Access to information and new technologies avails smallholder farmers an opportunity to improve their production decisions. However, access to information on technologies per se may not be relevant if the technologies are not adopted. This study was aimed at determining the factors that enable participants to access information on integrated soil fertility management (ISFM) technologies and to apply these technologies in groundnut production. It was conducted in the major groundnut producing districts of Tororo, Mbale, and Bukedea; where 155 Producers were surveyed. Probit and Multinomial Logit (MNL) models were fitted to the data using STATA analytical package. The results reveal that on average, producers in production groups were more likely to access information and to use new groundnut production technologies compared to their counterparts without groups. In addition, group membership, sex of farmer, and education also pre-disposed producers and processors to access and use new groundnut technologies. Smallholder farmers should be encouraged to form and work in groups as they facilitate knowledge sharing and technology adoption.
Agricultural production in most of sub-Saharan Africa (SSA) is predominantly smallholder based and charac-terized by low input and output systems. Agricultural production in this region is heavily nature driven with the lowest rates of application of external inputs in the world. For example, per-capita application of chemical fertilizers between 2010 and 2011 averaged only 8 kg per hectare of cultivated land much lower than any other developing region of the world (World Bank, 2014), with wide variations across countries. There is limited adoption of improved varieties, post-harvest handling methods and value addition techniques at subsequent storage and processing stages, even though these improved technologies are available at the respective national agricultural research institutes.
In Uganda, there is a glaring disparity between production at research stations and under farmers’ conditions (Nabbumba and Bahigwa, 2003; Pender, 2004). For instance, groundnut production on research stations is in the order of 2.7 to 3.5 ton per hectare while at farmers’ fields, it only averages 0.6 ton per hectare (Development Strategy and Investment plan (DSIP, 2010). In addition, adoption of improved technologies along the groundnut value chain is dismal. Whereas there are a number of technologies from production to marketing, most smallholder groundnut farmers are still stuck with the traditional practices. The majority of smallholder groundnut farmers plant their traditional varieties of groundnuts, they sometimes sell unshelled groundnuts and there is little or no value addition to their products. Use of low cost appropriate shellers and groundnut paste making machines is almost non-existent in the rural areas. When the smallholder farmers sell their groundnuts in primary form, they practically transfer their margins to the other chain participants.
This research was motivated by the desire to establish the determinants of soil fertility-enhancing technology use by smallholder groundnut farmers in Eastern Uganda.
This research was conducted in the purposively selected districts of Bukedea, Tororo and Mbale in Eastern Uganda. These districts are among the major groundnut producing areas in Uganda. In addition, they are in close proximity to Serere National Agricultural Research Institute, which conducts research aimed at improving groundnut production in the country. From each of the three districts, one sub-county was randomly selected and from the selected sub county groundnut farmers were also randomly selected using production lists drawn by the production coordinators. In total of 155 smallholder groundnut farmer, respondents were selected and interviewed from all the three districts.
Theoretical framework
Adoption can be defined as the application of a given technology as it is recommended (Ashby, 1991) and the degree of use of a new technology in the long-run equilibrium when full information is available about the new technology and its potential (Feder et al., 1985). In its simplest form, Rogers (1962) describes adoption as a process of willingly accepting innovations by members of a social system. When a new innovation is introduced, farmers go through a period of becoming knowledgeable about the new technology, to forming positive or negative attitudes toward the technology and ultimately to deciding whether to adopt the technology or not. Factors influencing the adoption of new agricultural technologies can be divided into three major categories: farm and farmers’ associated attributes; attributes associated with the technology (Adesina and Zinnah, 1993) and the farming objective (CIMMYT, 1993). On the other hand, other authors (Mauceri et al., 2007) categorized these factors under social, economic, managerial and institutional factors. In the farm and farmer category, the farmer’s level of education, age, or family and farm size are considered. The second category depends on the type of technology (e.g., the kind of characteristics a farmer likes in a given technology package: improved seeds, plant spacing, fertilizer application, weeding frequency among others). The third category assesses how different strategies used by the farmers, such as commercial versus subsistence farming, influence the adoption of technologies.
Analytical framework of adoption

Depending on the distribution of the error terms ei, a number of models can be used to determine the probability of adoption. In the literature, three models have been frequently used to analyze technology adoption: (1) linear probability, (2) logistic function (logit), and (3) the normal density function (probit) models (Verbeek, 2004; Salasya et al., 2007). Between the logit and probit models, choice of one and not the other largely depends on individual preference (IDRE, 2015) as the two yield the same output except when the binary variables are endogenous in which case the probit model is used to deal with selection bias (Heckman, 1979). The two models are only different in the assumption about the distribution of the errors.
These models use a binary choice variable as a dependent variable. The binary choice models also known as univariate dichotomous models describe the probability that an event will or will not happen, or as used in this context, that a technology will or will not be adopted, although the models are often derived from an underlying latent variable model (Salasya et al., 2007). For example in order to fit the probit regression model, the dependent variable is measured as equal to 1 for adopters and 0 otherwise.
Estimating joint adoption decision
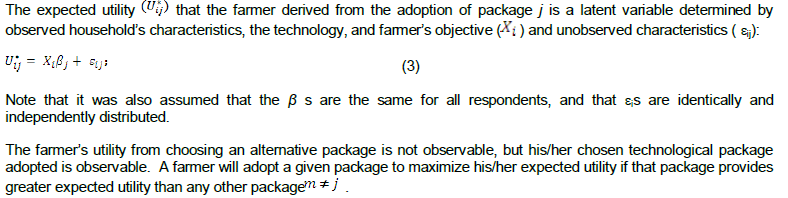
Estimating marginal effects of adoption decision
Marginal effects are an informative means of summarizing how change in a response is related to change in a covariate. With binary independent variables, the marginal effects measure discrete change, that is, how do predicted probabilities change as the binary independent variable changes from 0 to 1. Marginal effects for continuous variables measure the instantaneous rate of change, similar to measuring change in the probability of a success as a covariate increases by one. As such they provide a good approximation to the amount of change in Y (the decision to adopt a soil fertility-improving technology) that will be produced by a unit change in the independent variable while holding other variables in the model constant at some value, usually the mean. As such, for a categorical variable:
Marginal effect Xk = Pr(Y=1|X, Xk=1) – Pr(Y=1|X, Xk=0)
where Y is the dependent variable, in this case the choice of adoption, Xk is the categorical variable, and X is the vector of all other variables held at their mean value.
For the continuous independent variable:
Marginal effect of Xk = Pr(Y=1|X)*Pr(Y=0|X)×βXk
Table 2 shows the descriptive statistics of the continuous variables used in the model. The mean age of respondents was 43 years and households were large with a mean of 15 people including children, seniors and adults of productive age. The mean educational attainment was only 7 years implying that the household heads had at least primary level education. Households owned land in excess of 5 ha which is higher than the national average of 2 ha per household.
Results of the probit models
Table 3 contains results of the probability of adoption of soil bands as an ISFM technology in Eastern Uganda. The variables used in the analysis include membership in a farmer’s organization, household size, respondents’ age and sex, land owned in 2012, value of assets (UGX) and access to training on soil conservation. The probit approach shows that membership to a farmers’ organi-zation positively influences the adoption of soil bands pointing to the importance of collective action in adoption of improved technologies. Farmers who are members to certain organizations are sometimes more advantageous than their non-member counterparts, because organizations sometimes have projects that are funded for their members and under these projects the farmers may adopt new technologies and varieties. These organizations also provide free training services to the farmers, post-harvest handling and processing facilities, may provide provide them with planting material as well as extension follow-ups when necessary. Membership to groups also enhances access to information and learning/ training on the modern or improved technologies thereby fostering technology use and uptake by the farmers.
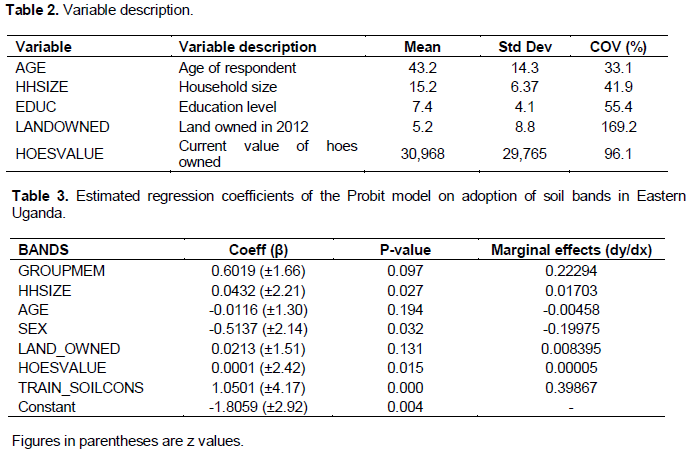
Household size is also positively related to the adoption of soil bands as an improved soil fertility technology. Household size is an indication of labor availability. Labor availability for farm has also been found to influence the decision to adopt improved soil and water conservation technologies (Yila and Thapa, 2008; Mazvimavi and Twomlow, 2009; Mugonola et al., 2013). Therefore, the number of family members or adult equivalents (labor) available for farm work is likely to influence whether a technology is adopted or not. For example if a technology is labor intensive to implement like soil bands, unavailability of family labor for farm work may deter the household from adopting the new technology while availability of household labor may enhance the adoption of such improved technologies.
Sex of the farmer was significantly and negatively related to the probability of adopting soil bands as a soil improving technology for groundnuts. This is however contrary to the findings of Moyo et al. (2007), Mamudu et al. (2012), Mugonola (2013) in which the sex of the farmer was found to significantly and positively influence adoption of IPM technologies. These authors report that male headed households are more likely to adopt improved technologies, especially those that are labor intensive.
The current value of the hand hoe positively and significantly influenced the adoption of soil bands. This is because the hand hoe is the major technology supporting agricultural production and land improvement technologies in sub-Saharan Africa mainly because of its user friendliness. However, although positively related to adoption, the power of its coefficient is small implying that it increases adoption of soil bands to a smaller extent. The expected sign for this variable was a negative because the higher the price of an implement, the less farmers would have been expected to adopt soil bands. It is therefore because of its primary importance (and demand) in smallholder agriculture that the value of the hand hoe is positively related to adoption; implying that even if the value of the hand hoe increased, farmers would still demand for it to carry out agricultural production including constructing soil bands.
In addition, the results in Table 3 also show that access to training in soil and water conservation positively influences the adoption of soil bands. Through trainings, farmers access information concerning availability and expert knowledge on the use of agricultural technologies. Training of farmers through institutional support especially from public extension services have been found to influence the adoption of new technologies (Shiferaw and Holden, 1998; Läpple and Rensburg, 2011).
The results in Table 4 show the factors influencing the adoption of contours. The variables used in the analysis were membership in farmers’ organization, household size, age and sex of the farmer, how much land the household owned and farmers’ access to training in soil and water conservation. The results show that household size, sex and access to training in soil and water conservation technologies significantly influenced the adoption of contours. From the results, household size positively influences adoption of contours which is in line with findings above. Given the labor intensiveness of soil fertility improvement technologies, a large household size implies availability of labor to implement soil fertility improvement technologies. Gender of the farmers was found to positively influence the adoption of contours which is not in congruence with the findings related above where sex was found to negatively influence the adoption of soil bands. The positive influence of sex in adoption of soil bands may be due to men’s access to information about the technologies or the women’s lack of information on the technologies (Bonabana-Wabbi and Taylor, 2012).
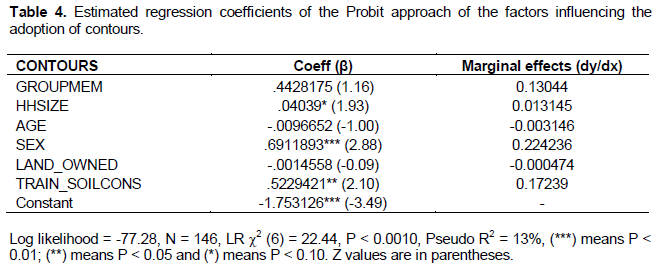
Training in soil and water conservation was positively related to the adoption of contours which is also in line with the findings in Table 3. Receipt of extension training enhances farmers’ knowledge on his or her production and equips the farmer with new techniques of managing agricultural production; so the farmer could then easily adopt improved methods of farming like the use of contours. For example in a study by Mugisha et al. (2005) extension was found to positively influence the adoption of improved groundnut technologies with the probability of adoption increasing by 40.5% if an extension agent visited the farmer. Extension visits enable the farmer to get information about new or improved technologies and the extension workers encourage them to adopt. Extension agents also establish relationships with these farmers who then act as contact farmers and thus can be selected to participate in training and demonstrations (Bisanda et al., 1998).
When the variables in Table 2 are held at their mean values, the marginal effect of each covariate in Tables 3 and 4 show the effect of a change in the independent variable on the probability of adopting a soil fertility-enhancing technology. For example, group membership increases the probability of adopting soil bands by 22.3% for a sample of 43 year old men owning 5.2 ha and whose household size is 15.2 people. With regard to contours, group membership increases the probability of adoption of contours by 13% for the same sample.
Multiple ISFM technology adoption
Table 5 shows results of the multinomial logits for multiple adoption of technologies. The variables used in the logit models include household size, total area cultivated by the household, membership in a farmers’ organization, receipt of training on chemical fertilizer, receipt of training on green manure, age of the farmer, education (no. of years in school) experience in farming and amount of land owned by the household. The results show that household size is negatively related to multiple non-adoption of soil fertility improvement technologies implying that a higher household size is likely to influence multiple adoption of soil fertility improvement technologies.
Total acreage cultivated by the farmer is positively related with non-adoption of multiple ISFM technologies and is significant at the 5% level; implying that the larger the size of cultivated acreage, the higher the probability that people would not adopt multiple ISFM technologies. This may be attributed to the higher labor demands by ISFM technologies compared to the competing labor demands from other agricultural enterprises. This makes farmers less likely to take up ISFM technologies. Membership in a farmers’ organization is negatively related to non-adoption of multiple ISFM technologies and is significant at 5% level. This finding was expected because membership in farmers’ organization has been found in literature to be positively related to adoption of agricultural technologies. This is because farmers in groups easily access information from each other and this fosters learning among such members. This helps members to try out the new technologies. This is therefore the reason as to why membership in farmers’ organization is negatively related to multiple non-adoption of agricultural technologies.
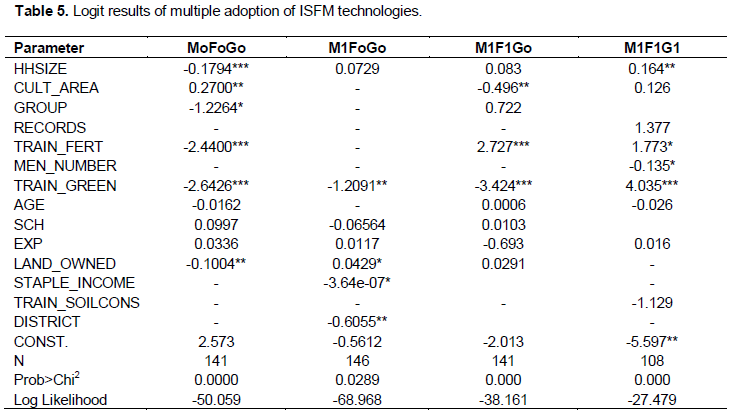
Receipt of training on green manure and chemical fertilizers were found to negatively influence non-adoption of multiple ISFM technologies and it is significant at 1%. This is line with what is found in literature in which it is stated that extension training enhances farmer learning thereby leading to knowledge acquisition which increases the likelihood of a person adopting agricultural technologies. This is therefore the reason as to why this variable is negatively related to non-adoption of agricultural technologies. The amount of land owned by the farmer is also negatively related to non-adoption of ISFM technologies and this variable is significant at 10%. This finding was expected because land is an important asset in agricultural production and since land improvement technologies like ISFM requires devotion of land to the specific enterprises that require use of the ISFM technologies; it is the reason as why land owned is negatively related to non-adoption of ISFM technologies.
The results further show factors that affect adoption farmyard manure as a soil fertility improvement technology. The variables used in the model include household size, receipt of training in green manure, education measured as the number of years completed in school, amount of land owned by the household, annual income earned from staple crops production and location of the farming households (in terms of the district). The results show that receipt of training on green manure was negatively related to adoption and non-adoption of farmyard manure and chemical fertilizers and green manure, respectively. This finding is significant at 5% and it implies that receipt of training in green manure does not necessarily increase the likelihood of adopting farmyard manure while receipt of training on green manure would positively enhance its adoption.
The amount of land owned by the household is positively related to adoption of M1F0G0 as a combination of soil fertility improvement technologies. The variable is significant at 5% level of significance. The positive relationship shows that farmers with higher land ownership are more likely to adoption the said combination of soil fertility improvement technologies. The implication of this finding is that farmers are keen to improving the soil fertility status of their owned land and will adopt soil fertility improvement technologies for so long as they are going to be applied on their piece of land.
The annual income from staple crops production is negatively related to adoption of soil fertility improvement technologies and is significant at 10% level of significance. The negative relationship may be attributed to farmers’ choice of soil fertility improvement technologies. Certainly, when farmers realize higher incomes from crops production, they are more likely to adopt more superior soil fertility technologies like chemical fertilizers which are easier to apply and are less bulky compared to farmyard manure.
The location of the farmer is also negatively related to adoption of soil fertility improvement technologies and is significant at 5%. A farmer belonging to a particular district influences his or her likelihood of adopting or not adopting the soil fertility improvement technologies. The location of the farmer influences adoption because it is attributed to availability or non-availability of information regarding the availability of improved ISFM technologies.
Farmers’ choice of two soil fertility improved technologies was also analyzed. This analysis was focused on the probability of farmers’ likelihood to either adopt two soil fertility improvement technologies or otherwise. The factors influencing theprobability of adoption were also estimated. The amount of total acreage cultivated is negatively related to adoption choices of the farmer and is significant at 5%. This means that as more land is cultivated, the probability of adopting soil fertility im-provement technologies is reduced. When farmers have bigger acreages, it may be more difficult for them to apply technologies and cover the whole acreage because even though the production costs per unit of output decline with increased economies of scale they may not have resources to acquire the technologies. This may discourage adoption of such soil fertility improvement technologies. Additionally, the more the acreage cultivated by farmers the less efficient farmers become in managing their production hence the less likely it is for farmers to adopt productivity enhancing/soil technologies.
Farmers’ receipt of training on use of chemical fertilizers was positively related to adoption of soil fertility improvement technologies. The variable is significant at 1% implying the role of agricultural information dissemination through training in technology adoption and commercialization of the agricultural sector. However, farmers’ receipt of training on green manure was negatively related to adoption of soil fertility improvement technologies (farmyard manure and chemical fertilizers). This means that a farmer who is trained on green manure is less likely to adopt a combination of soil fertility improvement technologies involving both chemical and farmyard manure but not involving green manure. Additionally, this finding can also be interpreted in a way that farmers who have received training on green manure are more likely to adopt green manure as a soil fertility improvement technology implying the importance of agricultural training on technology adoption. It is therefore the reason as to why training on green manure has appeared to be negatively related to the adoption of soil fertility improvement technologies whenever the combinations of soil fertility improvement technologies do not involve adoption of green manure.
The Multinomial Logit (MNL) results show farmers’ choice of three soil fertility improvement technologies (farmyard manure, chemical fertilizers and green manure). The independent variables that were used in the model included household size, total acreage cultivated by the farmer, record keeping, number of male members in the farmers’ group, receipt of training in soil and water conservation, training on green manure, training on use of chemical fertilizers, training on farmyard manure and the amount of land owned by the household. Household size was positively related to multiple and joint adoption of soil fertility improvement technologies and was significant at 5%. Household size is an indication of labor availability to implement the soil fertility improvement technologies, hence the reason it is positively related to multiple adoption. Additionally, household size is an indication of knowledgeable individuals whose ability and willingness to use the soil fertility improvement technologies is high.
The number of males (men) in a farmer group is negatively related to multiple adoption of soil fertility improvement technologies; implying that the higher the number of males in the farmer group, the less likely is the probability of adopting soil fertility improvement technologies by the household. This may be attributed to the role of males in household resource allocation and decision making. Since most households are headed by males, their knowledge of soil fertility improvement technologies through group involvement may not necessitate multiple and joint adoption of soil fertility improvement technologies because household resources may be required in order to adopt. Their unwillingness to devote household resources towards adoption of soil fertility improvement technologies may lead to the negative relationship between their number in a farmer group and their adoption decision. Additionally, the preferences in the available technologies may lead to the negative relationship between male numbers in a group and multiple and joint adoption decisions. Most males may prefer taking up only one technology for example chemical fertilizers as opposed to taking up three technologies. The number of male farmers who were willing to take up all the three technologies was only 10 compared to 68 who were unwilling to take up the three technologies. Therefore, extension policy efforts to ensure effective dissemination and uptake of multiple soil fertility improvement technologies using the farmer group approach should ensure an equal balance of both males and females in farmer groups. This is because the number of females in a farmer group is positively related to multiple and joint adoption of soil fertility improvement technologies.
Training on green manure was found to positively influence multiple adoption of soil fertility improvement technology as opposed to earlier findings where it was found to negatively influence multiple and joint adoption decisions. This finding is significant at 1% level and it shows that unlike earlier findings (given Tables 4 and 5) where farmers’ receipt of training on green manure was negatively related to adoption decision, it is positively related to adoption of a combination of three soil fertility improvement technologies (farmyard manure, chemical fertilizers and green manure). This implies the importance of green manure in technology adoption and the interpretation of this finding is that training on green manure is important in the adoption of soil fertility improvement technologies. This may be attributed to farmers’ perception regarding its use in agricultural production. It may be considered as a base technology by farmers who want to adopt a number of soil fertility improvement technologies.
Farmers’ receipt of training on chemical fertilizer was positively related to multiple and joint adoption of soil fertility improvement technologies and is significant at 10% level implying the importance of access to agricultural information on multiple adoption decision making of the farmer. However, farmers’ training on farmyard manure was negatively related to multiple and joint adoption of soil fertility improvement technologies implying that receipt of training on farmyard manure may discourage farmers’ adoption of other soil fertility improvement technologies. This may be due to the ready availability of soil fertility improvement technologies in the local environments because of the available cattle. The results of the logit model and multinomial logit models are not presented here but can be availed upon request.
The survey was carried out in three districts (Tororo, Mbale and Bukedea) of Eastern Uganda as part of a larger study on up-scaling of integrated soil fertility management (ISFM). Farmer interviews were made to both adopters and non-adopters of the targeted technologies in the targeted crops. The specific objectives included: to characterize smallholder agricultural production in Eastern Uganda; analyse the determinants of adoption of ISFM technologies, both as single interventions and as part of a technological package.
Our probit model results for single-technology adoption showed that household size and membership to a farmers’ organization were positively related to adoption of soil bands implying the importance of collective action in adoption of improved technologies. On the other hand, sex of the farmer was significantly and negatively related to the probability of adopting soil bands. Receipt of training in soil and water conservation positively influenced the adoption of soil bands implying the importance of farmer training in fostering information availability and technology uptake by farmers. Additionally, receipt of agricultural training was also found to positively influence multiple and joint adoption decisions. Thus, extension policy should therefore be strengthened to disseminate agricultural information on improved technologies for uptake by farmers.
Multiple-technology adoption decisions were modelled using a multinomial logit model. Results indicate that access to training, amount of land and household size were significant factors influencing adoption of multiple technologies. The availability of family labor is critical to being able to carry out labor intensive farm activities that enhance soil fertility. In addition membership to farming groups is important for adoption, perhaps because information flow and labor access (through labor sharing arrangements) is enhanced.