ABSTRACT
This study analyses the effects of school resources on student mathematics achievement in Zimbabwe using a 3-Level Hierarchical Linear Model (HLM). The major findings of the research are that a teacher trained in the relevant subject, class size, having a highly qualified school head and having a generally high resource endowment at school level improve student test scores. However, text book possession was seen as an insignificant predictor of student achievement. The implication of these findings is that resources owned by schools have an important effect on student test scores, suggesting that value and more attention should be given to what goes on in schools; as well as improving quality of human resources at school level. The study however has a limitation in that it does not fully explore all the probable school and class level determinants of student achievement due to data constraints.
Key words: School resources, student achievement, mathematics, hierarchical linear modeling.
Upon attaining independence in 1980, Zimbabwe embarked on an ambitious drive to increase student enrolment particularly in the previously marginalised black communities, and it greatly succeeded over the years . The key drive in this initiative is “the education for all” campaign where primary school fees were waived to encourage massive enrolments and the mobilisation of resources to build more primary schools. For instance by 2007, Zimbabwe had 5560 primary schools compared to 3116 in 1980 (Makopa, 2011). Unfortunately, this increase in both net and gross enrolment has been accompanied by a fall in overall student achievement. This is of great concern, bearing in mind that human capital accumulation is an important resource to a country’s economic growth. An educated labour force is likely to be more productive (Glewwe et al., 2011) than the less learned, hence the need to ensure that resources invested in human capital development are effective. Also, on the issues of poverty alleviation, such investments are vital considering that well-educated people have a better chance of improving their standard of living than the less educated. This kind of investment is particularly important in the rural areas of Zimbabwe, where the majority (60%) of the population resides (World Bank, 2014) and 70% of the people live below the poverty datum line (Nyamanhindi, 2014).
When analysing the composition of the students who fail their primary school-leaving examinations, rural primary schools have not been performing well on average compared to those in the urban areas. Research has attributed such low outcomes to inadequacy of school resources to influence student achievement, hence well-resourced urban schools have outperformed their rural counterparts (Nyagura and Riddell, 1993; Makopa, 2011). It is worth mentioning however that, rural areas are not a total right off; there are other rural primary schools which outperform those in urban areas. With such a background, this research sought to investigate whether school resources have an impact on primary school students’ performance in the rural areas. It also sought to establish which resources are important in enhancing the students’ achievements. Such findings are important in informing policy, considering that resources are scarce, and they have to be spent effectively to achieve the desired goal of educational achievement. Other than this paper, there are two similar papers identified in Zimbabwe which tend to vary in terms of methodology, focus and data employed. Nyagura and Riddell (1993) adopted a multilevel modelling procedure in ascertaining the percentage variance in primary school achievement attributed to different types of schools found both in rural and urban areas.
The study adopts a stratified random sample with a fair representation of the dominant tribes in the country, Shona and Ndebele. Though its methodology is robust, it tends to compare urban and rural schools, where it is visibly evident that urban schools outperform their rural counterparts. Makopa (2011) used SACMEQ III (collected in 2007) data to assess the changes in the availability of the basic teaching and learning provisions in Zimbabwe primary schools as well as establishes how these resources were related to the pupil’s achievements. This is a nationally representative research that groups both urban and rural schools, and it uses a descriptive methodology, which is not robust at all. Notwithstanding the fact that Nyagura and Riddell (1993) adopted a rigorous econometric approach, their paper is two decades old, and might no longer be relevant in informing policy, and their objectives are different from those of this paper. On the other hand, Makopa (2011) used a simple descriptive analysis in his study. With the discovery of more sophisticated research methodologies in this current era, this paper does not appeal much to policy (Glewwe et al., 2011).
The principal conceptual framework employed in educational outcome literature is the production function approach. This approach as with the conventional production function has inputs (such as school resources, socio-economic characteristics, and teacher characteristics), schools as ‘factories’ and outputs (mainly student achievement). Hence, an education production function defines the structural relation between inputs and achievements. In general, it can be depicted as:
A=f(S, Q, C, H, I)
With A denoting achievement, S years of schooling, Q a vector of school and teacher characteristics, C a vector of child characteristics, H a vector of household characteristics, and I a vector of inputs controlled by the parents (Glewwe et al., 2011). Though researchers in this area might differ in methodologies employed and data source and characteristics, they are guided by this framework. Many researchers concur that the seminal work of the Coleman et al, (1966) played a significant role in stirring the debate about the impact of school resources on student achievement (Rumberger and Palardy, 2004; Ehrenberg et al., 2001). In this respect, several researches, varying within space, time and methodological approaches have been performed in a bid to validate or reject the findings of the 1966 report. Though the Coleman report’s survey initially focused on measuring the extent of racial segregation of American schools, it collected comprehensive data on students’ test scores (aged 8, 11, 14 and 17 years), their family background, their teacher attributes, the schools they attended, and the characteristics of their communities (Ehrenberg et al., 2001). Such data enabled the researchers to learn whether students’ achievements were influenced by the variables in question. The research stated that variations in family background and community level characteristics better explain student achievement differentials across schools than were variations in school resources (such as pupil/teacher ratios or expenditures per pupil), and teacher characteristics (such as experience and degree levels). The Coleman report’s findings came under heavy criticisms in the 1980s through the findings of the study by Heyneman and Loxley (1983) study. They bemoaned the tendency of some scholars to assume that findings from one part of the world could be generalised as being applicable to the other part. In particular, the researchers observed that the debates about the significance of school resources in influencing educational outcomes were based on data from developed parts of the world (such as the USA, Europe, and Japan). These were then taken as if they applied even to the less developed nations. Heyneman and Loxley thus examined the Second International Mathematics and Science Study (SIMSS), a data set covering both developed and developing countries and contrary to the Coleman report, school characteristics were observed to be more important than family socioeconomic status in determining student achievement.
Students’ socio economic status had a weaker impact on their academic achievement, rather the quality of schools and teachers to which children are exposed to affect students’ achievement. Such insightful findings motivated Baker et al. (2002) two decades later to test whether the “Heyneman-Loxley effect” was still valid, considering there had been significant increases in enrolment and provision of school resources and there have not been a systematic review of the “Heyneman-Loxley effect”. Baker et al. (2002) examined data from the Third International Mathematics and Science Study (TIMSS) of the 1990s and estimated using Ordinary Least Squares (OLS) and observed that the “Heyneman-Loxley effect” had subsided. The research concluded that what determined student outcomes after such significant developments were factors external to the school. They however did not completely rule out the Heyneman-Loxley effect, as they consent that it could still be valid in those countries still rooted in deep poverty, unrests and epidemics which hampered mass school enrolments . Greenwald et al. (1996) employed meta-analytic methods on a variety of production function researches and revealed that school resources had a positive influence on student outcomes. Variables such as pupil expenditure and teacher remuneration were observed to have a significant positive and consistent influence on student achievement.
Also, having small schools, low student-teacher ratios, and teacher quality were seen to positively impact on student outcomes. Hanushek (1997) however maintained that there is no significant or consistent relationship between school resources and student’s educational outcomes, at least after variations in family inputs are taken into account, reaffirming the Coleman report’s findings. This study employed meta-analytic approaches as used by Greenwald et al. (1996), reviewing 400 educational production literature. He argued that Greenwald et al. (1996) overall sampling was biased on retaining both statistically significant positive and insignificant but positive results, just the direction that leads to supporting their general conclusions. Therefore, Hanushek (1997) argued that simple resource policies held little hopes for improving student outcome. Using data from the South African Living Standards Survey (SALSS) of 1993, questionnaires on local facilities, and a literacy and numeracy survey, Case and Deaton (1999) examined the relationship between educational inputs (pupil-teacher ratios and school facilities) and school outcomes (including school attendance, educational attainment, and test scores) in South Africa. Their analysis shows that pupil-teacher ratios have a marked impact on black children’s outcomes, holding constant a mix of teacher and other resources. They attribute it to the discrimination against the black community by the apartheid regime that which enabled other races to have significantly better school resources and lower pupil-teacher ratio. Hence, they advise of a need to improve resourcing in disadvantaged schools in order to improve student achievement. Such results to an extent conform to the “Heyneman-Loxley effect”, considering Baker et al. (2002) suppositions of stating that the severely marginalised communities need schools that are well resourced for them to raise student achievement.
Smith (2011) however argued that policy focus should be wider than just school resourcing levels and facilities in South Africa; as has been embarked on by the current government to narrow the gap between the educational attainments between races. He argues that there is a need to empower deprived neighbourhoods so that they can overcome acute social disadvantages that impact on student achievement. These include having poor nutrition, lower fluency in language of instruction and children having to travel long distances to attend school. To arrive at these results, Smith (2011) developed multilevel models for individual learners of similar socio-economic status to ascertain determinants of their achievement. The data used are grade 6 mathematics and reading scores obtained from SACMEQ II surveys of the year 2000. With a multilevel modelling technique, Nyagura and Riddell (1993) investigated the causes of primary school achievement variance between different primary school categories in Zimbabwe. In the analysis, the primary schools were placed into 5 categories; high fee paying (private), former group A, former group B , low fee paying, and rural district primary schools. Using the official Ministry of Education’s final leaving examinations (grade 7), the paper found that for both subjects, group A and high-fee-paying schools performed better than the rest, followed by group B and low-fee-paying schools, and at the bottom, district council schools.
District council schools are those located in the rural areas. Primary school student achievement were seen to be influenced by the amount of teacher training and instructional time, and pupil-teacher ratio for both subjects and instructional time particularly for mathematics, and these issues were prevalent in the rural areas. Makopa (2011) using SACMEQ III reading and mathematics scores of grade 6 pupils, sought to establish the availability of basic classroom resources and their impact on pupils’ achievements. The research shows a strong relationship between schools having more resources and increasing achievements in reading and mathematics tests. Relatively well resourced provinces, which happen to be metropolitan provinces in Zimbabwe (Bulawayo and Harare), performed better than their rural counterparts. This research however was a descriptive study without any econometric analysis. This paper recommended future research to focus on within province variations, to see whether resource differentials affected student outcomes. Glewwe et al. (2011) highlights important issues pertaining to the impacts certain methodologies have on the credibility of such research findings. In their review of educational achievement published between 1990 and 2010, they observed mixed results, which in actual fact are influenced by the quality screening the researchers subject the papers to. In particular, this paper reviews literature that estimates the impact of school infrastructure and pedagogical materials, teacher and principal characteristics as well as the general school organisation on student learning and time in school.
The reviewed researches employed various methodologies such as Randomised Controlled Trials (RCT), Difference in Differences (DD) regression, Regression Discontinuity Design (RDD) and Ordinary Least Squares (OLS). It starts with over 9,000 studies, but scales down to 79, 43, and eventually 13, screening them on the basis of the econometric method employed by the selected studies in what they term a ‘quality test’. Most school and teacher characteristics are statistically insignificant, especially when the evidence is limited to the “high quality” studies, thought availability of desks, teacher knowledge of the subjects they teach, and teacher absence do have significant effects. They conclude that having a fully functioning school is conducive for student learning.The quality of the econometric approach is important in educational research. There is need to ensure that the researcher is aware of the shortcomings of the methodology employed, and most importantly employ a more rigorous model such that the findings are closer to the obtaining situation on the ground. Some reviewed literature such as the Heyneman and Loxley (1983), Baker et al. (2002) and Case and Deaton (1999) adopted the ordinary least squares approach in estimating the determinants of student achievement. This methodology however does not pass the ‘Glewwe test’ as the paper regards such methodology is inadequate to correctly estimate the impacts such resources have on student achievement.
Though not mentioned by Glewwe et al. (2011), the methodology adopted by Makopa (2011) is equally insufficient. Simple descriptive statistics are not rigorous at all, and would not have passed the Glewwe test. Those which pass the test are Randomised Controlled Trials (RCT), Difference in Differences (DD) regression, Regression Discontinuity Design (RDD) and OLS regressions that further employ more sophisticated methodology to control for potential omitted variable or endogeneity bias. The paper by Nyagura and Riddell (1993) becomes more sophisticated than the simple OLS regression as it takes into account the inherent clustering nature of students into classes, clustered in schools, which are further clustered into districts. Such a methodology in cross sectional data is plausible. However, the other methodologies suggested by Glewwe et al. (2011) prove to be expensive and difficult to employ (such as natural experiments and RCT). To sum it up, the reviewed literature on estimating the effects of school resources on students’ achievement is ambiguous and contested. This however does not render such contrasting results futile; in fact, important lessons are deduced which help improve future research outcomes.
For instance, the realisation by Heyneman and Loxley (1983) that research findings from one part of the world cannot be misconstrued to be universally applicable. Such generalisations are likely to misinform policy, which might lead to a waste of scare resources, negatively impacting economic growth, and livelihood outcomes. Similarly, Baker et al. (2002) effort of testing the validity of the Heyneman-Loxley effect within time is equally relevant. It shows that in this dynamic world, no research findings can withstand time. So much changes as years go by and there is need to constantly invest in updating research findings for policy to remain relevant. Makopa’s (2011) recommendation for a further research to test the significance of school resources on within-province achievement differentials is equally valid. This suggestion is in line with the arguments of Heyneman and Loxley (1983). Also, methodological issues raised by Glewwe et al. (2011) highlight the importance of knowing the limitations of each methodology and make possible corrective measures such that findings do not deviate much from what is factual. Basing on this review, this research sought to establish whether school resources have an influence on primary school students’ outcomes in rural Zimbabwe, bearing in mind that the methodology to be employed is of critical importance.
Conceptual model
The schooling process is considered as a multilevel system that processes inputs into outputs. Thus a school is loosely equated to a ‘factory’, though in this instance the inputs are human, and the outputs are student achievement (Glewwe et al., 2011). The multilevel concept emanates from the realisation that a student’s achievement is influenced by factors that can be split into 3 distinct categories, which are student level factors (such as cognitive ability, family socio-economic status, age and gender), classroom level factors (such as teacher characteristics, class size and availability of learning resources), and lastly school level factors (such as the school climate, school size, and the availability of learning resources such as libraries and laboratories) (Rumberger and Palardy, 2004). All these level factors have an influence on the effectiveness of this ‘factory’ in processing its inputs into outputs. Rumberger and Palardy (2004) present this interaction in Figure 1.Such conceptual models are important as they guide the design of the study, such as the selection of participants, variables and the model to be employed in data analysis (Rumberger and Palardy, 2004).
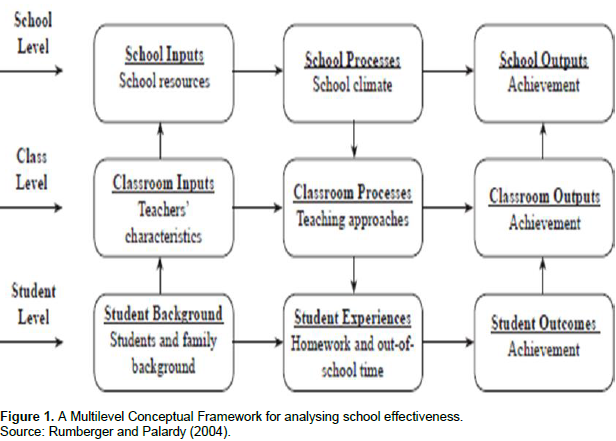
Data
Data was obtained from the Southern and Eastern Africa Consortium for Monitoring Educational Quality (2014), a consortium comprising of Southern African educational ministries and other stake holders whose main aim is to monitor educational quality amongst member states. The consortium has embarked on a large scale cross national research study in member states to assess the conditions of schooling and performance levels of learners and teachers in the areas of literacy, numeracy as well as the learners’ basic health knowledge. Since Zimbabwe is a member state, this research was made easier by the availability of such rich data, which is costly and almost impossible to collect as an individual due to many impediments.
The study population
In Zimbabwe, the SACMEQ III’s study population were all the pupils at the grade 6 level attending registered schools, as well as their teachers and the school heads of such schools. The desired, defined and excluded population statistics are presented in Table 1. The desired population is the total number of grade 6 pupils, their teachers and school heads at all registered educational institutions. The project excluded grade 6 enrolments of fewer than 20 students, hence the excluded population column on the Table 1.
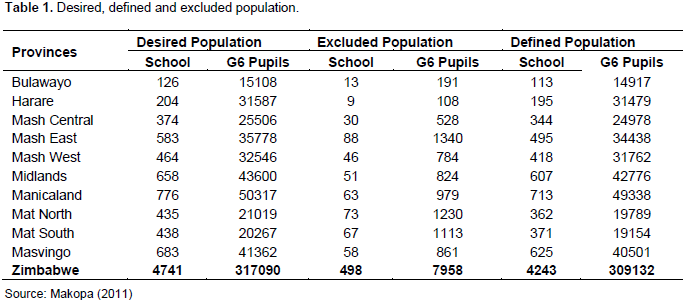
The study sample
The SACMEQ III project used a 2 stage cluster sampling approach to select a sample of 3021 grade 6 students from 155 schools, 155 heads, and 274 teachers were also selected (Makopa, 2011). To get the winning schools per province, a lottery was drawn, and a simple random sampling approach was employed to select the students per school. The consortium treats urban and rural schools as equals in its sampling approach, which hampers the success of this research to achieve its objectives. But however, since Matabeleland South Province is predominantly rural, there are higher chances that the majority of the selected students hail from rural areas. The researcher assumes that the results obtained by the SACMEQ III in Matabeleland South Province are more a reflection of the rural students as opposed to the urban ones. The SACMEQ’s response rate in Matabeleland South is relatively low, and Makopa (2011) attributes such low response rates to administration problems within this province. This further justifies the need to interrogate the effectiveness of these areas in churning out human capital. Table 2 is a summary of the planned and achieved sample as well as the percentage response rate per province.
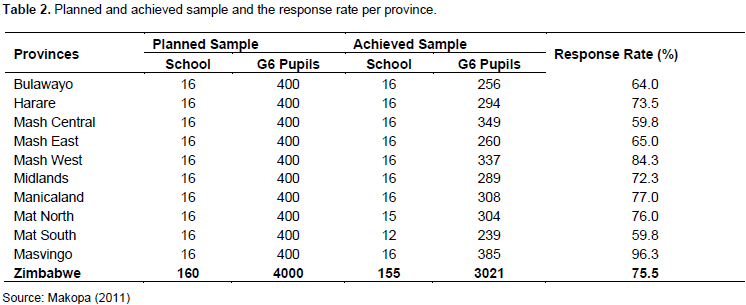
Method of analysis
This research adopts a HLM or Multilevel Regression Analysis (MRA) in order to achieve its set objectives. This method of analysis is rightly applicable to this kind of research considering the type of data to be analysed, which is nested, or clustered in nature. Previous researchers such as Raudenbush and Bryk (1988), Nyagura and Riddell (1983), and Ker (2014) have applied it to similar researches. Employing traditional analysis such as the Ordinary Least Squares (OLS) approach was observed to ignore dependence in the data that results from grouping, whereas analysis at the group level did not permit straightforward inferences or predictions at individual level (Ker, 2014). A fundamental assumption of most statistical models is that observations included in the analysis are ‘independent’ or uncorrelated with one another (Putnam-Hornstein, 2013). It is assumed that educational interventions have a constant effect on all students who are exposed to them, and these effects are invariant across organizational contexts (Bryk and Raudenbush, 1988). It is assumed these structures lead to less efficient parameter estimates, losing the fascinating interrelationships between the different levels (Nyagura and Riddell, 1993) since hierarchical data violates this assumption. The individuals clustered within one group are likely to be more similar to other members of the group than to individuals clustered within another group (Putnam-Hornstein, 2013).
This dependence is prevalent in schools because of the shared experiences among students and because of the non-random assignment of students to schools which is usually based on location. This dependence is also evident in survey research whenever a cluster sample is employed. As is applicable in this research, a sample of students drawn purely at random will provide a more precise base for statistical estimates than would a sample of students drawn through a two-stage procedure of first selecting a set of schools at random and then drawing students at random from within them (Bryk and Raudenbush, 1988). Moreover, ‘the inherent nesting of educational systems in which students are nested in classes which, in turn, are nested in schools; which themselves are nested in districts or regions, making the covariance within each level of direct interest (Nyagura and Riddell, 1993). Hierarchical linear modelling resolves this problem by incorporating the unique effects of individual schools into the statistical model for the outcome, thus the estimates adjust for the intra-class correlation that emanates from cluster sampling (Bryk and Raudenbush, 1988). Multilevel analysis helps explain student achievement as a function of student school-level or classroom-level characteristics, while taking into account the variance of student outcomes within schools (Webster et al., 1996).
Model specification
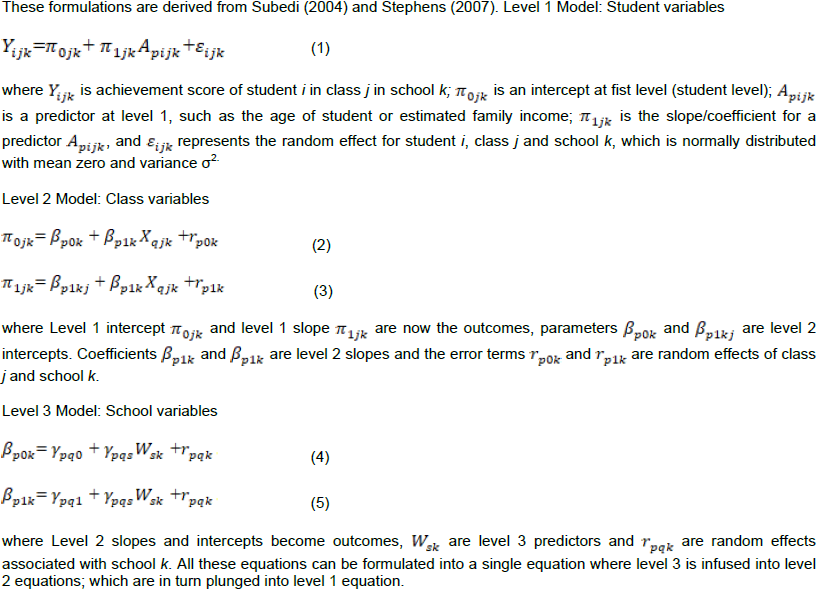
Variables
The variables considered in this research come in 3 levels, conforming to the theoretical framework and the model choice. It is important to note that this study has limited its variable selection, because of time constraints. This does not imply that the SACMEQ III variables are limited to the extent of this paper’s analysis.
Student achievement [dependent variable]
The standardised student mathematics test score is taken as a dependent variable to assess the impact of school resources on student achievement.
Student gender
Student's gender: Male =1, Female =0. The impact of gender on student achievement is a contentious issue in the student achievement discourse. It can be argued that depending on the socialisation processes within different cultures, this has an impact on how children view school and hence their achievement. In cases where a boy is considered as a bread winner and a girl as a child and family minder, one would expect that the boy performs better than the girl. However, literature provides mixed results to this effect. This paper, since it dwells on a rural setting which is dominantly patriarchal, would prematurely predict that boys do better than girls.
Student repetition
This variable reveals whether a student has ever repeated in his/her studies. The SACMEQ III questionnaire has a provision for one to respond as either never repeated, repeated once, twice or trice or more times. However, this paper recorded the responses as either yes or no. This limits the analysis, but then such recoding makes it easier to analyse. Usually, students repeat because they will not have progressed well in their studies. This research takes this variable as an indicator of a student's cognitive ability.
Parents' educational status
Parents' highest education level is actually the father's education level (or male guardian). It is compared between those without ordinary level, to those who have attained ordinary level or higher. This research assumes that the ordinary level is a meaningful cut off, comparing the overall impact of a parent's educational level on student outcome.
Class size
This is the number of children attending grade six in a sampled class according to the teacher's register. As discussed in the literature review section, this variable is contentious. Some studies argue that small class sizes enable students to achieve more, whereas some provide evidence that large classrooms enhance student achievement.
Availability of mathematics textbook
SACMEQ III presents a set of questions pertaining to text book availability in the class. These questions interrogate the availability, number, whether shared or not. This study uses information on whether there are mathematics text books allocated to students or not at any given time. Conventional wisdom states that the availability of textbooks goes a long way in empowering a student to achieve good grades as the student is able to follow what he/she is taught, and is able to revise or practice on his/her own. There is however evidence showing that having a textbook does not guarantee that a student will fare well in his/her studies. This could be because of other factors such as the inability of the child to use the book resourcefully or simply a lack of a reading culture in him.
Total school resources
This variable is obtained from the school head's response to questions about the availability of certain facilities within the school. These are ranked out of a maximum of 22, and each school is gauged on this benchmark.
School head qualification
The school head's qualifications are based on the responses pertaining to his/her actual academic training. The responses range from no professional training at all to more than 3 years of training. The researcher believes that such training is critical as the head understands what teachers go through on a daily basis and can easily monitor them to ensure that they are effective.
This paper performed four different regressions, using the Statistical Package for Social Scientists (SPSS) version 22 to better reveal whether school resources had any effect on student achievement. Firstly, a regression was run on Matabeleland South province data, and the behaviour of the school resources were analysed. The second regression was performed on the best performing province in Zimbabwe, which is Harare. This province is predominantly urban, and the behaviour of school resources on student achievement was also noted. Thirdly, we ran a regression on Matabeleland South and Harare data combined, and a dummy variable for being either a student in Matabeleland South (=1) or Harare (=0) is introduced. On the final regression, the researcher took advantage of the richness of the SACMEQ III data which sampled the entire country. Thus a regression on the complete SACMEQ III data was regressed on student maths achievement scores. At this stage, provincial dummy variables for all provinces were introduced to better understand how school resources impacted on student achievement at national level. Here, presents these results and also appropriate tests were performed to ensure that the results are robust.
Descriptive statistics
There were 4 different regressions performed in this analysis: the Matabeleland province (MTS), Harare province (HAR), combined Matabeleland and Harare provinces (MTS HAR) and lastly on the national sample (ZIM). The MTS and HAR data have no dummy variables; hence, no values are inserted on these rows. The MTS HAR data set has a dummy on either being enrolled at a school in MTS or HAR. Lastly, the national sample has provincial dummies indicating from which province a student studies. Table 3 shows the mean, standard deviation and the number of observations for each variable (N).
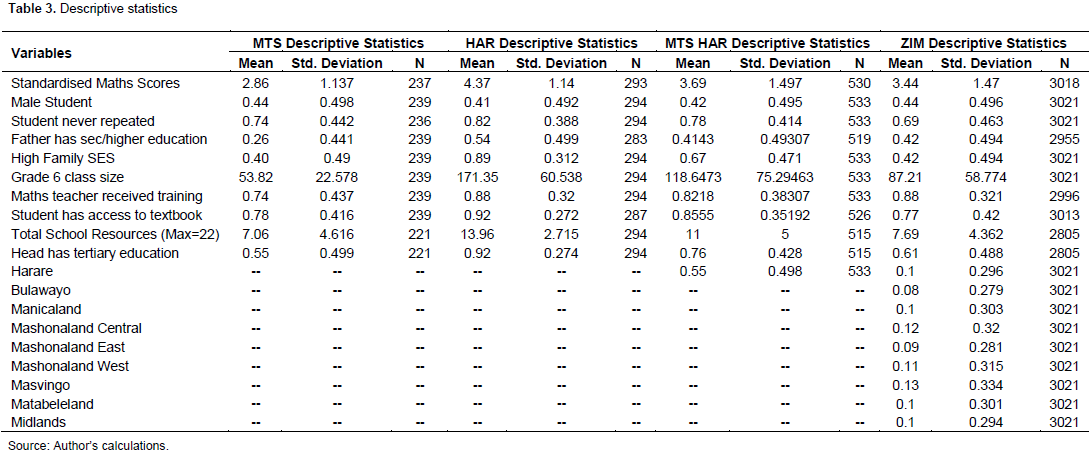
Diagnostic tests
It is important to examine that the regression analysis does not violate these assumptions. In assessing whether the residual errors are normally distributed, a Normal Probability Plot (P-P) of the Regression Standardised Residual was generated in SPSS. For normality to prevail, the points should be reasonably in a straight diagonal line from the bottom left to the top right of the plot (Boduszek, 2013). Thus, considering the plots in Figure 2, it can be concluded that in all the analyses, the random errors are normally distributed. To test for multicollinearity in HLM models generated in SPSS, one can observe the values of the tolerance and the Variance Inflation Factor (VIF). If the tolerance values are less than 0.10, and if the VIF value is above 10, one might conclude that there is multicollinearity (Boduszek, 2013). Table 4 shows the collinearity statistics for the regressions. Based on the aforementioned rule of thumb, it can be concluded that there is no multicollinearity in the model regressions. Boduszek (2013) suggests that one of the independent variables should be removed if there is multicollinearity. The variables being examined here are from the 3rd level of the HLM.
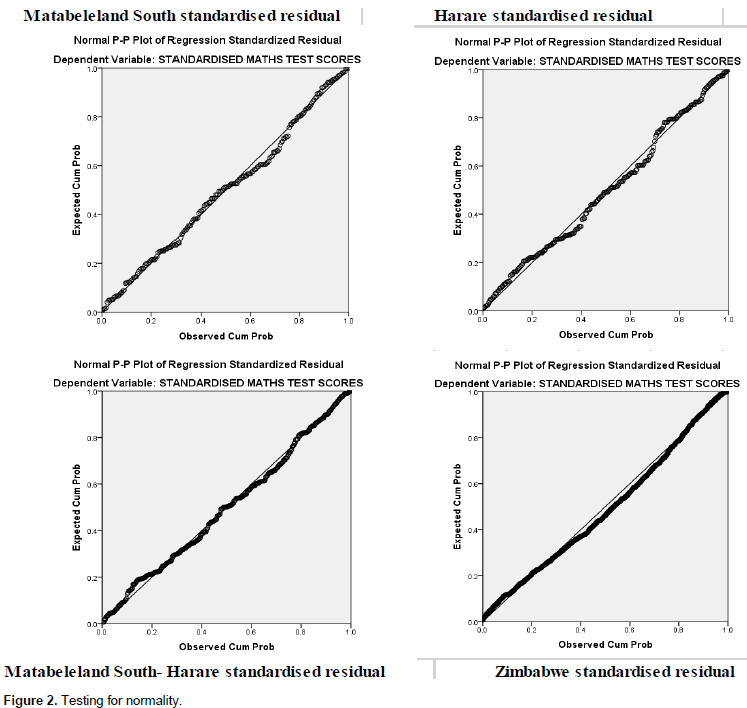
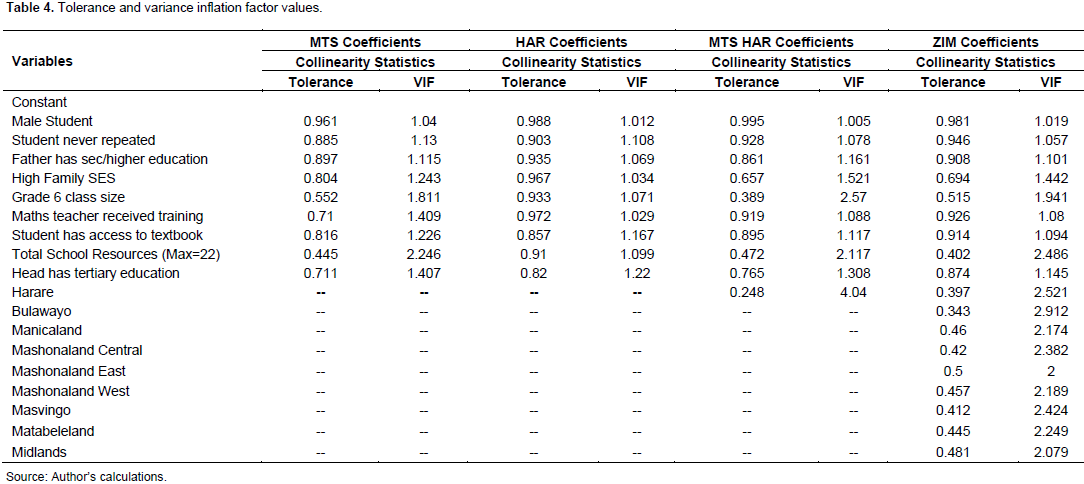
Interpreting the coefficients of the independent variables
To evaluate the effect of school resources on student achievement in a 3-level HLM method of analysis, one has to check the standardised coefficient (Beta values) generated under the significance sections of Model 3 in SPSS (Boduszek, 2013). The coefficients were generated for all 3 levels, but the important ones are those generated in Model 3 of the regression output. Table 5 depicts the extracts of the coefficients generated by SPSS for 4 regressions. The first was for Matabeleland South province (MTS), the second one for Harare province (HAR), the third for the combined regression of Matabeleland South and Harare Provinces (MTS HAR) and lastly for Zimbabwe’s 10 provinces. The regression analysis showed that in the case of MTS, a one student increase in class size decreased test scores by 0.167, contrary to the findings of the Coleman et al, (1966) and conforming to the Heyneman and Loxley (1983), though the combined regression (MTS HAR) is showing a positive effect, but not significant in the HAR regression and the 10 province regression. Furthermore, in the MTS regression, holding other variables constant, a teacher trained to teach mathematics positively impacted on a student’s achievement by as much as 0.161% points relative to untrained teachers, in line with Greenwald et al. (1996) who found teacher quality as having a significant impact on student outcomes, though not significant in the other regressions. It is worrying to find that several years after the Nyagura and Riddell (1993) study, Zimbabwe still had a high number of less qualified teachers.
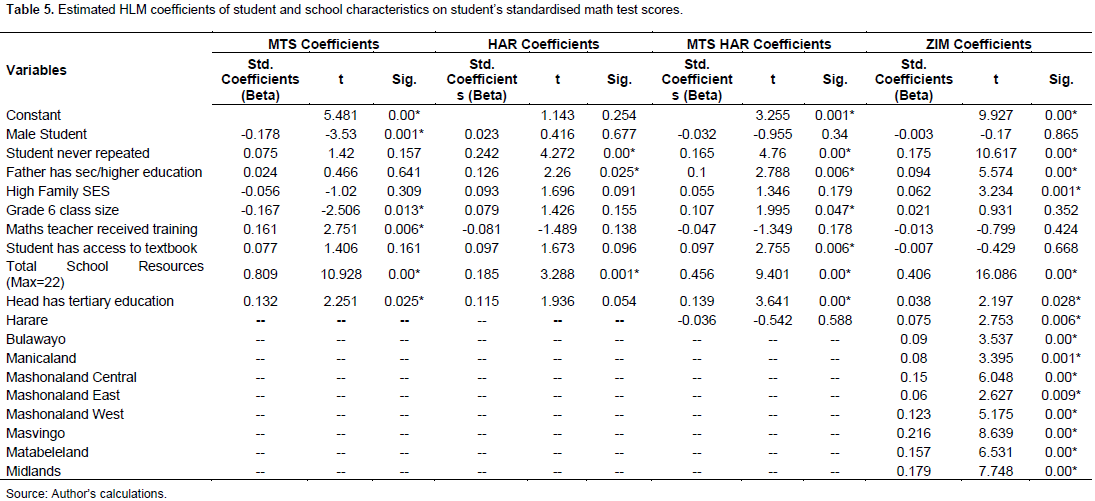
Students in schools with more facilities according to the SACMEQ III scale of 22, generally achieve higher scores than those with less facilities (beta = 0.809). The significance of school facilities is positive in all regressions, signifying the importance of such facilities irrespective of school location. These findings concur with Case and Deaton (1999) who observed that in South Africa, schools located in predominantly black communities had fewer facilities due to the Apartheid regime. The MTS data also shows that a headmaster with a tertiary qualification made students achieve atleast 0.132% points more compared to students with a school head who has no tertiary qualification. This variable is also significant in the MTS HAR and national level regressions. However, in the urban province of Harare; a head’s qualifications do not significantly influence student achievement. Holding all else equal, a male student is probably likely to score a lower maths score by 0.178% points compared to girls. The research finds a significant positive influence of having a mathematics textbook in the metropolitan province of Harare, contrary to findings in the Matabeleland South case (which finds it insignificant). Thus, a student without a maths text book falls behind with 0.097%, all things being equal.
In the MTS HRE regression, the research introduced a provincial dummy to observe whether learning in any of the provinces made a student have an edge over one who studied in a comparative province. The results show that being in any of the provinces does not explain student achievement; rather what could explain these variations was at the school level. One could suggest that what could make Harare schools perform better than Matabeleland South are not provincial level factors, but rather differences could emanate at school level. At the national level however, it shows that studying in any other province makes a student achieve better maths scores relative to being in the Matabeleland South province. Studying in the Midlands province enabled a student to achieve as much as 0.216% points (which is the highest), whereas being in Harare made a student achieve at least 0.075% points better than being in Matabeleland South. It is also important to note that this research aimed at establishing the effects of school resources, particularly school and classroom specific resources on student achievement. Considering that student level variables cannot be ignored, these have been added to this analysis as controls only. This helps address problems of bias considering that student level variables can be correlated with other regressor (Graddy and Stevens, 2003).
This research assessed how school resources have an impact on student achievement in Matabeleland South province of Zimbabwe, which has performed dismally over the years compared to other provinces. In terms of human capital resources in a school, the research reveals the importance of well trained teachers and headmasters for improved test scores. In the same vein, this will impact negatively on student achievement if teachers are made to teach large classes. There are basic facilities that are needed to enhance student achievement. Facilities such as libraries are surely important to this effect. It is therefore recommended that in order to improve student achievement in Matabeleland South, it is important that policy makers pay attention to improving human capital needs in the province (seconding well trained teachers and headmasters), class size as well as improvement of school facilities. An important contribution of this research to the student achievement discourse has been the employment of more sophisticated methodology, as recommended by Glewwe et al. (2011). Borrowing from the insights of Raudenbush and Bryk (1988), the HLM was seen appropriate considering that student achievement is impacted by various phenomena which can be grouped into 3 main levels. As earlier highlighted, if the research properly accommodates all variables, it is more likely to bring out a more accurate estimation of the effects of school resources on student achievement.
The author has not declared any conflicts of interests.
REFERENCES
Baker DP, Goesling B, Letendre GK (2002). Socioeconomic Status, School Quality, and National Economic Development: A Cross-National Analysis of the "Heyneman-Loxley Effect" on Mathematics and Science Achievement. Comparative Educ. Rev. 46:3.
Crossref
|
|
Boduszek D (2013). Multiple Regression Lecture Notes.
|
|
Bryk AS, Raudenbush SW (1988). Toward a More Appropriate Conceptualization of Research on School Effects: A Three-Level Hierarchical Linear Model. Am. J. Educ. 97(1):65-108.
Crossref
|
|
Case A, Deaton A (1999). School inputs and educational outcomes in South Africa. Q. J. Econ. 114(3):1047-1084.
Crossref
|
|
Coleman JS, Campbell EQ, Hobson CJ, McPartland J, Mood AM, Weinfeld FD York RL (1966) Equality of Educational Opportunity. Washington, DC: US Department of Health, Education & Welfare. Office of Education (OE-38001 and supp.), 1966. 548 p.
|
|
Ehrenberg RB, Brewer DJ, Gamoran A, Willms, JD (2001).Class Size and Student Achievement. Psychological Science in the Public Interest. 2:1.
Crossref
|
|
Glewwe PW, Hanushek EA, Humpage SD, Ravina R (2011). School Resources and Educational Outcomes in Developing Countries: A Review of the Literature from 1990 to 2010. NBER Working Paper No. 17554.
Crossref
|
|
Graddy K, Stevens M (2003). The impact of school inputs on student performance: An empirical study of private schools in the United Kingdom, University of Oxford, UK.
|
|
Greenwald R Hedges LV, Laine RD (1996). The Effect of School Resources on Student Achievement. Rev. Educ. Res. 66(3):361-396.
Crossref
|
|
Hanushek EA (1997). Assessing the effects of school resources on student performance: An Update. Educ. Evaluation Policy Anal. 19(2):141-164.
Crossref
|
|
Heyneman S, Loxley W (1983).The effect of primary school quality on academic achievement across twenty-nine high and low-income countries. Am. J. Sociol. 88:1162-94.
Crossref
|
|
Ker HW (2014). Application of Hierarchical Linear Models/Linear Mixed-effects Models in School Effectiveness Research. Universal Journal of Educ. Res. 2(2):173-180.
View
|
|
Makopa Z (2011).The provision of the basic classroom teaching and learning resources in Zimbabwe primary schools and their relationship with the grade 6 pupils' achievements in the SACMEQ III project.Memoir presented within the framework of the IIEP 2010/2011 Advanced Training Programme in Educational Planning and Management, UNESCO/IIEP.
|
|
Nyagura LM, Riddell A (1993). Primary School Achievement in English and Mathematics in Zimbabwe: A Multilevel Analysis. Education and Social Policy Department Human Resources Development and Operations Policy. The World Bank.
|
|
Nyamanhindi R (2014). Zimbabwe, 20 June 2014: UNICEF boosting children's nutrition to prevent stunted growth, UNICEF.
|
|
Putnam-Hornstein E (2013). Hierarchical linear modelling: Applications to social work research. J. Soc. Work. 13(6):599-615.
View
|
|
Raudenbush SW, Bryk AS (1988).Methodological Advances in Analysing the Effects of Schools and Classrooms on Student Learning. Rev. Res. Educ. 15:423-475.
View
|
|
Rumberger RW, Palardy GJ (2004). Multilevel models for school effectiveness research. In Handbook of quantitative methodology for the social sciences, edited by: Kaplan, D. 235–258. Thousand Oakes, CA: Sage.
Crossref
|
|
Southern and Eastern Africa Consortium for Monitoring Educational Quality (SACMEQ) (2014). The Southern and Eastern Africa Consortium for Monitoring Educational Quality;
View
|
|
Smith MC (2011). Which in- and out-of-school factors explain variations in learning across different socioeconomic groups? Findings from South Africa. Comparative Educ. 47(1):79-102.
Crossref
|
|
Stephens J (2007). Hierarchical Linear Models. University of Oregon.
|
|
Subedi BJ (2004). A Demonstration of the Three-Level Hierarchical Generalized Linear Model Applied to Educational Research. The Florida State University. Electronic Theses, Treatises and Dissertations. Paper 1521.
|
|
United Nation (UN) (2012). Zimbabwe 2012 Millennium Development Goals Progress Report.
View
|
|
Webster WJ, Mendro RL, Orsak TH, Weerasinghe D (1996).The Applicability of Selected Regression and Hierarchical Linear Models to the Estimation of School and Teacher Effects.Paper Presented at the American Education Research Association, (AERA). New York City.
|
|
World Bank (2014). World Development Indicators; Zimbabwe..
|