the lower.
Mineral oil was the adjuvant which provides the best spray retention on Eucalyptus leaves. The adjuvants concentrations of 0.005 and 0.01% increased foliar spray retention while 0.5, 1.0 and 2.0% decreased foliar spray retention. Pesticide spray application using high volumes in the presence of adjuvant which reduces drastically the surface tension should be carefully performed to avoid losses by run-off, increasing the cost of production and environmental pollution.
The authors have not declared any conflict of interests.
Thanks are due to the CAPES for financial support to the first author. Commercial products or trade names in this publication is solely for the purpose of providing specific information and does not imply recommendation.
REFERENCES
Buddadee B, Wirojanagud W, Watts DJ, Pitakaso R (2008). The development of multi-objective optimization model for excess bagasse utilization: A case study for Thailand. Environ. Impact Asses. Rev. 28:380-391.
Crossref
|
|
Colin EC (2009). Mathematical programming accelerates implementation of agro-industrial sugarcane complex. Eur. J. Oper. Res. 199:232-235.
Crossref
|
|
Center of Sugarcane Technology (CTC) (2012). Varieties CTC 2012, Brazil.
|
|
Florentino HO, Pato MV, Jones D, Cantane DR (2015). Biomass Production and Uses. In: Production and Management of Sugarcane Biomass Process Optimization. InTech.
View
|
|
Florentino HO, Pato MV (2014). A bi-objective genetic approach for the selection of sugarcane varieties to comply with environmental and economic requirements. J. Oper. Res. Soc. 65:842-854.
Crossref
|
|
Higgins AJ, Muchow RC, Rudd AV, Ford AW (1998). Optimising harvest date in sugar production: A case study for the Mossman mill region in Australia I. Development of operations research model and solution. Field crops Res. 57:153-162.
Crossref
|
|
Holland J (1975). Adaptation in natural and artificial systems. Ann Arbor University of Michigan Press, MI, USA.
|
|
Holland JH (1992). Adaptation.in natural and artificial systems. MIT Press, MA, USA.
|
|
Jena SD, Poggi M (2013). Harvest planning in the Brazilian sugar cane industry via mixed integer programming. Eur. J. Oper. Res. 230:374-384.
Crossref
|
|
Leboreiro J, Hilaly AK (2011). Biomass transportation model and optimum plant size for the production of ethanol. Bioresour. Technol. 102:2712-2723.
Crossref
|
|
Piewthongngam, K., Pathumnakul, S., Setthanan K. (2009). Application of crop growth simulation and mathematical modeling to supply chain management in the Thai sugar industry. Agric. Syst. 102:58-66.
Crossref
|
|
RIDESA (2008). Inter-university Network for development of the sugarcane industry. Varieties RB.
|
|
Rudorff BFT, Aguiar DA, Silva WF, Sugawara LM, Adami L, Moreira MA (2010). Studies on the Rapid Expansion of Sugarcane for Ethanol Production. in São Paulo State (Brazil) Using Landsat Data. Remote Sens 2:1057-1076.
Crossref
|
|
Salassi ME, Breaux JB, Naquin CJ (2002). Modeling within-season sugarcane growth for optimal harvest system selection. Agric. Syst. 73:261-278.
Crossref
|
|
Scarpari MS, Beauclair EGF (2010). Optimized agricultural planning of sugarcane using linear programming. Invest. Oper. 31:126-132.
|
|
Stray BJ, van Vuuren JH, Bezuidenhout CN (2012). An optimisation-based seasonal sugarcane harvest scheduling decision support system for commercial growers in South Africa. Comput. Electron. Agric. 83:21-31.
Crossref
|